In order to extract the quantitative three-dimensional (3-D) distribution of refractive index (RI) in live cells noninvasively, optical diffraction tomography (ODT) uses the non-ionizing light sources instead of x-rays to perform a computational holographic tomography. To resolve the cellular structures with sub-wavelength resolution, the cells are sampled using tomographic scanning with oblique illumination and as many as possible scattered photons are collected with high numerical aperture (NA) objective as shown in Figs. 1(a) and 1(b). Practically, the tomographic rotation of incident beam [Fig. 1(a)] or sample [Fig. 1(b)] is always confined by a limited NA of the objective or a working distance of the imaging optics. The scattering spectrum [Figs. 1(c) and 1(d)] at certain rotation direction is, therefore, bounded at high spatial frequencies. ODT reconstructs the 3-D distribution of RI by casting scattering spectrum caps from different rotation directions together, which leads to a range of missing data in a spatial frequency domain along the rotation axis of illumination or a sample: the so-called “missing cone.” For this reason, the reconstructed 3-D images are distorted and dim, making the segmentation and quantitative analysis difficult. Solving the missing cone problem is a crucial step for widening ODT applications in cell biology research and 3-D imaging of other subwavelength structures.
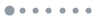
Figure 1.Schematic diagram of “missing cone” in optical diffractive tomography (ODT). (a), (b) Schematic diagram of ODT with rotating illumination (a) and rotating sample (b). (c), (d) The spatial scattering spectrum captured by the objective: red arrows indicate the transmitted and scattered signal projected on a spherical cap; the numerical aperture of the objective limits the angular bandwidth of the scattered signal. (e), (f) ODT casts spatial spectrum caps from different rotation directions together in order to reconstruct the 3-D spatial spectrum of the sample. The missing spectrum along the rotation axis is observed with both illumination rotation (e) and sample rotation (f).
To address the missing cone problem, several model-based iterative reconstruction methods were proposed by taking advantage of the sparse nature of cellular structures.1,2 In order to be consistent with measurements, these models incorporate prior information, such as, for example, non-negativity constraints on RI. However, no individual constraints can always be satisfied in real, complex cellular samples. The challenge remains to find an appropriate constraint according to the characteristics of specific cells.
In this issue of Advanced Photonics, Lim et al.3 present a deep neural network (DNN) to reconstruct the 3-D structure of red blood cells (RBCs). The progress is made in solving the missing cone problem in ODT by learning from DNN training based on the digitally constructed phantom models, which creatively provide the accurate ground truth for training the DNN. By using a digital artificial reconstruction as an initial model, the network extracts the missing features of the traditional Rytov reconstruction from the digital “ground truth” which makes a new model more accurate than previous. As a result, the network trained by these synthetic phantoms is able to successfully reconstruct an image of a mouse RBC from the experimentally measured data, resulting in image quality and resolution greatly improved over the previous methods. To further validate the result, the semisynthetic measurements generated from the reconstructed result were compared with the experimental data. Excellent consistency was obtained.
Sign up for Advanced Photonics TOC Get the latest issue of Advanced Photonics delivered right to you!Sign up now
The innovative idea of finding a specific constraint with respect to specific cell types by using the DNN as proposed by Lim et al. promotes an intriguing possibility for tackling the challenge of the missing cone problem that has plagued OTD since its inception. The image reconstruction of RBCs with high fidelity can be particularly useful for quantitative analysis of blood cells, especially in flow cytometer applications.4 DNN-based image reconstructions can also be further adapted in multi-modal imaging systems equipped with ODT,5 where correlative imaging data can be used to further support the experimental ground truth for DNN training.