1 Introduction
In the current era, the rapid advancement of optoelectronic imaging technology has highlighted the limitations of traditional imaging methods, which rely on linear models and low-order intensity information. These conventional approaches fall short in fully capturing and processing the increasing complexity of information encountered in modern imaging applications. As scenes requiring analysis become increasingly intricate—encompassing challenges such as scattering media, spectral fusion, and multi-dimensional information capture—traditional imaging systems face significant obstacles. These include increased size and weight, inaccuracies in modeling, loss of information, and limited resolution capabilities. To address these limitations, computational imaging technology (CIT)[1] has emerged as a transformative solution, offering innovative approaches to overcome the limitations of conventional imaging systems.
The integration of computing with optical imaging has given rise to the concept of “computational optical imaging,” one of the most dynamic fields within optoelectronic imaging. This interdisciplinary domain combines optical imaging, computer technology, and image processing algorithms, driving innovation at the intersection of these areas. By moving beyond the traditional “what you see is what you get” paradigm, computational optical imaging leverages front-end equipment to collect incomplete data, which is then reconstructed into complete images using computational techniques. This approach represents a significant departure from traditional optical imaging by reducing dependence on hardware design capabilities. Instead, it integrates signal processing, mathematical modeling, and informatics with other disciplines, relying on optimized algorithms and computational methods to enhance information capture and interpretation. The advent of deep learning and neural networks has further revolutionized computational imaging, particularly in handling large-scale data and modeling complex systems. The nonlinear expressive power of deep learning enables the efficient management of all aspects of the imaging process, including subsequent information extraction. This capability not only addresses the limitations of traditional physical models but also provides the automatic extraction of latent features from data, heralding a new paradigm for imaging technology.
In recent years, data-driven computational imaging technology has redefined the boundaries of optical imaging, demonstrating the unprecedented potential for a wide range of applications. Unlike traditional imaging methods that rely solely on optical hardware, computational imaging integrates light sources, media, optical systems, detectors, and processing algorithms into a cohesive and efficient imaging framework[2]. The core strengths of this technology can be categorized into three primary areas: computational optical system design, high-dimensional light-field information interpretation, and image processing and enhancement. As illustrated in Fig. 1, the optical system design in computational imaging enables the creation of compact and integrated imaging systems capable of acquiring higher-dimensional light-field information. By optimizing optical components and sensing techniques, computational imaging ensures quality and reliability while significantly reducing the size and weight of the system[3,4]. For instance, lens-free imaging, utilizing deep learning to reconstruct the light field, eliminates the reliance on intricate optical systems. Similarly, single-pixel imaging achieves image reconstruction from sparse data, offering miniaturization and lightweight designs. High-dimensional light-field interpretation extends the capabilities of imaging in terms of distance, resolution, and data dimensions[5,6]. Unlike conventional two-dimensional intensity imaging, computational imaging deciphers intricate target information, such as that obscured by haze or soot, through deep learning techniques. This advancement enables the transition from the invisible to the visible, representing a significant advancement in imaging technology. Techniques such as super-resolution imaging and stacked imaging transcend the diffraction limit by exploiting high-dimensional optical information, while holographic imaging enhances spatial dimensions, leading to significant resolution improvements. Moreover, computational imaging integrated with deep learning enables the concurrent capture of multimodal data, including spectral and polarization information. This multimodal fusion enables advanced visual tasks such as target detection, semantic segmentation, and 3D reconstruction[7,8]. Image processing and enhancement further strengthen computational imaging with advanced data handling capabilities. Deep-learning-based techniques excel in multi-source image integration, noise suppression, and detail enhancement. These methods allow efficient reconstruction even in low-signal-to-noise conditions or with incomplete data. Deep learning also predicts and supplements missing information, enabling the generation of high-quality images in challenging environments. This versatility ensures the practical effectiveness of computational imaging across a broad spectrum of real-world applications.
Sign up for Photonics Insights TOC Get the latest issue of Advanced Photonics delivered right to you!Sign up now
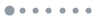
Figure 1.Data-driven computational imaging link.
This review explores the transformative potential of data-driven computational imaging technology to revolutionize the entire imaging chain, addressing the limitations of traditional imaging methods and enabling multidisciplinary applications. The analysis focuses on three core advantages: computational optical system design, high-dimensional light-field information interpretation, and advanced image processing and enhancement. In conclusion, this review highlights the current limitations of computational imaging technology, discusses prospective developments and breakthrough directions, and offers insights intended to inform and inspire researchers in related fields.
2 Development of Computational Photography
Vision is the primary means by which humans perceive and gather information about the objective world. However, the human eye is inherently limited by its visual performance, including constraints in time, space, and sensitivity. To overcome these limitations, optical imaging technology has emerged, employing systems such as microscopes and telescopes to visualize optical information while extending and enhancing the visual capabilities of the human eye. Despite these advancements, traditional optical imaging systems face significant constraints. These include limitations imposed by the intensity-based imaging mechanism, the current level of detector technology, optical system design principles, and the diffraction limit of imaging. As a result, challenges persist in achieving higher spatial, temporal, and spectral resolutions, greater information dimensions, and improved detection sensitivity. Such limitations hinder the ability of conventional systems to satisfy the growing demands for high-resolution, high-sensitivity, and multi-dimensional high-speed imaging in various applications. Moreover, in traditional optical system design, incremental improvements in imaging performance often lead to a sharp increase in the cost of hardware, making practical engineering applications difficult to achieve. Additionally, the physical limits of photodetector size, pixel size, and response sensitivity are being approached, further exacerbating the difficulty of meeting these increasingly challenging performance requirements.
With advancements in imaging electronics and enhanced computer data processing capabilities, optical field modulation, aperture coding, compressed sensing, holographic imaging, and other optoelectronic information processing technologies have seen significant progress. In addition, the natural world, through thousands of years of development, has developed diverse biological visual systems tailored to different survival needs. These biological systems provide valuable inspiration for the development of next-generation optical imaging technologies. In this context, during the mid-1990s, researchers in the optical imaging and image processing communities began exploring a novel imaging paradigm. This approach shifts away from relying solely on optical-physical devices for image formation. Instead, it integrates the joint design of front-end optics with back-end detection and signal processing, a concept termed “computational photography.” By combining optical modulation with information processing, this technology introduces innovative methods and perspectives to overcome the limitations of traditional imaging systems, as previously outlined.
At the end of the 20th century, universities such as Stanford University, Columbia University, Massachusetts Institute of Technology (MIT), and Boston University formally initiated research into computational imaging technology. They established pioneering laboratories, including the Media Lab Camera Culture Group, the Computational Imaging Lab, the Hybrid Imaging Lab, and the Electrical and Computer Engineering Lab, marking the beginning of this transformative field. Led by the United States, most top universities and research institutes quickly followed suit, creating dedicated laboratories and research centers.
Notable achievements in computational imaging research include the Media Lab at MIT, which developed single-photon sensitive airborne imaging technology. This breakthrough enables ultra-fast, real-time imaging and visual tracking of objects beyond the field of view[9]. In the field of 3D imaging, Wagner et al.[10] addressed challenges in reconstruction efficiency and quality in light-field microscopy. They proposed an artificial-intelligence-enhanced light-field microscopy framework that leverages high-resolution 2D light-sheet images as training data for convolutional neural networks. This framework achieves high-quality 3D reconstruction at video rates and can be further optimized using high-resolution light-sheet images. In coherent imaging, Babu et al.[11] developed a workflow for real-time stacked-layer imaging by integrating edge AI and deep learning. Their system employs high-performance computing (HPC) resources for online neural network training and uses a low-cost, palm-sized embedded graphics processing unit (GPU) system (edge device) at the beamline for real-time phase retrieval. This approach achieves accurate stacked-layer imaging under conditions of low spatial overlap and high frame rates, pushing the boundaries of real-time imaging capabilities.
Domestic research in computational optical imaging has progressed in parallel with international advancements. Institutions such as Xidian University, Tsinghua University, Beijing Institute of Technology, and Beijing Institute of Space Mechanics and Electricity, along with research centers like Institute of Optics and Electronics, Chinese Academy of Sciences (CAS), Xi'an Institute of Optics and Precision Mechanics of CAS, and Changchun Institute of Optics, Fine Mechanics and Physics, CAS have actively contributed to this field. These efforts have yielded internationally recognized achievements[12,13]. Beijing Institute of Technology has established dedicated laboratories to advance computational optical imaging research. The Laboratory of Computational Optical Imaging Technology at the CAS has conducted extensive studies on computational spectroscopy, optical fields, and active three-dimensional imaging[14]. The Computational Optical Remote Sensor team at the Beijing Institute of Space Mechanics and Electricity developed and launched the world’s first satellite-based computational spectroscopic imaging payload[15]. Similarly, the National Information Laboratory and the Institute of Optoelectronic Engineering at the Tsinghua University have made significant contributions to computational light-field imaging and microscopic imaging[16]. The Key Laboratory of Computational Imaging at the Xidian University focuses on scattering imaging, polarization imaging, and wide-area high-resolution computational imaging, achieving internationally recognized outcomes. The Laboratory of Optical Imaging and Computing and the Laboratory of Measurement and Imaging at the Beijing Institute of Technology have proposed optimized solutions for computational displays and computational spectral imaging[17]. Additionally, the Intelligent Computational Imaging Laboratory at the Nanjing University of Science and Technology has excelled in quantitative phase imaging, digital holographic imaging, and computational 3D imaging[18].
In recent years, the rapid growth of data volume and advancements in computing power have driven the development of artificial intelligence and deep learning technologies. These technologies have been widely applied across diverse fields, including computer vision, natural language processing, and human-computer gaming, achieving remarkable results. Simultaneously, deep learning has garnered increasing attention from researchers in optics, leading to its application in computational imaging and yielding significant achievements. Notable advances have been made in areas such as scattering imaging[19–21], ghost imaging[22–24], digital holography[25–27], super-resolution microimaging[28–30], Fourier stacked-layer micrography[31,32], and three-dimensional imaging[33–35], often surpassing the capabilities of traditional imaging methods. Compared to traditional computational imaging technologies based on physical models, deep-learning-based approaches address many longstanding challenges in the field. They offer substantial improvements in information acquisition, imaging functionality, and key performance metrics, including spatial resolution, temporal resolution, signal-to-noise ratio, and sensitivity. A diagram of this short history is shown in Fig. 1.
3 Computational Optical System Design
In contrast to conventional optical imaging, computational optical system design is based on the collaborative integration of optical measurement hardware and signal processing software. This collaborative approach enables the realization of specific imaging functions while overcoming the physical limitations inherent in traditional optical systems. It also provides the adaptability and versatility required to meet diverse mission requirements. A key research focus in computational imaging is the development of more compact and stable optical imaging systems. This includes efforts to simplify designs for lighter and smaller systems, as well as leveraging photon or optical information perception to enhance imaging capabilities, as shown in Fig. 2.
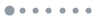
Figure 2.Applications of computational optical system design.
3.1 Compact optical system
Optical design involves optimizing the nonlinear relationship between the parameters of an optical system and its structural components. Traditional optical optimization algorithms often face challenges such as high sensitivity to damping factors and poor convergence. In contrast, deep learning offers advantages such as strong learning capability, high accuracy, and the ability to efficiently address nonlinear problems. The nonlinear problem-solving capabilities of deep learning hold significant promise for addressing various optical system design optimization problems. These include high-precision co-phase error detection, rapid and accurate wavefront reconstruction, streamlined and efficient optical design processes, and achieving low-cost yet high-quality optical imaging solutions.
3.1.1 Wavefront detection and reconstruction
In recent years, deep learning methods have been applied to wavefront detection, leveraging their powerful nonlinear fitting capabilities to establish a direct mapping relationship between aberrations and images. Unlike traditional iterative algorithms, such as phase retrieval, deep-learning-based approaches are simpler, easier to implement, and do not require iterative computations. This allows for the rapid and efficient prediction of phase information, significantly enhancing the speed and practicality of wavefront detection.
In 2014, Osborn et al.[36] introduced fully connected neural networks for open-loop wavefront reconstruction in multi-object adaptive optics (MOAO) systems. They proposed the Complex Atmospheric Reconstructor based on the Machine lEarNing (CARMEN) wavefront reconstruction method, illustrated in Fig. 3(a). While utilizing a neural network, this method adheres to the traditional process of reconstruction using wavefront slopes. In 2017, Li et al.[37] applied a backpropagation (BP) artificial neural network to sensorless adaptive optics systems, introducing a wavefront-free beam correction method based on focal plane images, as shown in Fig. 3(b). Unlike model-based methods, this approach requires only one or a few online measurements to correct wavefront aberrations, significantly enhancing real-time performance and substantially improving the Strehl ratio (SR). To address the limitations of the traditional center-of-mass calculation method in extreme conditions, Li et al.[38] proposed the SHWFS-neural network (SHNN) in 2018, illustrated in Fig. 3(c). This method reformulates center-of-mass detection as a classification problem, effectively reducing computation errors under conditions of strong background light and high noise. It improves the robustness of Shack–Hartmann wavefront sensors (SHWFSs), with results displayed in Fig. 3(d).
![Deep-learning-based wavefront reconstruction method for adaptive systems. (a) A simplified network diagram for CARMEN[36]. (b) System controller design based on BP artificial neural network[37]. (c) An architecture with 900 hidden layer neurons. (d) The original image and network output and the spot center algorithm were found[38].](/Images/icon/loading.gif)
Figure 3.Deep-learning-based wavefront reconstruction method for adaptive systems. (a) A simplified network diagram for CARMEN[36]. (b) System controller design based on BP artificial neural network[37]. (c) An architecture with 900 hidden layer neurons. (d) The original image and network output and the spot center algorithm were found[38].
In 2018, Swanson et al.[39] introduced a method for wavefront prediction that estimates the phase map of the wavefront one to five steps into the future. This predictive capability helps reduce time-delay variance in adaptive optics systems (AOS). However, the method lacks super-resolution wavefront reconstruction capability as it still relies on wavefront slopes as inputs. Building on Swanson et al.’s work, Dubose et al.[40] proposed the ISNet network, illustrated in Fig. 4(a). This method enhances the wavefront reconstruction capability of SHWFS by simultaneously utilizing both wavefront slopes and SHWFS intensity images to reconstruct aberrated wavefronts. To address the non-convergence issues in wavefront sensing optimization caused by significant wavefront errors, Paine et al.[41] developed an initial estimation method using the Inceptionv3 network architecture, shown in Fig. 4(b). This method estimates the wavefront phase using the PSF alone, providing an effective initial prediction of wavefront aberrations. It is more efficient than relying on numerous random starting guesses to handle large wavefront errors. Additionally, Ju et al.[42] proposed a feature-based phase-recovery wavefront sensing method, as depicted in Fig. 4(c). This approach directly uses image features as inputs and outputs the aberration coefficients of the optical system with good accuracy. While its precision may be slightly lower than conventional iterative phase-recovery methods, it is generally acceptable and offers practical efficiency improvements.
![Deep-learning-based wavefront phase recovery method. (a) ISNet architecture[40]. (b) Adapted Inception v3 architecture[41]. (c) Application procedure of the feature-based phase retrieval wavefront sensing approach using machine learning[42].](/Images/icon/loading.gif)
Figure 4.Deep-learning-based wavefront phase recovery method. (a) ISNet architecture[40]. (b) Adapted Inception v3 architecture[41]. (c) Application procedure of the feature-based phase retrieval wavefront sensing approach using machine learning[42].
In 2019, Hu et al.[43] introduced the SH-Net wavefront reconstruction method. This method directly utilizes SHWFS images as input, eliminating the need for image segmentation and center-of-mass localization. It enables the detection of higher-order Zernike aberrations while maintaining compatibility with existing SHWS. Subsequently, Hu et al.[44] advanced this work by achieving the prediction of up to 120 Zernike modes within 10.9 ms on a personal computer, with a model accuracy of 95.56%, depicted in Fig. 5(a). In the same year, Qi et al.[45] proposed an image-based wavefront sensing method, shown in Fig. 5(b). This approach enables deep neural networks to be trained without requiring any simulated or real extended scenes and can recover aberration coefficients from arbitrary extended scenes. Meanwhile, Nishizaki et al.[46] developed a novel wavefront sensor called DLWFS, which simplifies both optical hardware and image processing in wavefront sensing by broadening the design space. As shown in Fig. 5(c), this architecture estimates Zernike coefficients of the aberrated wavefront directly from a single-intensity image. The sensor is versatile, and capable of estimating wavefront aberrations stimulated by point sources or extended sources. Moreover, it can be trained at installation without the need for additional alignment or precision optical components, enhancing its practicality and ease of use.
![Image-based wavefront aberration estimation method. (a) Experimental optical system setup for learning based Shack–Hartmann wavefront sensor[44]. (b) Sketch map of the object-independent wavefront sensing approach using deep LSTM networks[45]. (c) Schematic and experimental diagrams of the deep learning wavefront sensor[46].](/Images/icon/loading.gif)
Figure 5.Image-based wavefront aberration estimation method. (a) Experimental optical system setup for learning based Shack–Hartmann wavefront sensor[44]. (b) Sketch map of the object-independent wavefront sensing approach using deep LSTM networks[45]. (c) Schematic and experimental diagrams of the deep learning wavefront sensor[46].
To enhance the efficiency and accuracy of wavefront-free sensing techniques, Guo et al.[47] proposed the De-VGG neural network in 2019, illustrated in Fig. 6(a). This novel phase-recovery method converts coefficient approximations into phase map computations, enabling the direct output of an optical system’s aberration phase map. Later, Wu et al.[48] introduced the phase diversity convolutional neural network (PD-CNN), shown in Fig. 6(b). This approach establishes a nonlinear mapping between intensity images and corresponding aberration coefficients. With an inference speed of approximately 0.5 ms, the method simultaneously fuses information from focal and defocused intensity images. It accurately recovers the first 15 orders of Zernike coefficients, significantly improving phase-recovery performance. Additionally, to address the limitation of wavefront recovery accuracy caused by the number of effective microlenses in SHWFS, Xu et al.[49] developed a high-precision wavefront reconstruction method using the Extreme Learning Machine (ELM) model. This method fits the nonlinear relationship between the sparse microlens center-of-mass displacements and Zernike model coefficients using a neural network. The residual wavefront RMS distributions derived from ELM are notably more stable than those calculated by traditional Zernike model methods, offering improved reliability and precision in wavefront reconstruction.
![Wavefront aberration estimation method based on phase and intensity information. (a) Working procedure of the De-VGG wavefront sensing approach[47]. (b) The architecture of the phase diversity-convolutional neural network (PD-CNN)[48].](/Images/icon/loading.gif)
Figure 6.Wavefront aberration estimation method based on phase and intensity information. (a) Working procedure of the De-VGG wavefront sensing approach[47]. (b) The architecture of the phase diversity-convolutional neural network (PD-CNN)[48].
In summary, deep learning has been applied to wavefront reconstruction in optical systems, achieving improved reconstruction performance. However, compared to traditional, well-established methods, several challenges remain to be addressed. First, the number of recoverable Zernike coefficients is currently insufficient, representing a significant limitation. Second, both accuracy and detection speed require enhancement. Complex network structures can increase computation time, negatively impacting detection speed and, consequently, the wavefront correction performance of the optical system. In addition, the growing hardware performance requirements of neural networks pose another critical challenge. To achieve high-precision wavefront reconstruction with minimal detection time, further targeted research is needed to overcome these issues in future developments.
3.1.2 Optical system optimization design
In recent years, advancements in artificial intelligence algorithms have led to the development of hybrid neural network algorithms that combine multiple approaches in the field of optical system design. For example, in 2017, Yang et al.[50] proposed a novel design framework based on a point-by-point design process to automatically create high-performance freeform surface systems. This framework requires only planar combinations as inputs, guided by configuration requirements or prior knowledge of the designer. Building on this work, the team introduced a neural-network-based method in 2019 for generating freeform off-axis three-mirror initial structures[51]. This approach reduces the difficulty of designing freeform imaging systems, making it accessible even for beginners in optical design. The design framework for automatically generating freeform initial structures is illustrated in Fig. 7.
![Design framework for automatic generation of free-form initial structures[51].](/Images/icon/loading.gif)
Figure 7.Design framework for automatic generation of free-form initial structures[51].
Finding the correct starting point is a crucial and time-intensive task in optical system design. In 2019, Hegde et al.[52] demonstrated a significant acceleration in design optimization when combined with deep neural networks (DNNs). To address this challenge, Geoffroi et al.[53] proposed a deep learning approach to identify high-quality starting points for lens design, as shown in Fig. 8(a). This method leverages machine learning to continuously expand a lens design database, enabling the generation of high-quality starting points from a variety of optical specifications. In 2021, the same team introduced a simple and modularized DNN framework[54] capable of automatically inferring initial optical system structures tailored to desired specifications. Building on this work, Geoffroi et al.[55] in 2022 proposed a neural-network-based approach to output multiple structurally distinct optical system designs for a given set of input metrics. For example, Fig. 8(b) illustrates an eight-group lens structure generated based on specified requirements and lens sequences. Simultaneously, Fan et al.[56] developed a method for automatically generating starting points for freeform three-mirror imaging systems with various folding configurations. This method automatically produces extensive datasets within a defined range of system parameters, providing well-designed starting points for immediate use. These approaches collectively significantly reduce the time and effort required for designing freeform imaging systems.
![Deep-learning-based method for automatic initial structure generation. (a) Overview of the deep learning framework used by Geoffroi et al.[53]. (b) Upon receiving a given set of specifications and lens sequence, the model outputs K = 8 different lenses that share the same sequence but differ in structures[55].](/Images/icon/loading.gif)
Figure 8.Deep-learning-based method for automatic initial structure generation. (a) Overview of the deep learning framework used by Geoffroi et al.[53]. (b) Upon receiving a given set of specifications and lens sequence, the model outputs K = 8 different lenses that share the same sequence but differ in structures[55].
In addition to time-consuming computations, the design of optical systems requires a high degree of expertise and extensive design experience, posing significant challenges. For instance, freeform lighting design often demands both specialized knowledge and lengthy calculation times. To address these issues, Gannon et al.[57] in 2018 proposed a method to enhance the efficiency and user experience of freeform lighting design. This approach simplifies the traditionally complex and computationally intensive process by training a neural network to directly map surface shapes to desired performance outcomes, bypassing intricate intermediate calculations. The result is a more streamlined and user-friendly design process. In 2021, Chen et al.[58] introduced a generalized framework, illustrated in Fig. 9(a), to generate starting points for designing freeform surface imaging optics. This framework supports a wide variety of applications, including non-rotationally symmetric freeform refraction, reflection, and retroreflection systems. It also accommodates a broad range of system parameters, offering significant flexibility in design. More recently, in 2023, Nie et al.[59] proposed and implemented a generalized differentiable freeform ray tracing module, as shown in Fig. 9(b). This module is tailored for off-axis, multi-surface freeform, or aspherical optical systems and requires minimal prior knowledge for training. After a single training session, the module can be extrapolated to various optical systems. An example of a network-generated eyepiece system created using this module is depicted in Fig. 9(c).
![Deep-learning-based optical design platform. (a) Illustration of the generalized design framework[58]. (b) Flowchart of the proposed deep learning optical design (DLOD) framework. (c) Eyepiece lens before and after training[59].](/Images/icon/loading.gif)
Figure 9.Deep-learning-based optical design platform. (a) Illustration of the generalized design framework[58]. (b) Flowchart of the proposed deep learning optical design (DLOD) framework. (c) Eyepiece lens before and after training[59].
Design optimization is a crucial but time-intensive aspect of optical system design, which can be significantly expedited with the integration of DNNs. However, several challenges remain in achieving fully automated optical system design optimization through deep learning. One key issue is that the automatically generated initial structures may fall into local optima due to insufficient metrics or conditional constraints, leading to prolonged optimization processes. Additionally, the trained network models often lack the flexibility to adapt to more complex design requirements or the incorporation of additional constraints, limiting their applicability. Addressing these challenges will be critical for future development.
3.1.3 Optical system simplification and aberration compensation
The integration of deep learning methods has significantly enhanced the imaging capabilities and aberration compensation of optical systems, effectively reducing imaging costs. In 2019, Sun et al.[60] combined single-lens design with the construction of a recovery network. This approach utilized rough surrounding depth values, rather than wavelength-scale surrounds, to wrap the optimized surface profile in diffractive lens design. This innovation enabled high-quality imaging over a large field of view using a single lens. In 2021, addressing the limitations of traditional single convex lens image recovery methods based on optimization theory, Wu et al.[61] proposed a recursive residual group network [RRG-generative adversarial network (GAN)] for generating clear images from aberration-degraded blurred images. The network architecture, depicted in Fig. 10(a), proved more effective for non-uniform deblurring tasks compared to earlier methods, with the results shown in Fig. 10(b).
![Large field-of-view computational thin plate lens imaging method. (a) The RRG-GAN network architecture. (b) RRG-GAN restoring results based on manual dataset[61].](/Images/icon/loading.gif)
Figure 10.Large field-of-view computational thin plate lens imaging method. (a) The RRG-GAN network architecture. (b) RRG-GAN restoring results based on manual dataset[61].
In 2023, Wei et al.[62] introduced a low-cost and simple optical system that effectively addressed the limitations of optical aperture and depth of field. As shown in Fig. 11(a), the system reduces the requirement for full-field-of-view aberration correction by employing an optimized phase-slice coding method within a deep learning framework. The approach utilizes a deep residual U-Net (Res-U-net)++ to simultaneously correct aberrations and coding effects across different fields of view. The imaging results, depicted in Fig. 11(b), demonstrate a remarkable 13-fold increase in depth of field, extending from 27.5 to 317.5 mm.
![U-Net (Res-U-net)++ network structure and imaging effects at different defocus distances. (a) Schematic of the network structure. The raw image is used as the input for the model, and the reconstructed image is the output of the model. (b) Image quality of the Cooke triplet lens and the doublet lens systems with the defocus amount varying within the range of −0.2 to 0.2 mm[62].](/Images/icon/loading.gif)
Figure 11.U-Net (Res-U-net)++ network structure and imaging effects at different defocus distances. (a) Schematic of the network structure. The raw image is used as the input for the model, and the reconstructed image is the output of the model. (b) Image quality of the Cooke triplet lens and the doublet lens systems with the defocus amount varying within the range of to 0.2 mm[62].
In 2019, Xu et al.[63] proposed a method for compensating wavefront aberrations using a Deep Learning Control Model (DLCM), which eliminates reliance on the deformable mirror response matrix and enhances the system’s tuning tolerance. Although the DLCM requires data collection and estimation during training, this does not affect its online operation, as an appropriately designed network structure can minimize computational overhead. Simultaneously, Nikonorov et al.[64] introduced a novel end-to-end framework, illustrated in Fig. 12(a), which employs two CNNs to reconstruct images captured with multilevel diffraction lenses (MDLs). This approach effectively addresses the inherent challenges of MDLs, such as patch-specific color blurring and smart context-aware color distortion.
![Deep-learning-based aberration compensation method. (a) End-to-end deep learning-based image reconstruction pipeline for single and dual MDLs. (b) Examples of images with reconstruction artifacts[64].](/Images/icon/loading.gif)
Figure 12.Deep-learning-based aberration compensation method. (a) End-to-end deep learning-based image reconstruction pipeline for single and dual MDLs. (b) Examples of images with reconstruction artifacts[64].
In conclusion, the continuous advancement of deep learning technology has significantly facilitated the integration of optical system design with digital algorithms, simplifying numerous imaging tasks. The robust computational capabilities of deep learning have opened new avenues for aberration compensation, enabling high-quality imaging with large fields of view, even for simple optical systems. However, these methods often involve complex computations that are disproportionate to the simplicity of the imaging systems, resulting in low image throughput and challenges in achieving real-time imaging. Additionally, most deep-learning-based approaches require the distribution of the training data to closely match that of the actual data. Ensuring this consistency, especially given the inherent limitations of computational imaging system mechanisms, remains a critical area for future research to enable reliable and practical applications.
In summary, researchers have increasingly applied deep learning to address nonlinear challenges in the automatic optimal design of optical systems. These efforts have demonstrated significant improvements in both the effectiveness and efficiency of design optimization. Notable advancements have also been achieved in wavefront detection and reconstruction, piston co-phase error estimation, optical system simplification, and aberration compensation. While deep learning faces challenges such as difficulties in dataset acquisition, high data demands during training, gradient explosion issues, and stringent hardware requirements, these limitations are progressively being mitigated through the development of network structures integrated with physical models. As the integration of deep learning and optical system design optimization deepens, deep-learning-based methods are poised to play an increasingly pivotal role in creating more convenient, cost-effective, and high-quality optical system designs.
3.2 Integrated perception imaging
3.2.1 Lens-free imaging
The essence of lens-free imaging lies in encoding the light field using methods distinct from those employed by traditional lenses, followed by recovering the desired information from the encoded light field. The chosen encoding method dictates the nature of the encoded light-field information and influences the design of subsequent decoding algorithms. Based on the modulation approach, lens-free imaging systems can be broadly classified into illumination-based modulation imaging and mask-based modulation imaging.
(1) Lens-free imaging based on illumination modulation
An illumination modulation system is designed to illuminate the imaging setup, typically using coherent or partially coherent light sources. Light waves passing through the object carry information about the object’s diffraction pattern, which is captured by a sensor. The object’s information can then be reconstructed through computational inversion of the captured data. Lens-free imaging methods based on illumination modulation commonly include shadow imaging and holographic lens-free imaging.
Shadow imaging. Shadow imaging is the simplest form of lens-free imaging, requiring only a basic hardware setup without the need for image reconstruction algorithms. However, the performance of shadow imaging systems relies heavily on uniform illumination and the presence of prominent features in particulate samples. As a result, shadow images often suffer from low resolution and indistinct target features, which significantly limits the advancement and broader application of shadow imaging technology.
To address these limitations, in 2016, Huang et al.[65] proposed a single-frame super-resolution processing network (CNNSR), shown in Fig. 13(a), to enhance low-resolution shadow images and improve the accuracy of cell counting. In 2018, Ma et al.[66] introduced the Cell Super Resolution Network (CSRNet), a reconstruction network based on the fast super-resolution CNN[67] (FSRCNN). As shown in Fig. 13(b), CSRNet was trained on a dataset comprising high-resolution and low-resolution cellular images, significantly improving image resolution according to both subjective visual assessment and objective evaluation metrics. In 2021, Zhang et al.[68] employed a super-resolution GAN (SRGAN) to overcome the resolution limitations imposed by sensor chip pixel sizes. This method effectively recovers high-resolution images of objects, as shown in Fig. 13(c). It achieves sub-micron spatial resolution across the entire effective area of the sensor chip and can enhance image resolution in under 1 s, enabling single-cell resolution across the entire effective region of the sensor chip.
![Architecture of deep-learning-based super-resolution method for shadow imaging. (a) Overall architecture of CNNSR processing[65]. (b) CSRnet structure model[66]. (c) Schematic of SRGAN-enabled contact microscopy[68].](/Images/icon/loading.gif)
Figure 13.Architecture of deep-learning-based super-resolution method for shadow imaging. (a) Overall architecture of CNNSR processing[65]. (b) CSRnet structure model[66]. (c) Schematic of SRGAN-enabled contact microscopy[68].
Another approach to addressing these challenges is enhancing the ability to extract image information, which helps mitigate issues such as blurring caused by diffraction. For instance, in 2018, Fang et al.[69] applied a CNN to leukocyte classification in a lens-free imaging system, achieving an accuracy of 90%. In 2020, Li et al.[70] introduced an unsupervised self-coding neural network fusion algorithm. This algorithm fuses multiple similar cell images, removes redundancy, and extracts common information. Compared to traditional algorithms, this method demonstrates a 7.69% improvement in information entropy (IE) and a 22.2% increase in standard deviation (SD) on average. In addition, to reduce the time and expertise required for implementation, Minyoung et al.[71] proposed a novel method for the label-free identification of CD34+ cells. By developing a customized AlexNet deep learning model, as shown in Fig. 14, this method analyzes images with greater accuracy and efficiency. The trained network achieves a classification accuracy of 97.3%, demonstrating its effectiveness in cell identification tasks.
![Architecture of the customized AlexNet deep learning model. (a) Input cell images of size 30 pixel × 30 pixel were resized to 50 pixel × 50 pixel. (b) Went through eight convolutional layers[71].](/Images/icon/loading.gif)
Figure 14.Architecture of the customized AlexNet deep learning model. (a) Input cell images of size 30 pixel × 30 pixel were resized to 50 pixel × 50 pixel. (b) Went through eight convolutional layers[71].
Since the diffraction characteristics of particles depend on their size and the signal-to-noise ratio, any background noise can adversely impact the overall performance of the system. To address this issue, in 2022, Rajkumar et al.[72] proposed a signal enhancement scheme for lens-free shadow imaging. The network structure and its enhancement effect are shown in Fig. 15. This approach improves the signal quality of captured images by more than 5 dB.
![Schematic of the signal enhancement network and enhancement effect used by Rajkumar et al. (a) Schematic of the proposed denoising and classification architecture. (b) Reconstructed results from the optimized CNN[72].](/Images/icon/loading.gif)
Figure 15.Schematic of the signal enhancement network and enhancement effect used by Rajkumar et al. (a) Schematic of the proposed denoising and classification architecture. (b) Reconstructed results from the optimized CNN[72].
Deep-learning-based methods aim to enhance the intuitive perception of feature information in shadow images, addressing issues such as diffraction noise and image blurring inherent to lens-free shadow imaging systems. While the integration of deep learning technology significantly expands the application potential of shadow imaging systems, these methods still exhibit certain limitations compared to mature traditional techniques in practical applications. Moving forward, a key development direction is to further improve the automation, performance, and practicality of shadow imaging systems while continuing to enhance their image perception capabilities. This will be essential for achieving more robust and versatile solutions in real-world scenarios.
Lens-free holographic imaging. Lens-free holography[73] is essentially a form of coaxial holography, where no microscope head is used between the object and the image sensor, and where interference between scattered light waves illuminating the object and reference light waves transmitting through the object is utilized for imaging. Since the image sensor only captures the intensity information, the phase information retrieval requires post-processing algorithms. Therefore, in the process of hologram reconstruction, there is a twin-image problem caused by the lack of phase on the reconstructed image of the direct backpropagation, which leads to a simple reconstruction for holograms that is only applicable to large and sparse objects. Therefore, in order to suppress the twin image, researchers have introduced a number of methods for phase recovery in lens-free holographic imaging techniques.
In 2017, Yair et al.[25] proposed a method for amplitude and phase recovery using the focused complex-valued field from a single holographic intensity map, as shown in Fig. 16(a). Compared to the multi-height phase-recovery algorithm, this approach significantly reduces the number of input images and shortens image processing time by a factor of three to four. The recovery results, illustrated in Fig. 16(b), demonstrate performance comparable to multi-height phase recovery, although the method is not end-to-end. In 2018, Wu et al.[74] introduced holographic imaging using deep learning for extended focus (HIDEF), a U-Net-based hologram reconstruction method. This approach reconstructs the sample’s amplitude and phase information while also performing autofocusing. However, similar to Yair et al.’s method, HIDEF requires training with sample complex-valued fields recovered from multiple phase heights as network labels. Later, Wang et al.[75] proposed a recovery method based on the Y-net network, shown in Fig. 16(c), which outputs the intensity and phase of samples separately through two-branch networks. However, its reconstruction speed was relatively slow. To address this, in 2020, Wang et al.[76] developed a Y4-type (four-channel output) network based on the Y-net architecture, as depicted in Fig. 16(d). This network efficiently and simultaneously reconstructs the amplitude and phase information of two wavelengths from a single digital hologram. The reconstruction results are shown in Fig. 16(e).
![Framework and results of the hologram information reconstruction network. (a) Network flowchart by Yair et al. (b) Red blood cell volume estimation using deep-neural-network-based phase retrieval[25]. (c) Architecture of Y-Net[75]. (d) Process of dataset generation (orange), network training (gray), and network testing (blue). (e) Reconstruction results of the example pit and pollen for single and synthetic wavelengths[76].](/Images/icon/loading.gif)
Figure 16.Framework and results of the hologram information reconstruction network. (a) Network flowchart by Yair et al. (b) Red blood cell volume estimation using deep-neural-network-based phase retrieval[25]. (c) Architecture of Y-Net[75]. (d) Process of dataset generation (orange), network training (gray), and network testing (blue). (e) Reconstruction results of the example pit and pollen for single and synthetic wavelengths[76].
In 2019, Ren et al.[77] proposed an end-to-end holographic image reconstruction method, HRnet, capable of reconstructing amplitude objects, phase objects, and more. Building on this, Zeng et al.[78] introduced another end-to-end hologram reconstruction method based on HRnet, as illustrated in Fig. 17(a). For the first time, the capsule network (CapsNet) was applied to hologram reconstruction, effectively minimizing information loss during processing. The reconstruction results, shown in Fig. 17(b), indicate that the RedCap network outperforms HRnet in terms of reconstruction quality. Furthermore, RedCap is more efficient, with only 0.71M parameters compared to HRnet’s 2.86M. However, like other network-based methods discussed earlier, these approaches have only been validated on simple objects and lack testing on more complex datasets.
![Architecture of the RedCap model and results. (a) Architecture of proposed RedCap model for holographic reconstruction. (b) Reconstructed image comparison[78].](/Images/icon/loading.gif)
Figure 17.Architecture of the RedCap model and results. (a) Architecture of proposed RedCap model for holographic reconstruction. (b) Reconstructed image comparison[78].
Denoising and super-resolution are key challenges in the development of lens-free holography. To address these issues, in 2019, Luo et al.[79] proposed an end-to-end method for generating high-resolution holograms, as shown in Fig. 18(a). This method employs a resolution decay model during training, utilizing a dataset synthesized from low-resolution holograms without requiring high-resolution holograms as ground truth. The approach not only achieves super-resolution for the specific class of samples used during training but also generalizes effectively to other types of samples.
![Deep-learning-based super-resolution holographic imaging. (a) Overview of the deep-learning-based pixel super-resolution approach[79]. (b) Schematic diagram of the CNN architecture. (c) The main components of GAN[80].](/Images/icon/loading.gif)
Figure 18.Deep-learning-based super-resolution holographic imaging. (a) Overview of the deep-learning-based pixel super-resolution approach[79]. (b) Schematic diagram of the CNN architecture. (c) The main components of GAN[80].
The inherent twin-image noise inherent in lens-free coaxial holograms is a major factor contributing to image quality degradation. Traditional model-based suppression methods require multiple lens-free images, which can be time-consuming and computationally intensive. To address this issue, Zhang et al.[80] proposed an imaging method based on GANs, as illustrated in Fig. 18(b). This method produces clear processed images, shown in Fig. 18(c), achieving image quality comparable to commercial microscope objectives. Moreover, during the image reconstruction stage, only a single lens-free image is needed, and complex operations like Fourier transforms are unnecessary, significantly reducing imaging time while maintaining high-quality results.
In 2021, Chen et al.[81] successfully reduced twin-image noise by employing a wavelet domain residual network (WavResNet), as shown in Fig. 19. Compared to direct reconstruction methods, WavResNet improves resolution by a factor of 1.6. Notably, the network demonstrates strong generalizability, performing well not only in the training region but also in different, untrained regions containing varied structures.
![WavResNet network flow[81].](/Images/icon/loading.gif)
Figure 19.WavResNet network flow[81].
Lens-free digital holograms capture the spatial information of objects but lack surface color information, resulting in a loss of color characteristics compared to digital or true-color images. To address this limitation, in 2018, Gorocs et al.[82] designed a portable and cost-effective imaging flow cytometer. This system acquires diffraction patterns of flowing micro-objects within a microfluidic channel and reconstructs them in real time using a deep-learning-based phase recovery method, generating color images of each micro-object without requiring external markers. Building on this, Riversson et al.[83] developed a digital staining technique, PhaseStain, capable of performing virtual staining of quantitative phase imaging (QPI) images. The workflow for PhaseStain is illustrated in Fig. 20(a). Conceptually, the images provided by PhaseStain serve as digital equivalents of brightfield images obtained after traditional histological staining processes, as shown in Fig. 20(b).
![PhaseStain workflow and virtual staining results. (a) PhaseStain workflow. (b) Virtual H&E staining of label-free skin tissue using the PhaseStain framework[83].](/Images/icon/loading.gif)
Figure 20.PhaseStain workflow and virtual staining results. (a) PhaseStain workflow. (b) Virtual H&E staining of label-free skin tissue using the PhaseStain framework[83].
The reliance on calibration information during lens-free holographic reconstruction limits the robustness of many algorithms for practical applications involving lens-free cameras. To address this challenge, in 2021, Rego et al.[84] proposed a neural network architecture, illustrated in Fig. 21(a), that performs joint image reconstruction and PSF estimation. This approach enables robust recovery of images captured from multiple PSFs across different cameras, achieving high reconstruction quality without requiring explicit PSF calibration. The method not only reconstructs high-quality images but also estimates the PSFs simultaneously. This innovation allows lens-free cameras to be employed in a broader range of applications requiring multiple cameras, eliminating the need to train separate models for each new camera and enhancing their practicality and versatility.
![Deep-learning-based PSF estimation method[84].](/Images/icon/loading.gif)
Figure 21.Deep-learning-based PSF estimation method[84].
The aforementioned deep-learning-based lens-free holographic reconstruction methods demonstrate the ability to rapidly reconstruct high-resolution sample images, effectively suppress twin-image noise caused by missing phase information, and even recover color information that is absent in the original data. However, most of these methods have not been validated on datasets with complex sample types. They are typically applied to simple or specific sample reconstructions, raising concerns about the stability and accuracy of these algorithms when faced with application scenarios involving diverse samples and complex contours.
(2) Lens-free imaging based on mask modulation
Mask-modulation-based lens-free imaging systems offer a versatile solution by incorporating a fixed optical mask, making them suitable for various object distances and passive or uncontrolled illumination conditions. In recent years, the rapid advancement of deep learning has driven researchers to apply techniques such as DNNs and GANs to mask-based lens-free imaging. These approaches have yielded promising results, significantly enhancing imaging quality and reconstruction speed.
Mask-based lens-free imagers traditionally rely on model-based reconstruction methods, which often involve long computational times and depend heavily on system calibration and heuristically chosen denoisers. To overcome these challenges, in 2019, Kristina et al.[85] introduced a network framework, shown in Fig. 22(a) for image reconstruction. This architecture delivers improved perceptual image quality and operates up to 20 times faster than traditional methods, enabling interactive previews of the scene. However, it requires prior training before camera operation. Building on these advancements, Wu et al.[86] in 2023 proposed MultiFlatNet, specifically designed for lens-free imaging systems, as illustrated in Fig. 22(b). They also developed the first real-time lens-free microscope, offering performance comparable to conventional lens-based microscopes. This system features the advantages of a compact design and a large field of view, with reconstruction results and processing times presented in Fig. 22(c).
![Workflow diagram of deep learning methods for computational acceleration. (a) Overview of the imaging pipeline by Kristina et al.[85]. (b) The overall architecture of MultiFlatNet. (c) Reconstruction results of real captures using various approaches[86].](/Images/icon/loading.gif)
Figure 22.Workflow diagram of deep learning methods for computational acceleration. (a) Overview of the imaging pipeline by Kristina et al.[85]. (b) The overall architecture of MultiFlatNet. (c) Reconstruction results of real captures using various approaches[86].
In addition to direct denoising, some deep learning methods leverage the unique characteristics of masks to enhance imaging quality. FlatCam has been widely utilized since its introduction in 2015 by Asif et al.[87], who designed row and column separable masks based on maximum length sequences and developed the FlatCam system. However, its iterative-optimization-based reconstruction algorithm often results in noisy images with poor perceptual quality. To address this issue, in 2020, Salman et al.[88] proposed FlatNet, a deep-learning-based approach for recovering coded images from FlatCam. FlatNet significantly improves the quality of lens-free reconstructed images, achieving enhancements by several orders of magnitude. However, training FlatNet requires tens of thousands of image datasets, representing a considerable workload. This limitation highlights the need for more efficient training methods or alternative approaches to reduce data collection requirements.
![Deep neural network based on mask properties. (a) Overview of imaging pipeline by Zhou et al.[89]. (b) Diagram of end-to-end DPDAN.](/Images/icon/loading.gif)
Figure 23.Deep neural network based on mask properties. (a) Overview of imaging pipeline by Zhou et al.[89]. (b) Diagram of end-to-end DPDAN.
Later, Zhou et al.[89] modeled the optimization process of traditional iterative methods as a deep parsing network and proposed a deep parsing network (DPDAN) based on deep denoising prior, as illustrated in Fig. 23(c). This approach was demonstrated to be more efficient than gradient descent within the unfolding framework. DPDAN is a generalized non-blind recovery method, providing a solution to the broader class of inverse problems. The reconstruction results achieved by this method outperform previous approaches in both visual perception and quantitative evaluation.
In addition to FlatCam, the Fresnel zone aperture (FZA), a widely used mask in lens-free imaging, was introduced by Tajima et al.[90,91]. This technique enables lens-free coded aperture imaging in the visible light spectrum by employing Fresnel waveband slices with varying phases. However, this method requires multiple images of waveband slices with different phases for reconstruction, which limits its efficiency. To address this limitation, in 2020, Wu et al.[3] proposed a single-frame reconstruction algorithm for lens-free imaging. Since FZA images resemble holograms of point sources, the imaging model can be explained using theories from coaxial holography. However, iterative reconstruction methods based on geometric optical models tend to produce artifacts and are computationally intensive. To overcome these challenges, Wu et al.[92] in 2021 developed an end-to-end deep neural network called DNN-FZA (deep neural network-Fresnel zone aperture), as shown in Fig. 24. This network addresses the Fresnel inverse problem for waveband-slice-encoded aperture imaging. While DNN-FZA significantly enhances reconstruction efficiency, it relies on simulated PSFs to generate large datasets for training. Consequently, when recovering real data, the network—trained exclusively on simulated data—produces some errors.
![Schematic of the network framework of DNN-FZA. (a) Image acquisition pipeline and reconstruction for the DNN-FZA camera. (b) Architecture of the U-Net. (c) Up- and down-projection units in DBPN[92].](/Images/icon/loading.gif)
Figure 24.Schematic of the network framework of DNN-FZA. (a) Image acquisition pipeline and reconstruction for the DNN-FZA camera. (b) Architecture of the U-Net. (c) Up- and down-projection units in DBPN[92].
Existing reconstruction algorithms typically assume that the forward imaging process is a convolutional operation with a PSF based on a system model. However, in practice, many of these methods suffer from model mismatch, resulting in poor image reconstruction quality. To address this issue, Zeng et al.[93] proposed a physical information deep learning architecture (MMCN), as illustrated in Fig. 25, designed to mitigate mismatch errors. This architecture integrates physical imaging principles with deep learning, enhancing both the effectiveness and robustness of the reconstruction process for real lens-free images. Experimental results demonstrate that MMCN significantly outperforms other reconstruction methods.
![MMCN network configuration framework[93].](/Images/icon/loading.gif)
Figure 25.MMCN network configuration framework[93].
The multiplexed nature of lens-free optics makes global features essential for accurately interpreting optical coding patterns. However, commonly used fully convolutional networks (FCNs) are not efficient at inferring global features. To address this limitation, Pan et al.[94] proposed a fully connected neural network framework with a Transformer, as illustrated in Fig. 26(a). This architecture excels in global feature inference, significantly improving reconstruction results. Its superiority is demonstrated through comparisons with model-based and FCN-based approaches in optical experiments, as shown in Fig. 26(b).
![Schematic of the network of the method proposed by Pan et al. (a) Lens-free imaging transformer proposed by Pan et al. (b) Results of image-on-screen experiment and object-in-wild experiment[94].](/Images/icon/loading.gif)
Figure 26.Schematic of the network of the method proposed by Pan et al. (a) Lens-free imaging transformer proposed by Pan et al. (b) Results of image-on-screen experiment and object-in-wild experiment[94].
Although deep learning algorithms have significantly advanced the development of mask-based lens-free imaging, they still face several challenges that require further research. The first challenge is dataset acquisition. While simulated data generation[92] is fast and convenient, it often deviates from real-world scenarios. Conversely, acquiring data through actual imaging[93] is more accurate but is time-consuming and labor-intensive. Second, the complexity of network structures imposes higher hardware requirements on the imaging system. Additionally, running these algorithms introduces issues such as increased power consumption, heat generation, and processing delays, which contradict the inherent goals of convenience and low cost that define lens-free imaging.
In summary, while the elimination of lenses in lens-free imaging systems offers advantages such as low cost, portability, and a simplified structure, it also introduces challenges, including significant noise, poor image quality, and low resolution. The performance of the reconstruction algorithm plays a crucial role in determining the final image quality of the system and significantly influences the development potential of lens-free imaging technology. Future research should focus on several key areas: improving the adaptability of reconstruction methods to real-world application scenarios and strengthening their relevance to subsequent tasks, designing networks with stronger generalization capabilities and more efficient structures, and reducing the training costs associated with these networks. Addressing these challenges will be essential for advancing lens-free imaging technology.
3.2.2 Correlated imaging
Correlated imaging is a non-deterministic imaging modality distinct from conventional optical imaging techniques. The concept was first proposed by Klyshko[95] in 1988 and experimentally validated by Pittman et al.[96] in 1995. This method reconstructs images of unknown objects by analyzing the correlations in the rise and fall of light intensity. Compared to traditional optical imaging, correlated imaging offers advantages such as high sensitivity, strong anti-interference capabilities, and compatibility with a wide range of wavelengths. These characteristics enable it to significantly enhance imaging quality in complex environments. This unique imaging approach, often referred to as ghost imaging, holds great promise in applications such as super-resolution imaging[97], biomedicine[98], information encryption[99], and LiDAR[100].
(1) Ghost imaging
Traditional ghost imaging techniques require a large number of random samples to suppress noise fluctuations and achieve high-contrast images. This results in high data demands and a time-consuming data acquisition process. Combining computational ghost imaging with deep learning has emerged as a solution to these challenges, enabling real-time image recovery at low sampling rates. In 2017, Lyu et al.[22] introduced the first ghost imaging technique based on deep learning, capable of reconstructing high-quality binary images at low sampling rates. This approach used thousands of ghost imaging patterns as inputs and the image of the target object as the output to train DNNs. Recent advancements in deep-learning-based ghost imaging have focused on several key areas: increasing imaging speed for real-time, high-quality imaging; achieving reliable imaging performance in extreme environments; and producing high-quality images at significantly lower sampling rates.
To improve the imaging rate, Mizutani et al.[101] in 2021 analyzed the causes of image degradation in ghost imaging and applied a CNN to enhance the imaging rate for low-light detection. Experimental results demonstrated that this method simultaneously improved both the imaging rate and the quality of ghost imaging. The experimental system, network structure, and corresponding results are shown in Fig. 27. In 2022, Zhou et al.[102] proposed a real-time computational reimaging method based on deep learning and array spatial light-field modulation. This approach achieves real-time, high-quality imaging of continuously moving objects by adjusting the array light field to enable physically compressed imaging. Deep learning is then used for multiplexing and downsampling multiplication.
![GI incorporating CNN. (a) Experimental setup of GI for detecting scattered light. (b) Diagram of CNN architecture for GI. (c) Experimental results of GI image without CNN. (d) Experimental results of GI image reconstructed using CNN[102].](/Images/icon/loading.gif)
Figure 27.GI incorporating CNN. (a) Experimental setup of GI for detecting scattered light. (b) Diagram of CNN architecture for GI. (c) Experimental results of GI image without CNN. (d) Experimental results of GI image reconstructed using CNN[102].
In extreme environments, where the signal from the target object is very weak, obtaining high-quality imaging can be challenging. To address this issue, Li et al.[103] developed a polarimetric ghost imaging (PGI) system in combination with deep learning. This system suppresses the backward scattering of volumetric media, achieving high-quality reconstruction at a sampling rate as low as 1.6% under high scattering conditions. The imaging results showed significant improvement compared to traditional methods. The experimental system, network structure, and corresponding results are illustrated in Fig. 28. In 2022, Zhang et al.[104] proposed a CGAN trained on simulated data and integrated with computational ghost imaging for weak signal detection.
![Network architecture of the PGI system and result diagrams[102]. (a) CNN architecture in the PGI system. (b) Comparison of the DL reconstruction and CS reconstruction results for different sampling rates and turbidity scenarios.](/Images/icon/loading.gif)
Figure 28.Network architecture of the PGI system and result diagrams[102]. (a) CNN architecture in the PGI system. (b) Comparison of the DL reconstruction and CS reconstruction results for different sampling rates and turbidity scenarios.
For imaging at low sampling rates, Wu et al.[105] developed an end-to-end DNN (DDANet) for computational ghost imaging reconstruction, enabling clear imaging at sub-Nyquist sampling rates. Similarly, Song et al.[106] proposed a computational ghost imaging method combining deep learning with peak noise scattering patterns. This method enhances the quality of reconstructed images by training the network solely on simulated data. Experimental results demonstrate that it achieves high-quality imaging at sampling rates as low as 0.8% and is robust in noisy environments. The network structure, training process, and experimental results are presented in Fig. 29. While these methods enable image recovery at low sampling rates, achieving high-quality imaging for complex targets remains challenging. To address this, Zhu et al.[107] introduced multiscale randomized patterns in computational ghost imaging to capture detailed contour information of the target. They employed a multi-input network to adapt to low sampling rate conditions. Experimental results showed that this approach accurately recovers target images even at very low sampling rates, significantly advancing the capabilities of computational ghost imaging.
![(a) Diagram of DNN network architecture. (b) Training process of CGIDL. (c) Plot of experimental results of different methods[106].](/Images/icon/loading.gif)
Figure 29.(a) Diagram of DNN network architecture. (b) Training process of CGIDL. (c) Plot of experimental results of different methods[106].
In summary, as a representative computational imaging technology, ghost imaging offers highly flexible imaging modes. When combined with the powerful learning capabilities of deep learning, it significantly enhances the information acquisition, imaging speed, quality, and functionality of ghost imaging systems. This integration provides a robust solution for addressing increasingly complex imaging scenarios and mitigating the impact of various disturbances, paving the way for broader and more advanced applications.
(2) Single-pixel imaging
Single-pixel imaging is an emerging imaging technology that captures target image information using a single-pixel detector without spatial resolution. Compared to traditional optical imaging methods based on object-image conjugation, single-pixel imaging offers advantages such as strong anti-interference capability, high sensitivity, and high resolution. As a result, it is widely applied in fields such as remote sensing imaging[108], microscopic imaging[109], and three-dimensional imaging[4]. In single-pixel imaging, the image reconstruction algorithm plays a crucial role in determining the quality and efficiency of image reconstruction, making it a key aspect of the technology. Based on the image reconstruction algorithm used, single-pixel imaging can be categorized into correlation single-pixel imaging, compressed sensing single-pixel imaging, and Fourier single-pixel imaging (FSPI). Each method has its own focus: the association algorithm aims to enhance imaging quality and reduce imaging time; the compression perception algorithm aligns with the theoretical framework of single-pixel imaging and significantly improves reconstruction quality; and Fourier single-pixel imaging introduces frequency-domain information, leveraging spectral data to reconstruct the target information more effectively.
In recent years, researchers have integrated deep learning with single-pixel imaging technology, offering new possibilities while addressing challenges such as low image reconstruction quality and the difficulty of achieving real-time, high-quality imaging under undersampling conditions associated with traditional reconstruction algorithms. Currently, the focus is on leveraging neural networks to reduce the number of required samples, enhance reconstruction quality, and shorten imaging times. This enables faster and more accurate image restoration, presenting new opportunities for advancing single-pixel imaging technology.
Single-pixel imaging enables high-speed imaging and imaging across a wide wavelength range; however, its image quality often degrades under undersampling conditions. Integrating deep learning can effectively address this limitation. Hoshi et al.[110] combined a recurrent neural network (RNN) with single-pixel imaging technology, reducing the number of internal parameters in the imaging process and reconstructing higher-quality images with improved noise robustness. The network structure and reconstruction results are shown in Fig. 30. While this method effectively reduces noise for sparse objects, its performance on complex objects remains relatively poor. To address the issue of prediction uncertainty in deep learning applications, Shao et al.[111] proposed a single-pixel imaging method based on a Bayesian CNN (BCNN). This approach predicts each pixel as a probability distribution rather than a fixed intensity value, and it approximates uncertainty by minimizing training errors. Experimental results demonstrate that this method effectively quantifies the uncertainty of deep learning predictions.
![(a) Structure of recurrent neural network combining convolutional layers. (b) Comparison of results[110].](/Images/icon/loading.gif)
Figure 30.(a) Structure of recurrent neural network combining convolutional layers. (b) Comparison of results[110].
The aforementioned methods primarily leverage prior knowledge from data to derive the optimal solution for the target object image. During reconstruction, these methods require only an approximate image or a single-pixel detection signal as input into the mapping relationship, eliminating the need for iterative operations and enabling rapid results. To address the issue of poor generalization associated with these approaches, Wang et al.[112] proposed a physically enhanced deep learning method for single-pixel imaging. This method integrates a physical information layer with a model-driven fine-tuning process to complete the image reconstruction. Experimental results demonstrate that this approach surpasses several widely used algorithms in terms of robustness and fidelity. The network structure and corresponding experimental results are shown in Fig. 31.
![(a) Structure of DNN network. (b) Comparison of the original image and the effects of different methods[112].](/Images/icon/loading.gif)
Figure 31.(a) Structure of DNN network. (b) Comparison of the original image and the effects of different methods[112].
The FSPI technique reconstructs high-quality images by acquiring the Fourier spectrum of the target image. This technique has opened new avenues for single-pixel imaging technology. However, it requires collecting a large amount of data to capture sufficient spatial information, which increases imaging time and degrades real-time imaging quality. To address these challenges, many researchers have incorporated deep learning into FSPI, achieving promising results. These approaches aim to enhance imaging efficiency and quality, particularly in real-time applications, by leveraging the powerful learning and inference capabilities of neural networks.
To address the issue of low real-time imaging quality in FSPI, Rizvi et al.[113] employed a deep convolutional autoencoder network (DCAN) with a symmetric jump-connectivity architecture to reconstruct images. Experimental results demonstrated that this method achieves real-time imaging at low sampling rates and surpasses traditional FSPI techniques in terms of imaging quality. The system schematic, network architecture, and corresponding experimental results are illustrated in Fig. 32. Yang et al.[114] proposed an FSPI method based on a GAN. This method integrates perceptual loss, pixel loss, and frequency loss into the total loss function, enabling it to better preserve the detailed information of the target. Experimental results showed that this approach produces high-quality reconstructions at low sampling rates, with superior visual effects and improved image quality evaluation metrics.
![Network architecture of the proposed DL-FSPI system and experimental results[113]. (a) DCAN network architecture used. (b) Experimental results of the DL-FSPI system.](/Images/icon/loading.gif)
Figure 32.Network architecture of the proposed DL-FSPI system and experimental results[113]. (a) DCAN network architecture used. (b) Experimental results of the DL-FSPI system.
FSPI operates in the frequency domain, and its combination with deep learning effectively addresses the challenge of prolonged reconstruction times, enabling real-time imaging. However, the method requires substantial time and computational resources for network training, highlighting the need for improvements to reduce these costs. Currently, deep-learning-based single-pixel imaging demonstrates significant advantages, including higher-quality reconstructions, faster real-time imaging, and adaptability to complex scenarios such as scattering media, space remote sensing, and microscopic observation. Nonetheless, challenges remain, such as the need to readjust and retrain network structures when factors like the number of samples, modulated light fields, or imaging sizes change. Developing flexible and adaptive deep-learning-based single-pixel imaging methods is therefore crucial to overcoming these limitations and advancing the practical applications of this technology.
3.2.3 Photon-counting imaging
Photon-counting imaging is an ultra-sensitive detection technique that typically employs avalanche photodiode arrays operating in Geiger mode to detect weak light signals. It is characterized by its high sensitivity, fast response speed, and low leakage and false detection rates, making it indispensable in applications such as LiDAR, radiation detection, and spectral measurement. Single-photon-counting imaging is particularly effective under extremely low-illumination conditions, where it is used to reliably detect and reconstruct target images.
Single-photon-counting imaging, which detects and counts individual photons from reflected light, is a versatile technique for target detection in extremely low-light conditions. However, low photon counts and noise can significantly degrade imaging quality in such environments. To address these challenges, researchers have proposed integrating deep learning into single-photon-counting imaging.
To address the problem of noise in single-photon-counting imaging, Tian et al.[115] proposed a Poisson-optimization-inspired neural network for denoising single-photon-counting images. This method begins by establishing a learnable prior, which is used to regularize the Poisson optimization problem. It then unfolds an iterative shrinkage-thresholding algorithm to solve the optimization problem effectively. Experimental results demonstrate that this approach significantly enhances both the visual quality and quantitative accuracy of the reconstructed images. The network architecture, experimental setup, and corresponding results are shown in Fig. 33.
![(a) Architecture of SPCI-Net layer[115]. (b) Diagram of the experimental setup. (c) Visualization of different methods under high-SNR conditions. (d) Visualization of different methods under low-SNR conditions.](/Images/icon/loading.gif)
Figure 33.(a) Architecture of SPCI-Net layer[115]. (b) Diagram of the experimental setup. (c) Visualization of different methods under high-SNR conditions. (d) Visualization of different methods under low-SNR conditions.
In addition to the methods discussed above, some researchers have applied compressed sensing (CS) theory to single-photon-counting imaging to address the problem of imaging noise. To overcome the time-consuming nature of traditional CS reconstruction algorithms, Guan et al.[116] proposed a single-photon-counting compressed imaging system based on a sampling and reconstruction-integrated deep network (BF2C-Net). This method incorporates a binarized fully connected layer as the first layer of the network, which is trained as a binary measurement matrix to perform efficient compressive sampling. The subsequent layers are dedicated to fast image reconstruction. Similarly, Wen et al.[117] introduced a sampling and reconstruction-integrated neural network for single-photon-counting compressed imaging. This approach simulates compressed sampling by jointly training a sub-pixel convolution with a deep reconstruction network. By modifying the forward and backward propagation of the network, they train the first layer as a binary matrix, which is applied to the imaging system to improve imaging speed. Additionally, Gao et al.[118] proposed a compressed reconstruction network (OGTM) based on a generative model. This method improves reconstructed images by increasing the sampling mesh and achieving joint optimization of sampling and image generation. The network structure and experimental results are shown in Fig. 34.
![Comparison of OGTM network architecture and experimental results[118]. (a) OGTM network architecture and migration learning network. (b) Comparison of OGTM experimental results.](/Images/icon/loading.gif)
Figure 34.Comparison of OGTM network architecture and experimental results[118]. (a) OGTM network architecture and migration learning network. (b) Comparison of OGTM experimental results.
In summary, in single-photon-counting imaging, the primary source of noise is Poisson noise, and the integration of neural networks can effectively eliminate this noise, thereby improving imaging quality. This combination enhances the visualization performance of photon-counting images, both in terms of visual appeal and evaluation metrics. By leveraging the advantages of deep learning, it is possible to significantly improve the quality of reconstructed images in low-light environments. This provides a solid theoretical and experimental foundation for future advancements in the classification and denoising of noise. The approach holds promising potential for applications in low-light imaging fields such as fluorescent molecule detection, X-ray imaging, and deep-space probe imaging.
4 Interpretation of High-Dimensional Information in Light Field
In the field of computational and optical imaging, the extraction and decoding of high-dimensional optical field information have been central to driving both technical and theoretical advancements. This section focuses on three core dimensions: imaging distance, imaging resolution, and information acquisition capability, as shown in Fig. 35. The limitations of distance and penetration through complex media have been addressed through techniques such as scattering imaging, non-line-of-sight imaging, polarization descattering, and laser selectivity. These methods have made it possible to observe details that were previously unobservable. Concurrently, techniques such as stacked imaging, super-resolution imaging, and holographic imaging have improved clarity at the microscopic level, enabling the precise rendering of minute structures. Additionally, polarization and spectral imaging techniques go beyond the capabilities of traditional imaging methods, allowing for the acquisition of multi-dimensional data and providing richer background information about scenes and targets. At the heart of these advancements is the interpretation of higher-order light-field information. The integration and advancement of these technologies offer a more nuanced and multifaceted understanding of the intricacies of the world, redefining how both visible and invisible environments can be explored.
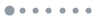
Figure 35.Applications of light-field high-dimensional information acquisition.
4.1 Imaging distance extension
4.1.1 Imaging through scattering media
Imaging through scattering media has been a significant challenge in complex environments, such as rain, snow, haze, and biological tissues, because the wavefront of incident light is distorted due to the interference caused by the inhomogeneous distribution of scattering media. In recent years, substantial progress has been made in scattering media imaging, with methods such as measurement matrices, speckle correlation imaging, and the shower curtain effect. These approaches leverage the property that target information does not entirely disappear after passing through scattering media. However, these methods are limited to reconstructing sparse objects and struggle with non-sparse samples, such as biological specimens. With the advancement of artificial intelligence, powerful deep learning methods have been applied to optical imaging to address the scattering problem[119–121]. Lyu et al.[122] were among the first to use a trained DNN for the acquisition of hidden targets. However, a large number of fully connected architectures in the DNN model can lead to the risk of overfitting[1]. To mitigate this, Li et al.[19] proposed a CNN-based method for imaging through ground glass. In this model, each neuron is connected only to a few nearby neurons from the previous layer, reducing the likelihood of overfitting. These studies demonstrate that deep learning can reveal the characteristics of complex relationships by transforming lower-level representations into higher, more abstract levels[123]. This capability is widely used to solve inverse problems in scattering imaging. By combining deep learning with classical scattering media inverse problems, such as wavefront focusing, speckle correlation, and deconvolution, the application scope of these methods has been significantly expanded.
(1) Wavefront refocusing
Wavefront refocusing enables the focusing of light in or through scattering media by optimizing the incident wavefront and replanning the light transmission path within the medium. Deep learning’s ability to model complex relationships has been leveraged to focus light[124–126] and to reconstruct images through static scattering media[9,11,19,20,127,128]. In 2018, Turpin et al.[124] introduced neural networks for binary amplitude modulation, using them to focus light through a single diffuser. Similarly, Li et al.[20] employed speckle patterns generated by four diffusers on various objects for U-Net training. The pre-trained network demonstrated the ability to generalize to “invisible” objects or diffusers. However, a limitation of these approaches is that all the diffusers used shared the same macroscopic parameters.
Luo[129] introduced a deep-learning-based adaptive framework to tackle the challenge of optical focusing and refocusing through nonstationary scattering media using wavefront shaping. This approach circumvents the reliance on classification or pre-trained models. The core of the framework is recursive fine-tuning, which maximizes the correlation between the media states before and after the change, commonly referred to as speckle correlation[130–132]. Therefore, only a small number of new samples are required to fine-tune the previous network, leading to rapid recovery of the focusing performance and enabling real-time imaging through the transmitted scattering medium.
However, the “one-to-one” mapping approach is highly susceptible to speckle decorrelation—small perturbations in the scattering medium can lead to significant degradation in model accuracy and imaging performance. To address this issue, Li et al.[20] proposed a statistical “one-to-all” deep learning (DL) technique that can adapt to speckle decorrelation. Specifically, Li et al. developed a CNN capable of learning the statistical information contained in speckle intensity patterns captured across a set of diffusers with the same macroscopic parameters. This approach demonstrated, for the first time, that a trained CNN can generalize and predict high-quality object images through a set of completely different diffusers from the same class. The training process and results are shown in Fig. 36.
![(a) Experimental setup. (b) CNN architecture. (c) Testing results of “seen objects through unseen diffusers[20].](/Images/icon/loading.gif)
Figure 36.(a) Experimental setup. (b) CNN architecture. (c) Testing results of “seen objects through unseen diffusers[20].
To enhance the speed and accuracy of wavefront reconstruction, researchers have proposed using DL methods to directly obtain aberrations from far-field images. Trained neural networks can quickly and accurately reconstruct the wavefront. In 2018, Jin et al.[133] employed a CNN to establish a nonlinear mapping from PSF images with single-frame distortion to Zernike polynomial coefficients. The experimental results showed that the aberration correction accuracy reached approximately 90%, with an average mean square error of 0.06 for each Zernike coefficient across 200 replicates. In 2020, Jin et al.[134] constructed a dual-stream CNN framework with shared weights to predict Zernike coefficients, addressing the domain drift issue caused by differences between the training set and target test set. This method improved accuracy by 18.5% compared to the traditional CNN-based approach, with almost the same training and processing times. In 2019, Tian et al.[135] proposed an aberration correction method based on DNNs that directly infers wavefront distortion from the intensity image captured by a charge-coupled device (CCD). This method helps resolve delays caused by the numerous iterations required by traditional optimization algorithms in adaptive optics for wavefront-free sensors. Simulation results demonstrated that the proposed method significantly reduced computation time and improved real-time performance. In 2023, Siddik et al.[136] developed a method to infer corrected Zernike coefficients in the pupil of the imaging system from a single turbulence-degraded intensity image. The performance of the neural network was tested and compared under four conditions: noise-free point light source, noise-free extended light source target, low-noise extended light source, and high-noise extended light source. The results indicated that for a noise-free point light source target, the neural network achieved the smallest mean square error and the best wavefront reconstruction performance. However, the network’s performance declined as turbulence intensity increased, with a corresponding rise in mean square error.
The methods discussed above overcome the inherent limitations of traditional imaging techniques and significantly enhance the scalability of scattering imaging. Traditional methods are limited by the “one-to-one” limitation, meaning that a model is only effective for a specific scattering medium. In contrast, DL employs a “one-to-all” strategy, where a single model can be applied to all scattering media within the same class. This flexibility enables DL to improve both the speed and accuracy of scattering imaging, making it highly valuable in practical applications.
(2) Speckle correlation imaging
Speckle correlation imaging is widely used as a noninvasive method for scattering imaging, but its application is limited by the influence of the optical memory effect (OME). DL, which optimizes the parameters within the neural network through data training to simulate the imaging process, has shown significant potential in addressing the inverse problem of scattering imaging. In 2015, Ando et al.[137] first used support vector machines (SVMs) to identify and classify the speckle patterns formed by target objects hidden behind scattering media on the image surface. In 2016, Horisaki et al.[138,139] applied support vector regression (SVR) to simulate the scattering imaging process by learning the relationship between a large number of target objects and their corresponding speckle patterns. This approach enabled refocusing and imaging through ground glass. In 2017, Lyu et al.[122] achieved imaging of target objects through scattering media for the first time using DL. Building on this, in 2018, Li et al.[19] used a CNN to enable scattering imaging through ground glass and explored factors affecting the quality of image reconstruction, including the dataset and loss function used in neural network training, as well as the characteristics of the scattering medium.
However, in practice, the scale of the measured target and the nature of the scattering medium are highly complex. The interaction between light and particles disrupts the traditional imaging mechanism, and the detector typically records a disordered speckle pattern. Deep-learning-based scattering imaging, which uses an “end-to-end” training strategy, directly employs the target image and its corresponding speckle pattern as training data to train the neural network. While effective, this approach causes the trained network to rely heavily on the fixed imaging environment, significantly limiting its generalization ability across different scattering media or object types. As a result, this limits the applicability of DL in real-world scattering imaging scenarios. To overcome this limitation, the reconstruction algorithm must exhibit strong generalization ability to adapt to tasks involving different diffusers and varying scale targets. Addressing the obstacle posed by the OME and ensuring that the system has both robust reconstruction capability and adaptability for complex targets are essential conditions for the practical application of scattering media imaging.
Guo et al.[140] developed the PDSNet network (Pragmatic De-scatter ConvNet) to eliminate the obstacle of the OME that limits the field of view, utilizing a data-driven approach. They combined the traditional principles of speckle correlation imaging to guide the design and optimization of the network, enabling it to handle random scale and complex targets. Experimental results demonstrated that PDSNet achieved at least a 40-fold expansion of the OME range, with the average peak signal-to-noise ratio (PSNR) exceeding 24 dB. Under conditions with untrained scales, the average PSNR of the reconstructed image was greater than 22 dB, successfully reconstructing complex targets such as faces. The implementation process and experimental results are shown in Fig. 37.
![(a) Experimental setup uses a DMD as the object. (b) Test results of the complex object dataset with two characters, three characters, and four characters[140].](/Images/icon/loading.gif)
Figure 37.(a) Experimental setup uses a DMD as the object. (b) Test results of the complex object dataset with two characters, three characters, and four characters[140].
Although CNNs perform well in image processing tasks, they are considered “local” models. The limited size of the convolution kernel reduces CNNs’ ability to process non-local information, which weakens their capability to extract speckle pattern features and reconstruct images, especially in scattering imaging. To address this limitation, recent advancements have incorporated the Transformer into scattering imaging to enhance the network’s ability to interact with global information. In 2023, Wang et al.[141] proposed a “non-local” lightweight network model, Speckle-Transformer (SpT) U-Net, designed to extract speckle pattern features from face data under different scattering mechanisms and reconstruct face images. The network combines the U-Net and Transformer codec structure, offering exceptional efficiency and performance in reconstructing complex images, such as faces. The implementation process and experimental results are shown in Fig. 38.
![Facial speckle image reconstruction by SpT UNet network[137]. (a) SpT UNet network architecture and (b) reconstructed image results.](/Images/icon/loading.gif)
Figure 38.Facial speckle image reconstruction by SpT UNet network[137]. (a) SpT UNet network architecture and (b) reconstructed image results.
In scattering imaging technology based on DL and speckle autocorrelation, two key challenges persist. First, the phase-recovery algorithm used in speckle autocorrelation imaging is typically effective only for objects with good sparsity. This limitation makes it difficult to perform multi-target scattering imaging of non-sparse objects, which remains an urgent problem to address. Second, the quality of the autocorrelation output from the neural network heavily depends on the training data. If the autocorrelation deviates significantly from the training data, the output can contain substantial errors, which, in turn, degrade the quality of the images reconstructed by subsequent iterative phase-recovery algorithms.
Wavefront refocusing can help compensate for some of the speckle decorrelation that occurs during the iterative process of finding a suitable wavefront using an iterative algorithm. The speckle correlation method is more robust than speckle decorrelation because it requires only a single image taken by a high-definition camera to restore the image. However, both wavefront modulation and speckle correlation methods are based on memory effects, which limit the field of view of the object to the range of memory effects of the scattering medium. For strongly scattering media, the memory effect range is very small, and the speckle correlation method typically requires a large number of calculations to achieve a good reconstruction. This makes the application of both methods challenging in strongly scattering media.
(3) Deconvolution
The deconvolution method effectively addresses some of the inherent problems associated with the aforementioned methods. It allows for real-time reconstruction of the object’s image based on the speckle pattern of the object and the PSF of the scattering system. If prior knowledge of the reference object, the spatial correlation of the speckle, or an estimate of the speckle pattern can be obtained, noninvasive imaging recovery through scattering media can be achieved.
Current unblinded image restoration methods based on DL are primarily iterative approaches[142,144]. In 2017, Zhang[144] and Pan[145] integrated convolutional deep networks with traditional iterative methods, using the deep network as a denoising tool to construct an unblinded image restoration method. This approach follows the same basic principles as traditional iterative methods, with the key advantage of enhancing the overall restoration quality through the nonlinear fitting capability of an end-to-end convolutional network. The denoising network uses a fully convolutional structure, which consists of convolutional layers, rectified linear units (ReLUs), and batch normalization (BN) layers. For example, in[144], the author employs an end-to-end residual convolutional network to perform denoising after deconvolution. The network structure for denoising is shown in Fig. 39.
![The architecture of the proposed denoiser network. (a)–(e) Single image super-resolution performance comparison for Butterfly image[144].](/Images/icon/loading.gif)
Figure 39.The architecture of the proposed denoiser network. (a)–(e) Single image super-resolution performance comparison for Butterfly image[144].
In 2019, Dong et al.[146] proposed using a filter network with a U-Net structure for deconvolution, integrating the U-Net network into an iterative process. In each iteration, the U-Net network was trained with separate parameters to improve the image restoration effect. While most convolutional network parameters are real numbers, Quan et al.[147] introduced a complex value (CV) convolutional network for denoising, known as CV-CNN. This method combines inverse filtering and optimized formatting to produce high-quality restored images. It is currently considered one of the best restoration algorithms. The algorithm flow and experimental results are shown in Fig. 40.
![(a) CNN’s architecture. (b) Visual comparison of deblurring results on images “Boat” and “Couple” in the presence of AWGN with unknown strength[143].](/Images/icon/loading.gif)
Figure 40.(a) CNN’s architecture. (b) Visual comparison of deblurring results on images “Boat” and “Couple” in the presence of AWGN with unknown strength[143].
Chen et al.[148] proposed an unblind restoration method to address night scene blurred images. This method uses a confidence map to express the influence of each pixel on the restoration process. The conjugate gradient method is then applied for deconvolution, followed by the use of a deep convolutional network to suppress noise and ringing artifacts resulting from the deconvolution process. Additionally, this paper introduces a method for adaptive learning of regularization parameters through deep networks, offering a novel approach to the adaptive determination of parameters.
(4) Shower curtain effect
The shower curtain effect can transfer the diffraction pattern of an object from the front surface of a scattering medium to its back[149], making it particularly useful for addressing the scattering problems in dynamic media, where the phase distortion due to scattering is negligible. In this case, the diffraction pattern can be captured by imaging the surface of the scattering medium onto a camera sensor. By combining the bath curtain effect (PSE) with this technique, hidden objects can be reconstructed. This approach offers several advantages, including being unaffected by the OME, providing a large field of view, and demonstrating high performance with non-sparse samples. However, the original PSE method has some limitations, such as slow speed due to the need for mechanical scanning and time-consuming image reconstruction caused by iterative retrieval processes.
To address the above problems, Zhou et al.[150] proposed a deep-learning-based fast imaging method for radio pharyngeal media, PSE-Deep, which integrates ptychography and the shower curtain effect. In this method, a digital micromirror device (DMD) replaces mechanical translation, offering significant advantages by enabling the training of networks with a large number of real target data. Meanwhile, PSE-Deep uses a single-lens input mode, allowing for fast imaging without the need for scanning. Compared to traditional scanning and iterative reconstruction strategies, this method significantly improves reconstruction speed, making it more efficient for real-time applications. The principle of the experimental setup and the corresponding experimental results are shown in Fig. 41.
![(a), (b) Schematic illustration for imaging through a scattering medium. (c), (d) Schematic of the PSE-deep method. (e) The comparison of the reconstruction results from different methods[150].](/Images/icon/loading.gif)
Figure 41.(a), (b) Schematic illustration for imaging through a scattering medium. (c), (d) Schematic of the PSE-deep method. (e) The comparison of the reconstruction results from different methods[150].
However, the data-driven DL methods mentioned above often suffer from poor generalization. With the continued advancement of DL techniques, integrating physical model-driven methods has shown the potential to further enhance the model’s generalization ability and reduce its dependence on training data. In general, the generalization of the model can be improved by increasing the amount of training data and diversifying the acquisition scenarios. Imaging through dynamic scattering media can be achieved through end-to-end training[151–153]. In addition, neural networks can classify scattering media with different properties and select appropriate reconstruction subnetworks, enabling imaging of targets behind scattering media with macroscopic dynamic changes[154]. For highly dynamic scattering media, speckle patterns can be captured by reducing the exposure time. When the exposure time is reduced, the medium can be approximated as quasi-static, though this results in a decrease in the speckle signal-to-noise ratio (SNR) collected by the camera. Despite this, DL can still facilitate high-speed target reconstruction from a single frame[155]. Furthermore, combining physical models with DL can significantly reduce the amount of training data required, improving the generalization of the model[156,157].
In view of the above problems, to achieve more high-fidelity images under dynamic media, Sun et al.[154] proposed a classification image reconstruction method for dynamic scattering media based on deep learning. The proposed method consists of a classification network and a GAN reconstruction network. By iteratively training the network, the optimal solution is automatically found to achieve image reconstruction. The experimental results show that this method considerably improves the imaging performance of the transparent scattering medium. The experimental process and results are shown in Fig. 42.
![(a) Experimental setup. (b) The structure of GAN, (b1) the structure of the generative network, and (b2) the structure of the discriminative network. (c) The reconstruction results of multiple continuous shots captured with the same object under the same dynamic scattering media[154].](/Images/icon/loading.gif)
Figure 42.(a) Experimental setup. (b) The structure of GAN, () the structure of the generative network, and () the structure of the discriminative network. (c) The reconstruction results of multiple continuous shots captured with the same object under the same dynamic scattering media[154].
Due to the complex and random nature of dynamic scattering media, the mapping relationship between objects and scattering images can be automatically learned using DL networks. This approach eliminates the need to model the complex inverse problem, allowing the scattering system to be characterized explicitly or parametrically. The deep-learning-based imaging method for radiopaque scattering media offers more powerful optimization capabilities, presenting advantages over traditional methods in solving a variety of problems. It enables faster and more accurate reconstruction of the target’s structure, spectrum, and position. As the development of physics-learning coupling progresses, DL methods are expected to play an increasingly significant role in addressing different challenges in anti-scatter imaging, further enhancing the field’s capabilities.
4.1.2 Non-line-of-sight imaging
In daily life, the limitations of line-of-sight often restrict our ability to fully perceive our surroundings. However, with the advancement of technology, NLOS imaging is gradually changing this situation. This technique analyzes the diffuse reflections from relay surfaces to reconstruct hidden objects and has demonstrated significant potential in fields such as medical imaging and remote sensing[158–160].
NLOS imaging can be categorized into two main types based on whether or not a controllable light source is used: active[161–163] and passive[164–167], as shown in Fig. 43. Active NLOS imaging relies on actively emitting light sources, such as lasers or LEDs, to illuminate the target area. Hidden objects are then reconstructed by receiving light signals that are reflected or scattered back. This method typically results in high-resolution images and is highly resistant to interference. In contrast, passive NLOS imaging does not require an active light source. Instead, it captures natural light from the environment or scattered light from other existing sources. The advantages of passive NLOS imaging include good covertness, low energy consumption, and suitability for scenarios where using an active light source is impractical.
![Active and passive NLOSs[161–167" target="_self" style="display: inline;">–167].](/Images/icon/loading.gif)
Figure 43.Active and passive NLOSs[161–167" target="_self" style="display: inline;">–167].
The concept of NLOS imaging was first introduced by Raskar and Davis in 2008[168]. In recent years, researchers have proposed various NLOS imaging solutions based on different principles[169–176], including time-correlated single-photon counting (TCSPC)[177–180], acoustical methods[181], passive imaging[182], interferometry[175,183], thermography[184,185], and fluctuation methods[186–188]. In addition, several image reconstruction techniques have been developed to address NLOS imaging challenges, such as occlusion-aware light reversal methods[189–193], linear inverse methods[194,195], inverse projection methods[196,197], data-driven algorithms[198–200], and deconvolution methods[178,179]. These methods have contributed to solving various problems in NLOS imaging and advancing the field.
Among the various techniques, time-of-flight (ToF)[177,201,202] based NLOS imaging methods offer high-ranging accuracy and real-time imaging capabilities, making them suitable for dynamic scenes. However, they are highly susceptible to ambient light interference in complex NLOS environments and also require high equipment accuracy and cost. Alternatively, imaging based on the spatial coherence of light scattered from rough surfaces[165] recovers information about hidden objects by analyzing the scattering patterns formed by the scattered light. While this method offers better anti-interference capabilities, it requires high coherence of light, involves highly complex imaging processing, demands significant computational resources, and suffers from poor real-time performance. In this context, traditional physically oriented recovery algorithms exhibit certain limitations. However, DL methods, with their powerful data-driven capabilities, significantly improve NLOS imaging. By learning from large datasets representing complex scenarios, DL overcomes the challenges faced by traditional physical model recovery algorithms, especially in highly nonlinear systems, and demonstrates great potential for practical applications.
(1) ToF-based method for NLOS imaging
Typically, ToF-based systems consist of a pulsed laser transmitter, a beam navigator, and a synchronized single-photon avalanche diode (SPAD) detector or streak camera. By using the ToF information, the position and topographic features of hidden objects can be extracted based on geometric optics. However, these systems are vulnerable to ambient light interference in complex scenes, and their time efficiency is compromised by high computational demands due to the complexity of data processing, particularly with the multiple reflections of optical signals. DL technology effectively compensates for these limitations by learning and optimizing the imaging algorithm directly from the data. This reduces the time required for manual adjustments and signal processing, significantly improving the system’s time efficiency while maintaining high accuracy and robustness. As a result, DL shows great potential for a wide range of applications in complex targets and dynamic environments.
In 2020, Isogawa et al.[203] proposed an optical-based NLOS 3D human posture estimation method. This approach simulates the propagation and scattering behaviors of light in complex environments, constructs a physical model, and combines optimization algorithms. By integrating techniques such as NLOS imaging, human pose estimation, and deep reinforcement learning, the method infers 3D human poses in non-visible regions using indirect light. The results of this approach are shown in Fig. 44. However, the accuracy of this imaging is often limited by the material properties of the target in the non-visible domain. To address this, in 2021, Shen et al.[204] introduced a neural modeling framework for non-visible imaging, represented by the neural transient field (NeTF), implemented using a multilayer perceptron. Experiments demonstrated that NeTF achieves state-of-the-art performance and provides reliable reconstruction, even in scenarios involving semi-obscured or non-Lambertian materials[205]. In 2023, Liu H et al.[206] proposed a new polarized infrared NLOS (PI-NLOS) imaging method. This method utilizes a polarized infrared scattering pattern generated by an active light source. By analyzing the reflected rays in different polarization states, the method enhances contrast and detail, significantly improving the accuracy and robustness of the imaging system.
![(a) Non-line-of-sight (NLOS) physics-based 3D human pose estimation. (b) Isogawa M DeepRL-based photons to 3D human pose estimation framework under the laws of physics[203].](/Images/icon/loading.gif)
Figure 44.(a) Non-line-of-sight (NLOS) physics-based 3D human pose estimation. (b) Isogawa M DeepRL-based photons to 3D human pose estimation framework under the laws of physics[203].
In addition to line-of-sight 3D imaging, researchers have made significant advances in NLOS dynamic imaging to achieve higher accuracy and robustness. In 2020, Metzler et al.[170] proposed a deep-inverse correlography method. This approach uses spectral estimation theory to derive a noise model for NLOS correlation imaging and combines this physical model with a DL inversion algorithm. The result is a scatter-correlation-based technique for recovering occluded objects from indirect reflections, enabling high-resolution, real-time NLOS imaging. The following year, Cao et al.[207] introduced steady-state NLOS imaging based on dynamic excitation, as shown in Fig. 45. They used multi-branch CNNs for image reconstruction, achieving high-precision NLOS imaging in dynamic environments. This method effectively addresses time-dependent challenges in NLOS imaging, improving both the accuracy and robustness of the imaging process.
![Overview of the proposed dynamic-excitation-based steady-state NLOS imaging framework[207].](/Images/icon/loading.gif)
Figure 45.Overview of the proposed dynamic-excitation-based steady-state NLOS imaging framework[207].
(2) Spatial-coherence-based method for NLOS imaging
Scattering, as a manifestation of the spatial coherence function, offers a unique advantage in target information acquisition and decoding. Due to the memory effect[208,209], images of objects around corners can be directly recovered from the autocorrelation of the scattering pattern using a phase-recovery algorithm[175]. However, this technique is effective only within a small field of view (FoV), which is determined by the range of the memory effect. Additionally, the phase-recovery process is often ill-posed, leading to high uncertainty in the results[210–213]. Compared to ToF-based imaging methods, scattering correlation-based imaging techniques have gained significant popularity in the field of NLOS imaging. This is due to their ability to provide richer spatial coherence information[19,21]. Furthermore, when combined with the powerful high-dimensional feature extraction and nonlinear modeling capabilities of DL, speckle-correlation-based imaging techniques have been rapidly advancing in the field of non-line-of-sight imaging.
In 2021, Zheng et al.[214] introduced a white-light NLOS imaging method that incorporates scattering correlation-based models into a DNN, and the structure is shown in Fig. 46. This method uses an ordinary camera under passive white-light illumination to acquire target scattering information. It then applies a two-step DNN strategy: one step to learn the scattering pattern autocorrelation and the other to optimize the target image reconstruction. In 2023, He et al.[215] proposed a two-input U-Net-based passive NLOS imaging reconstruction method, which utilizes a DL algorithm to capture scattered light information from the environment and perform image reconstruction. In the same year, Yu et al.[216] introduced a passive NLOS imaging method based on a channel-aware GAN (CAGAN). This method achieved high-precision image reconstruction by analyzing scattered light information from different channels using a GAN. Additionally, Wang et al.[217] proposed a scattering-based position and image recognition network (SPIR-Net). This network reconstructs images using a DL algorithm by analyzing the environmental reflected and scattered light information. The data is processed through a CNN, which extracts features from the light-scattering data to reconstruct a 3D static image of the target and determine its position.
![(a) The structure of the two-step DNN strategy. (b) The corresponding cropped speckle patterns, their autocorrelation, and the reconstructed images with the proposed two-step method[214].](/Images/icon/loading.gif)
Figure 46.(a) The structure of the two-step DNN strategy. (b) The corresponding cropped speckle patterns, their autocorrelation, and the reconstructed images with the proposed two-step method[214].
In 2024, Wang et al.[218] proposed a passive NLOS imaging framework that utilizes in-sensor computation for dynamic light-field feature extraction. This was accomplished by integrating event-based vision techniques into NLOS imaging. The framework combines event streams with intensity information, thereby enhancing the reconstruction performance in dynamic NLOS scenes. Additionally, it offers a novel approach to utilizing limited real-world datasets for passive NLOS imaging, which is crucial for real-world applications with scarce data. In the same year, Wu et al.[219] introduced a novel reconstruction method known as the physical constraint inverse network (PCIN). This method combines physical constraints with DL by leveraging the relationship between PSF constraints and CNN optimization. The approach constructs an accurate PSF model and an inverse physical model. The diffuse reflection angle and neural network parameters within the PSF model are optimized by alternating between iteration and gradient descent algorithms, leading to high-quality reconstructed images. The underlying principle of this approach is illustrated in Fig. 47.
![Flowchart of PCIN algorithm for NLOS imaging reconstruction[219]. The speckle image captured by the camera is put into CNN, and PCIN iteratively updates the parameters in CNN using the loss function constructed by the speckle image and forward physical model. The optimized parameters are utilized to obtain a high-quality reconstructed image.](/Images/icon/loading.gif)
Figure 47.Flowchart of PCIN algorithm for NLOS imaging reconstruction[219]. The speckle image captured by the camera is put into CNN, and PCIN iteratively updates the parameters in CNN using the loss function constructed by the speckle image and forward physical model. The optimized parameters are utilized to obtain a high-quality reconstructed image.
4.1.3 Polarimetric descattering
Clear vision in scattering media is crucial for various applications, including industrial and civil applications[220], traffic monitoring systems[221], autonomous driving[222], remote sensing[223], rescue operations[224], undersea mapping[225], marine species migration, coral reef monitoring, and scene analysis[226]. However, capturing images in a scattering environment presents significant challenges. The backscattered light mixes with the target signal as it propagates toward the camera, leading to a dramatic decrease in image visibility. Fortunately, backscattered light is partially polarized, which offers a solution. By employing a polarized imaging system, the image quality and contrast can be significantly enhanced[227–229].
(1) Dehazing
The polarization dehaze algorithm, based on the atmospheric scattering model, utilizes the atmospheric optical properties and polarization information to mitigate or eliminate the haze effect present in an image. The goal of haze removal is to analyze information from different polarization channels in the image, extract the polarization components, and then infer the true characteristics of the scene. In recent years, DL has been introduced into the field of image defogging as a powerful data-driven approach to overcome the limitations of traditional methods[230,231]. DL models can learn complex features and defogging patterns from large datasets, enabling them to address image defogging challenges across a variety of complex scenes and lighting conditions. These models demonstrate strong adaptive and generalization capabilities, making them more effective than conventional techniques[232–234].
In 2020, Zhang et al.[235] proposed an unsupervised deep network to fuse intensity and degree of linear polarization (DoLP) images. This method uses a feature extraction module to convert images of zero-degree polarization maps and DoLP into high-dimensional nonlinear feature maps. A stitching operator is then applied to fuse these feature maps, reconstructing the fused image. In 2022, Shi et al.[232] introduced a polarization-based self-supervised dehazing network that combines polarization information with DL. This method requires only two frames of the scene with orthogonal polarization state images as input to remove smoke from the scene through online training. Compared to similar methods, this approach improves the visibility of target details more effectively and demonstrates strong robustness across different scenes. Later that year, Zhang J et al.[236] proposed a cyclic CNN (CCNN). This network fuses the smoke-free region generated by a sub-network with the original visible smoke polarized image. The fused image is then reintroduced as new input to the model, recovering the final clear image. In 2024, Suo et al.[237] introduced an improved defogging algorithm that combines polarization properties and DL. The method enhances the accuracy of transmission map estimation by improving the YOLY network architecture, incorporating a multiscale module and an attention mechanism in the transmission feature estimation network. This approach extracts feature information and applies weight allocation at different scales, significantly improving the defogging of polarized images. The different network architectures and corresponding experimental results are shown in Fig. 48.
![(a) Network architecture and result of Ref. [235]. (b) Network architecture and result of Ref. [237].](/Images/icon/loading.gif)
Figure 48.(a) Network architecture and result of Ref. [235]. (b) Network architecture and result of Ref. [237].
(2) Underwater descattering
Underwater descattering and image clarification techniques are of significant academic and practical value in various fields such as marine scientific research, diving activities, and underwater engineering[238,239]. However, the visibility and clarity of underwater imaging are severely constrained by the substantial scattering and absorption of light in the underwater environment[240]. Various underwater image recovery methods based on polarization imaging have been proposed[241–245] to establish a mapping between polarization information and target signals in clear water. However, these methods often deviate from practical applications due to the challenges posed by real-world underwater environments. DL, as a powerful neural-network-based technique, has shown great promise in underwater polarization imaging, particularly in high-density turbid water[231,246,249].
In 2020, Hu’s team at Tianjin University[247] was the first to apply polarization to underwater complex imaging environments, introducing the polarimetric residual-dense network (PDN), the structure as shown in Fig. 49. This method demonstrates that the descattering effect achieved by using multi-dimensional polarization information as input is significantly superior to the effect obtained by using light intensity images alone. The network structure is illustrated in the figure. Additionally, to improve image quality under low-light conditions, Hu’s team introduced polarization information to establish the first-ever low-light polarization imaging dataset[248]. They proposed a three-layer hybrid network capable of synchronously enhancing and balancing the image quality across intensity, DoLP, and angle of linear polarization (AoLP).
![Network architecture and result of PDN[247].](/Images/icon/loading.gif)
Figure 49.Network architecture and result of PDN[247].
In 2022, Xiang et al.[250] introduced a novel four-input polarization recovery residual-dense network (PRDN) for highly turbid water bodies, as shown in Fig. 50(a). By combining the PRDN with a residual image recognition network (ResNet34), they constructed an integrated model for recognition, classification, and restoration. This approach demonstrated superior polarization image restoration, especially in environments where polarization information is severely degraded. In the same year, Ren et al.[251] proposed a lightweight CNN that achieved clear imaging in underwater environments with varying turbidity. This was done by fusing two key parameters from the polarization descattering model into a single parameter. However, many existing networks heavily rely on data-driven techniques and do not incorporate physical principles or real-world physical processes. To address this limitation, Gao et al.[252] proposed the Mueller transform matrix network (MTM-Net) for recovering underwater polarization images [Fig. 50(b)]. This approach integrates the physical dehazing model based on the Mueller matrix, significantly improving image quality through the use of an inverse residual and channel attention structure while maintaining the integrity of the image recovery process. The inclusion of polarization information extends the dimensionality of the image data and enhances the recovery of the target from 2D to 3D. Yang K et al.[5] proposed a polarization-polarized 3D reconstruction method for turbid water, as shown in Fig. 50(c). They designed an end-to-end underwater polarization-enhanced scattering network that generates clear underwater images in turbid environments and enhances the texture details of objects in such environments. The method estimates the texture details of underwater objects at the pixel level with high accuracy and calculates the DoLP and AoLP of the objects with precision. Ultimately, this approach yields high-quality 3D image reconstruction of water body targets.
![(a) Network architecture and result of PRDN[245]. (b) Network architecture and result of MTM-Net[252]. (c) Network architecture and result of underwater descattering network[5].](/Images/icon/loading.gif)
Figure 50.(a) Network architecture and result of PRDN[245]. (b) Network architecture and result of MTM-Net[252]. (c) Network architecture and result of underwater descattering network[5].
4.1.4 Range-gated imaging
Laser range-gated imaging technology utilizes the time-of-flight range-gated principle, employing pulsed lasers and range-gated cameras to separate scattered stray light from reflected light at different distances. This allows the camera to capture the radiation pulse reflected from the target scene within a specific open range-gated time window. Unlike traditional imaging technology, laser range-gated imaging selectively images the target within a specified distance interval, filtering out media scattering noise between the region of interest and the imaging system, as well as background noise outside the region of interest. This selective imaging improves both distance accuracy and image quality. Laser range-gated imaging offers several advantages, including better environmental adaptability, higher resolution, high real-time performance, and long-range capabilities. These advantages make it widely applicable in fields such as three-dimensional mapping, long-distance reconnaissance, navigation, and underwater target detection.
(1) Underwater range-gated imaging
Laser range-gated imaging is one of the most effective methods for achieving high-resolution underwater imaging. However, forward scattering in the underwater environment alters the direction of light, resulting in blurred images of the target. Additionally, the combined effects of scattering and blur lead to a degraded image quality in underwater laser range-gated imaging. To address this issue, integrating DL methods to enhance imaging quality has become one of the primary research directions.
To address the blurring problem in underwater laser range-gated imaging, Lin et al.[253] considered the combined effects of forward scattering and defocusing blurring on imaging results. They proposed a deep-learning-based method to de-blur laser range-gated images. This method effectively removes blur of varying degrees and can handle both clear and blurred target images simultaneously. The network structure and experimental results are shown in Fig. 51. For underwater target feature extraction, Zhang et al.[254] developed a fishing net detection and recognition method using underwater laser range-gated imaging. The method first acquires high-quality images of underwater fishing nets using laser range-gated imaging, then extracts features from these images through a dual-phase training strategy. This strategy includes a mask-guided feature extraction phase followed by classification fine-tuning. Experimental results show that this method can effectively recognize underwater fishing nets, helping underwater vehicles avoid entanglement.
![Network architecture and experimental results of Lin et al.[253] (a) Proposed network architecture. (b) Results of image deblurring experiments.](/Images/icon/loading.gif)
Figure 51.Network architecture and experimental results of Lin et al.[253] (a) Proposed network architecture. (b) Results of image deblurring experiments.
In underwater laser range-gated imaging, image blurring caused by forward scattering and defocusing significantly impacts imaging quality. However, deep-learning-based methods can effectively enhance imaging quality by mitigating these issues. This approach holds great potential for applications in fields such as underwater detection, underwater navigation, and other related areas.
(2) Three-dimensional laser range-gated imaging
The laser range-gated imaging technique is widely implemented using the time-of-flight method, which enables the acquisition of spatial slices of the scene. This allows for the capture of numerous time-sliced images, enabling three-dimensional imaging. However, traditional 3D range-gated imaging techniques require processing a large number of time-sliced images, which results in poor real-time performance. Additionally, these methods have stringent hardware limitations on various gain modulation techniques. By introducing DL approaches, it is possible to overcome the constraints of traditional methods and hardware limitations, enabling high-quality real-time imaging.
To address the problem of time-consuming 3D range-gated imaging, Gruber et al.[255] proposed a CNN approach to solve the challenges of 3D range-gated imaging from a visual perspective. This method aims to enhance the efficiency of the imaging process, enabling faster results. The network structure and experimental results are shown in Fig. 52. Meanwhile, Liu et al.[256] tackled the issue of instability in existing DL methods caused by incomplete training data. They introduced a Bayesian neural network to extend the Gated2Depth framework. This approach addresses the problem of depth maps by incorporating a method to expand the recognition of uncertainty, which helps explain unknown data and improves the model’s accuracy.
![Comparison of Gated2Depth network architecture and experimental results[255–257" target="_self" style="display: inline;">–257]. (a) Gated2Depth network architecture. (b) Comparison of experimental results of different methods.](/Images/icon/loading.gif)
Figure 52.Comparison of Gated2Depth network architecture and experimental results[255–257" target="_self" style="display: inline;">–257]. (a) Gated2Depth network architecture. (b) Comparison of experimental results of different methods.
In 3D range-gated imaging techniques, traditional distance-intensity correlation algorithms require specific distance-intensity curves, making them difficult to implement. Moreover, existing deep-learning-based algorithms often require large amounts of real-world training data, which can be challenging to obtain. To address these issues, Xia et al.[257] proposed a distance-intensity-profile guided range-gated ranging and imaging method based on a CNN. This method first obtains the range intensity profiles for a given range-gated optical ranging and imaging system. It then uses synthetic data for training and fine-tuning with the range intensity profiles. The network learns both semantic depth cues and range intensity depth cues from the synthetic data, while also learning accurate range intensity depth cues from real range intensity profile data. The experimental results demonstrate that this method outperforms others in both real-world scenarios and comprehensive test datasets.
Compared with traditional range-gated 3D imaging, combining DL can effectively break through the hardware limitations and acquire high-quality images. Simultaneously, deep-learning-based methods have higher prediction accuracy and stronger anti-interference ability. Therefore, the effective use of DL and artificial intelligence algorithms can realize the synergistic optimization between different system hardware, better exploit the upper performance limit of the hardware and expand the functional dimension and information dimension of the imaging system.
4.1.5 Photoacoustic imaging
Photoacoustic imaging (PAI) is an emerging non-invasive photonic imaging technique widely used in disease detection and diagnosis, as well as in the observation of biological tissue structure and function. The underlying physics of PAI is based on the photoacoustic effect in biological tissues. When the imaging sample is exposed to short-pulse laser light emitted by a laser source, the tissue or material absorbs the light energy and undergoes thermoelastic expansion, leading to instantaneous expansion and contraction of the surrounding medium, thus generating ultrasound waves. These ultrasound waves propagate towards the surface of the tissue and are detected by sensors. By solving the inverse acoustic problem from the received ultrasound signals, the initial pressure distribution on the tissue surface can be reconstructed, enabling the visualization and diagnosis of the tissue’s structure and function[258,259]. Due to the difference in scattering intensity between ultrasound and photons in biological tissues, which spans approximately two to three orders of magnitude, photoacoustic imaging can overcome the optical diffraction limit (about 1 mm) in imaging depth. Furthermore, PAI not only benefits from the depth advantage of ultrasound imaging, but also combines the high contrast and high resolution of optical imaging, allowing for high-depth, high-contrast, and high-resolution imaging of biological tissues. In the field of medical imaging, PAI holds great promise for applications, as it combines acoustic penetration depth, optical resolution, and non-invasive characteristics, offering numerous advantages for a wide range of potential uses.
However, photoacoustic imaging still faces many challenges, including imaging quality limitations imposed by acoustic and optical diffraction, as well as various issues in data acquisition, processing, and inversion. For example, in photoacoustic tomography (PAT), it is difficult to simultaneously achieve low-cost devices and high-signal-to-noise ratio (SNR) image reconstruction. Additionally, widely used sparse detectors often fail to produce ideal reconstruction results using conventional inversion methods. In photoacoustic microscopy (PAM), there are also shortcomings in imaging speed. Although the scanning rate can be increased by altering the excitation pulse repetition rate and changing scanning mechanisms, these approaches often have a non-negligible impact on image quality. In short, photoacoustic imaging currently involves a trade-off between imaging quality, cost-effectiveness, and time efficiency. Although many methods have been proposed to address these issues and have shown some success, further exploration and improvement are still needed. Deep learning methods aim to discover complex mappings from training data to optimize existing parameter space problems. With the past limitations of computational power, current advancements in the graphics processing unit (GPU) capabilities now allow neural networks to continually improve in terms of depth[260,261], width[262], and computational speed, gradually leading to the development of various network architectures. Deep learning has become a key method in fields such as computer vision, natural language processing, and artificial intelligence. At present, many deep learning networks, including convolutional neural networks (CNNs), U-shaped networks (U-Net), and generative adversarial networks (GANs), have been widely applied in various aspects of photoacoustic image reconstruction[263–265], with significant body of research demonstrating the superiority of these methods.
In recent years, photoacoustic microscopy (PAM) technology has made significant progress in quantitative and anatomical imaging of skin vascular lesions. Through non-invasive photoacoustic microscopic biopsy methods, researchers were able to capture the vascular structure and hemodynamic parameters of the dermal layer with high precision. The research team developed an advanced photoacoustic microscopic biopsy technique, enabling the quantitative and anatomical imaging of dermal vascular lesions[266]. This method not only provided high-resolution vascular images but also accurately measured vascular density and blood flow velocity, offering reliable imaging evidence for the early diagnosis and monitoring of skin diseases, such as psoriasis and lupus erythematosus. Based on this, the application of photoacoustic imaging technology was further expanded. Through multi-scale confocal photoacoustic skin imaging technology, a comprehensive assessment of skin health status was achieved[267]. The study combined micro and millimeter-scale imaging, allowing the simultaneous capture of multi-level structural information from both microvessels and macroscopic vascular networks. This multi-scale imaging strategy significantly enhanced diagnostic accuracy and detail, while effectively reducing artifacts and noise during the imaging process, thereby improving the signal-to-noise ratio of the images. This provided a more detailed and reliable method for clinical skin health assessment. With the continuous advancement of technology, three-dimensional imaging techniques in photoacoustic microscopy have been studied in depth. The research team introduced an auto-focusing acousto-optic probe, significantly improving the imaging capability of three-dimensional confocal photoacoustic skin microscopy[268]. The auto-focusing feature ensured high-resolution imaging at various depths and complex skin structures, maintaining the accuracy and consistency of three-dimensional reconstruction. This innovative technology not only improved imaging efficiency but also provided strong support for the three-dimensional diagnosis and dynamic monitoring of complex skin lesions.
Building on the success of multi-scale confocal photoacoustic skin microscopy in enhancing the diagnostic accuracy of skin diseases, the research team introduced time-resolved photoacoustic microscopy (TR-PAM) to extend the application of photoacoustic imaging to the detection of microvascular calcification (MC)[269]. The operational workflow of time-resolved photoacoustic microscopy (TR-PAM) and its imaging mechanism based on phase principles is illustrated in Fig. 53. By leveraging the high temporal resolution of TR-PAM, it became possible to precisely observe and document the subtle structural changes in microvessels induced by calcification. This novel, non-invasive approach offered a significant advancement in the early detection of cardiovascular and metabolic diseases.
![Underlying principle of TR-PAM and theoretical prediction of PA response[269]. (a) Laser-induced thermoelastic displacement (u) and subsequent PA response based on the optical-absorption-induced thermoelastic expansion for vascular tissues. (b) The principle of TR-PAM: elasticity and calcification estimations from vascular PA time response characteristics. (c) Experimental setup of the TR-PAM.](/Images/icon/loading.gif)
Figure 53.Underlying principle of TR-PAM and theoretical prediction of PA response[269]. (a) Laser-induced thermoelastic displacement (u) and subsequent PA response based on the optical-absorption-induced thermoelastic expansion for vascular tissues. (b) The principle of TR-PAM: elasticity and calcification estimations from vascular PA time response characteristics. (c) Experimental setup of the TR-PAM.
Against the backdrop of ongoing advancements in photoacoustic microscopy (PAM) to improve imaging depth and resolution, the research team proposed an adaptive multi-layer photoacoustic image fusion method (AMIPF) to address the challenges posed by variations in the point spread function (PSF) in multi-layer imaging[270]. By integrating blind deconvolution and image registration techniques, AMIPF efficiently optimized multi-layer image fusion and resolution enhancement without relying on prior PSF information. Experimental validation demonstrated that this method could rapidly extract high-quality photoacoustic images and significantly outperformed traditional approaches in terms of contrast enhancement and structural restoration. This work not only provided essential technological support for high-contrast imaging of deep tissues but also extended the applicability of photoacoustic microscopy to complex multi-layer biological samples, opening new possibilities for biomedical research and clinical practice.
Photoacoustic dermoscopy (PAD), as a non-invasive imaging technology, has demonstrated significant potential in achieving high-resolution and deep imaging of skin tissues. However, the quantification and high performance of PAD systems face several challenges, such as the interplay between optical scattering and acoustic attenuation in complex skin structures and the limitations of hardware sensitivity and resolution. A study proposed a 4D spectral–spatial computational PAD model, which integrates theoretical computation, experimental optimization, and deep learning to comprehensively enhance the imaging quality of PAD systems[271]. The study first constructed a photoacoustic computational framework based on a seven-layer skin model, encompassing heterogeneous multilayer structures such as the stratum corneum, epidermis, dermis, and subcutaneous fat. The framework employs the Monte Carlo method to precisely simulate light propagation in skin tissues while incorporating the k-Wave tool to calculate the backpropagation of ultrasound waves. This approach enables the quantitative analysis of the interaction between optical and acoustic fields and provides theoretical guidance for PAD system design. The core workflow is clearly illustrated in Fig. 54.
![The process of using the 4D spectral–spatial computational PAD combined with experiments for dataset acquisition and system optimization for deep learning[271]. (a) Relevant parameters can be set before data acquisition, and the distribution of the model optical field and detector acoustic field under a collimated Gaussian beam in the model is shown. (b) Feedback on relevant performance optimization parameters is provided to the experimental system after simulating calculations. (c) Experimental system. (d) The dataset is used for training the spread-spectrum network model. (e) The dataset is used for training the depth-enhanced network model. (f) The low-center-frequency detector skin imaging results obtained in the experiment are input into the trained spread-spectrum model to obtain the output image. (g) The skin imaging results under conventional scattering obtained in the experiment are input into the trained depth-enhanced model to obtain the output image.](/Images/icon/loading.gif)
Figure 54.The process of using the 4D spectral–spatial computational PAD combined with experiments for dataset acquisition and system optimization for deep learning[271]. (a) Relevant parameters can be set before data acquisition, and the distribution of the model optical field and detector acoustic field under a collimated Gaussian beam in the model is shown. (b) Feedback on relevant performance optimization parameters is provided to the experimental system after simulating calculations. (c) Experimental system. (d) The dataset is used for training the spread-spectrum network model. (e) The dataset is used for training the depth-enhanced network model. (f) The low-center-frequency detector skin imaging results obtained in the experiment are input into the trained spread-spectrum model to obtain the output image. (g) The skin imaging results under conventional scattering obtained in the experiment are input into the trained depth-enhanced model to obtain the output image.
4.2 Improved imaging resolution
4.2.1 Ptychographic imaging
Ptychographic imaging is an advanced coherent diffraction imaging (CDI) technique that reconstructs three-dimensional images by superimposing data from multiple facets. With the rise of DL, researchers have progressively integrated it with ptychographic imaging, leading to significant advancements in both traditional ptychographic imaging and Fourier ptychographic imaging. DL techniques can effectively reduce the impact of noise and artifacts and substantially enhance the resolution of imaging, enabling more precise and detailed reconstructions.
(1) Typical ptychographic imaging
Ptychographic imaging uses a set of offset local illuminations to capture multiple diffraction patterns of the sample, which are then used as the basis for image reconstruction. This method generates a large amount of data during the image acquisition process, making the adoption of DL models highly feasible. As a super-resolution imaging technology, ptychography has particularly demanding requirements, one of which is the high image overlap rate. To overcome this limitation, Guan et al.[272] proposed the ptychography network (Ptycho Net) in 2019, a deep-learning-based method. This approach enables phase retrieval in ptychographic imaging in a non-iterative manner, allowing image reconstruction even with extremely sparse scanning. Similarly, in 2023, Pan et al.[273] introduced and improved a neural network model, fine-tuning it using various datasets. This significantly enhanced the reconstruction performance of ptychographic imaging under different overlap rates. The PtyNet-S network architecture and corresponding experimental prediction results are shown in Fig. 55. To further improve resolution, Lv et al.[274] proposed a resolution-enhanced ptychography framework (REPF) in 2022. This framework utilizes a forward model based on an a priori physical model, enabling a doubling of spatial resolution compared to traditional methods under the same conditions.
To address the challenges of high computational cost and complexity, Gan et al.[275] proposed ptychography deep vision (PtychoDV) in 2024. This method uses visual converters to generate initial images, followed by a learnable convolutional prior and measurement model to optimize the initial image. Compared to traditional iterative methods, this approach significantly reduces computational cost. Building on the challenge of computational difficulties in phase recovery, Cherukara et al.[276] proposed the ptychography neural network (PtychoNN). The network learns the mapping relationship between far-field diffraction data and the amplitude and phase of the real sample image, which further accelerates image acquisition and reconstruction speed.
![PtyNet-S network architecture and experimental prediction results[273]. (a) Structure of PtyNet-S. (b) Phase reconstruction results of the PtyNet-S in tungsten test pattern.](/Images/icon/loading.gif)
Figure 55.PtyNet-S network architecture and experimental prediction results[273]. (a) Structure of PtyNet-S. (b) Phase reconstruction results of the PtyNet-S in tungsten test pattern.
The application of DL methods to traditional ptychographic imaging has led to significant improvements in both image quality and processing efficiency. Through techniques like denoising and image enhancement, the resolution of the images is enhanced, and the reconstruction process is optimized. Moving forward, traditional ptychographic imaging should continue to focus on enhancing computational speed and imaging resolution while integrating DL techniques in data processing and algorithm development. This integration will facilitate the exploration of more efficient and effective methods for image reconstruction.
(2) Fourier ptychographic imaging
Fourier ptychography (FP) is a super-resolution imaging technique developed in recent years. Its principle is based on the Fourier transform, utilizing the characteristics of light waves to convert spatial information of objects into frequency-domain information. During the imaging process, the spectrum containing object information is collected and analyzed to reconstruct a clear image of the object.
Fourier ptychographic microscopy (FPM) is an extension and application of Fourier ptychography in the field of microscopy. It enables large-field-of-view imaging, high spatial resolution, and quantitative phase imaging (QPI). The combination of FP and DL can further optimize the reconstruction process, enhancing both reconstruction efficiency and image quality, making it a promising approach for advancing imaging capabilities.
Optimized reconstruction process. The phase-recovery algorithm plays a critical role in the reconstruction results, but if the system structure is misaligned, it becomes challenging to reconstruct the image without professional adjustment. In 2022, Bianco et al.[277] proposed a network framework based on conditional GAN (cGAN) to address the issue of significant misalignment. This approach enables better alignment handling and improves image reconstruction despite misalignment. In the same year, Chen et al.[278] introduced the FPM reconstruction method using untrained DNN priors (FPMUP). This method helps eliminate the ringing effect caused by spectrum truncation in the image by predicting the spectrum outside the pupil. In addition, Yang et al.[279] proposed the Fourier ptychography multi-parameter neural network (FPMN), which models optical parameters in the forward imaging model into different network layers. This method addresses the challenges posed by high requirements for precise LED positioning, accurate focus, and appropriate exposure times.
Aberrations generated by optical components in FPM imaging systems can significantly degrade image quality. To address this issue, Sun et al.[280] proposed a novel FPM reconstruction model called the “Forward Imaging Neural Network with Pupil Recovery (FINN-P)” in 2019. By combining pupil recovery with the forward imaging process in a TensorFlow-based FPM, this method significantly improves reconstruction results, even in the presence of large aberrations. In 2023, Bouchama et al.[281] trained a two-channel U-Net artificial neural network that provides both intensity and phase information. By constructing specialized training datasets, the network enhances the contrast and resolution of the target composition at the subcellular scale. This method addresses the challenges of resolution, color accuracy, and coherence artifacts in color reconstruction in FPM imaging. In 2020, Wang R et al.[282] proposed a cycle-consistent adversarial network (CycleGAN) with multiscale structure similarity loss. This network was trained using a set of monochromatic FPM reconstructed images and a set of color images captured by a microscope. During the inference stage, the FPM image input is processed by the network, which outputs a virtual colored image, enabling virtual brightfield and fluorescent staining of the FPM image. This approach helps reduce coherence artifacts and significantly improves image quality. The CycleGAN architecture and corresponding reconstruction results are shown in Fig. 56.
![Using networks to reduce color artifacts and improve image quality for different slices[282]. (a1), (a2) FPM raw data. (b1), (b2) Network input. (c1), (c2) Network output. (d1), (d2) FPM color image. (e1), (e2) True value. (f) Train two generator-discriminator pairs using two mismatched image sets. (g) Generator A2B accepts FPM input and outputs almost stained images.](/Images/icon/loading.gif)
Figure 56.Using networks to reduce color artifacts and improve image quality for different slices[282]. (a1), (a2) FPM raw data. (b1), (b2) Network input. (c1), (c2) Network output. (d1), (d2) FPM color image. (e1), (e2) True value. (f) Train two generator-discriminator pairs using two mismatched image sets. (g) Generator A2B accepts FPM input and outputs almost stained images.
![Network architecture and results comparison[285]. (a) Network architecture combined with two models. (b) Amplitude contrast. From left to right, the original image is restored using GS algorithm, MFP algorithm, and the algorithm proposed in this review. (c) Phase contrast. From left to right, the original image is restored using GS algorithm, MFP algorithm, and the algorithm proposed in this review.](/Images/icon/loading.gif)
Figure 57.Network architecture and results comparison[285]. (a) Network architecture combined with two models. (b) Amplitude contrast. From left to right, the original image is restored using GS algorithm, MFP algorithm, and the algorithm proposed in this review. (c) Phase contrast. From left to right, the original image is restored using GS algorithm, MFP algorithm, and the algorithm proposed in this review.
In 2019, Shamshad et al.[283] proposed a method that combines pre-trained reversible generation models with untrained generation models. The pre-trained reversible generation model helps mitigate representation errors, while the untrained generation model reduces dependence on large amounts of training data. Experiments demonstrated the feasibility of achieving FP super-resolution under low sampling rates and high noise levels. The network architecture and results are shown in the following figure. In 2023, Bianco[284] proposed the blind multi-look FP (ML-FP) technique, combining it with the DL network cGAN. This method accelerates phase retrieval and reconstructs high-resolution images even in the case of dislocations. In the same year, Sha et al.[285] introduced a dual-structured prior neural network model that leverages inherent prior information in the network to reconstruct FP images beyond the system’s numerical aperture. This method performs well under conditions of low sampling rates and high noise levels, showcasing the effectiveness of pre-trained generation models in FP image reconstruction. However, these methods face challenges due to the limited characterization ability of pre-trained generation models and the need for large, fully observed specific images as training samples, which makes their application in FP difficult. The comparison of network architecture and results is shown in Fig. 57. To address the shortcomings of FP iterative methods, which are prone to falling into local minimum solutions and are sensitive to noise, Li et al.[286] proposed the Single Implicit Neural Network (SCAN) in 2024. This method cleverly associates target coordinates with amplitude and phase within a unified network in an unsupervised manner, achieving accurate predictions of both target amplitude and phase. The network outperforms traditional DL models in terms of accuracy and robustness.
By leveraging the powerful nonlinear fitting and computational capabilities of DL, FP can effectively handle the interference caused by external factors such as hardware defects and noise during the reconstruction process. This integration greatly optimizes the reconstruction process and minimizes the impact of defects in optical system components. As a result, image quality is preserved while other interferences are effectively eliminated.
Improve reconstruction efficiency. Combining FP with DL can effectively address its inherent limitations, particularly when dealing with large datasets and complex algorithms that lead to low efficiency, long reconstruction times, and other challenges. These issues have traditionally hindered the ability to perform real-time imaging. By incorporating DL, these shortcomings can be mitigated to some extent, enabling faster and more efficient image reconstruction.
FPM requires a high overlap rate for a series of low-resolution images, which poses a challenge for acquisition time and workload. To improve reconstruction efficiency and speed, Boominathan et al.[287] developed an autoencoder-based neural network architecture in 2018. This architecture adapts the training phase recovery at low-overlap levels, overcoming complete failure. The method achieves high-quality and fast image reconstruction under four low-overlap conditions: 0%, 15%, 40%, and 65%. The training process and results are shown in Fig. 58. In 2020, Strohl et al.[288] addressed the transformation of estimating illumination parameters into an object detection problem and solved this by employing fast CNNs. By training faster region-proposal CNNs (FRCNN), they were able to reduce errors by up to three times. To reduce the need for a large number of low-resolution images required for reconstruction, Bouchama et al.[289] proposed an algorithmic approach in 2023 that combines a physics-informed optimization DNN with statistical reconstruction learning. This method requires fewer low-resolution images to achieve super-resolution reconstruction.
![Reconstruction methods and results under different overlapping rates[287]. (a) Image reconstruction process with low overlap rate. (b) Image reconstruction process with high overlap rate. (c) Phase recovery results of different methods with low overlap rate; from top to bottom are alternate projection (AP) phase recovery algorithm, PtychNet, cGAN-FP, and truth value. (d) Phase recovery results of different methods with high overlap rate; from top to bottom are AP phase recovery algorithm, PtychNet, cGAN-FP, and truth value.](/Images/icon/loading.gif)
Figure 58.Reconstruction methods and results under different overlapping rates[287]. (a) Image reconstruction process with low overlap rate. (b) Image reconstruction process with high overlap rate. (c) Phase recovery results of different methods with low overlap rate; from top to bottom are alternate projection (AP) phase recovery algorithm, PtychNet, cGAN-FP, and truth value. (d) Phase recovery results of different methods with high overlap rate; from top to bottom are AP phase recovery algorithm, PtychNet, cGAN-FP, and truth value.
To achieve real-time imaging, Nguyen et al.[31] proposed a framework based on CNNs in 2018. This method requires only a single frame of FPM image data collected at any given time. The network is trained to predict morphological changes in each cell, frame by frame. The method achieves image reconstruction in about 25 s, which is 50 times faster than traditional model-based FPM algorithms. The network architecture and results are shown in Fig. 59. In 2019, Cheng et al.[290] introduced an FPM image reconstruction method combined with DL, which successfully reduced acquisition time by 69 times, achieving a single high-quality image without altering the original spatial bandwidth product. In live cell imaging, traditional methods require a large number of observations to cover the full cell change cycle to achieve dynamic imaging. In 2023, Zhou et al.[291] proposed a method combining FP microscopy with implicit neural representations (PFM-INRs). By integrating physics-based optical imaging models with INRs, this method increases the representation speed of the continuous image stack by 25 times and reduces memory usage by 80 times, making it more suitable for real-time imaging.
![Network architecture and result analysis[31]. (a) Work flow of Fourier lamination dynamic imaging reconstruction based on deep learning. (b) Use the temporal dynamic information reconstructed by the proposed CNN and compare it with the true value. (c) Network architecture of generative adversarial network (cGAN) for FPM dynamic image reconstruction.](/Images/icon/loading.gif)
Figure 59.Network architecture and result analysis[31]. (a) Work flow of Fourier lamination dynamic imaging reconstruction based on deep learning. (b) Use the temporal dynamic information reconstructed by the proposed CNN and compare it with the true value. (c) Network architecture of generative adversarial network (cGAN) for FPM dynamic image reconstruction.
In 2018, Jiang et al.[292] used CNNs to simulate the FP forward imaging model, recovering complex target information during the network training process, which significantly accelerated the image reconstruction process. The network architecture and reconstruction results are shown in Fig. 60. In 2023, Bohra et al.[293] introduced a dynamic FP reconstruction framework based on neural networks. This framework performs implicit spatial prior on the input images and correlates their similarity with temporal changes in the image acquisition sequence. Dynamic FP image reconstruction can be achieved with a small amount of training data, further improving reconstruction efficiency. In 2019, Gupta et al.[294] proposed a method combining FP with GAN, referred to as FP-GAN. By replacing the agent loss function in GAN with a Siamese network, the method optimizes the network architecture to achieve fast and high-quality image reconstruction even at low overlap rates. Addressing the limitations of FP in macro remote detection, Li et al.[295] proposed a fast FP technology based on DL in 2022. This method uses a 3 × 3 array camera to quickly acquire partial spectrum information of objects and utilizes large-aperture imaging results under non-laser irradiation as ground truth to build the network. With this approach, only nine low-resolution images are needed to reconstruct high-quality images. However, when the FP system experiences distortion (such as noise or random update sequences), the reconstruction results may be affected. To address this issue, Chen et al.[296] proposed a new retrieval method in 2023 using CNNs. This model establishes a nonlinear end-to-end mapping between low-intensity input images and their corresponding high-resolution images, enabling quick high-quality image reconstruction with GPUs.
![The network architecture and simulation results[292]. (a) The network architecture. (b1), (b2) High-resolution amplitude and phase images for simulation. (c1)–(c3) The output of the CNN based on (a) and different wave vectors.](/Images/icon/loading.gif)
Figure 60.The network architecture and simulation results[292]. (a) The network architecture. (b1), (b2) High-resolution amplitude and phase images for simulation. (c1)–(c3) The output of the CNN based on (a) and different wave vectors.
In summary, FP and FPM can significantly improve processing efficiency, reduce the time required for image acquisition and processing, and make large-scale data handling more efficient by integrating DL techniques. This combination helps address challenges posed by large datasets, high computational complexity, strict data processing requirements, and high demands on motion sensitivity and optical systems. However, some challenges remain, such as the potential light damage to samples during prolonged scanning and the need for more specialized knowledge in operation and data analysis. Additionally, the high cost of the equipment makes large-scale applications difficult. For ptychographic imaging, future efforts should focus on enhancing both the efficiency and quality of image reconstruction, while mitigating the influence of system component defects and noise through the integration of DL.
4.2.2 Super-resolution microscopic imaging
Super-resolution microscopy technology overcomes the resolution limits of traditional microscopy, achieving resolutions beyond the optical diffraction limit. It includes techniques such as stimulated emission depletion (STED), fluorescence microscopy, and stochastic optical reconstruction microscopy (STORM). These methods employ advanced optical and computational techniques to surpass diffraction limits, enabling detailed observations at the cellular and molecular levels[297].
(1) Fluorescence microscopy
Fluorescence microscopy is a technique that utilizes the fluorescence effect to observe and analyze samples. It works by exciting a fluorescently labeled substance within a sample, which then emits fluorescence. This fluorescence is captured and recorded by the microscopy system to create images. With the recent advancements in DL, fluorescence microscopy has significantly benefited, particularly in the field of super-resolution imaging, where DL techniques enhance image quality and resolution beyond traditional limits.
In 2019, Wang et al.[282] proposed a deep-learning-based super-resolution imaging method for different fluorescence microscope modes. By training a GAN, their framework converts diffraction-limited input images into super-resolution images. This method allows for the transformation of images captured with a low numerical aperture into those with a high numerical aperture, enabling super-resolution imaging. In 2020, Zhao et al.[298] combined DNNs with light fluorescence microscopy to develop imaging technology with a large field of view (FoV) and high resolution. Their method achieved a 15-fold increase in resolution, allowing for the imaging of a wide range of biological tissue samples and reaching an isotropic resolution at the level of a single cell. The Deep-SLAM network architecture and corresponding experimental prediction results are shown in Fig. 61.
![Network architecture and result analysis. (a) Deep-SLAM procedure[298]. (b)–(d) Wide-FOV and isotropic Deep-SLAM imaging. x-z maximum intensity projections (MIPs) of sub-diffraction fluorescence beads imaged by thick SLAM mode (b, 0.02 illumination NA), thin SLAM mode (c, 0.06 illumination NA), and Deep-SLAM mode (d, 0.02 illumination NA + iso-CARE). Scale bars: 50 µm. The magnified views of the PSFs indicate the resolving power by each mode. Scale bars: 20 µm for insets.](/Images/icon/loading.gif)
Figure 61.Network architecture and result analysis. (a) Deep-SLAM procedure[298]. (b)–(d) Wide-FOV and isotropic Deep-SLAM imaging. x-z maximum intensity projections (MIPs) of sub-diffraction fluorescence beads imaged by thick SLAM mode (b, 0.02 illumination NA), thin SLAM mode (c, 0.06 illumination NA), and Deep-SLAM mode (d, 0.02 illumination NA + iso-CARE). Scale bars: 50 µm. The magnified views of the PSFs indicate the resolving power by each mode. Scale bars: 20 µm for insets.
(2) STORM
STORM[29] is a super-resolution imaging technique that overcomes the resolution limits of traditional optical microscopy by utilizing stochastic excitation and imaging of fluorescently labeled single molecules. This technique uses red and green lasers to alternately excite and annihilate fluorescent molecules. The molecules in different positions are randomly excited one at a time, and the fluorescence emitted during each excitation is captured. These individual fluorescence captures are then superimposed to reconstruct a super-resolution image, providing detailed and high-resolution images beyond the diffraction limit.
In dense and overlapping fluorophore emission regions, accurately pinpointing the position of each fluorophore can be challenging. In 2018, Nehme et al.[29] introduced Deep-STORM, a method for obtaining super-resolution images from fluorescent molecules in positioning microscopy. This technique does not rely on prior knowledge and can reconstruct super-resolution images without needing to precisely locate each fluorophore. In 2021, Li et al.[299], inspired by Deep-STORM, combined it with an RNN to develop deep recurrent supervised network-STORM (DRSN-STORM). By incorporating time information from images, additional features are extracted, resulting in more efficient models. Compared to Deep-STORM, this method reduces the runtime by at least 40%, enhancing both the speed and accuracy of the reconstruction, which is crucial for improving the temporal resolution of high-density super-resolution microscopy. In 2020, Yao et al.[300] introduced Deep Residual Learning STORM (DRL-STORM), which integrates residual layers into the network. This allows for the observation of the dynamic structure of mitochondria at a time resolution of 0.5 s, significantly improving temporal resolution. The network architecture of DRL-STORM and a comparison with Deep-STORM experimental results are shown in Fig. 62.
![(a) DR-Storm network architecture. (b) Comparison of experimental STORM data of Deep-STORM and DRL-STORM[300]. (b1) The sum of 500 frames of original images. (b2) Intensity distribution along the dotted white line. (b3), (b4) Images reconstructed using Deep-STORM and DR-Storm, respectively.](/Images/icon/loading.gif)
Figure 62.(a) DR-Storm network architecture. (b) Comparison of experimental STORM data of Deep-STORM and DRL-STORM[300]. (b1) The sum of 500 frames of original images. (b2) Intensity distribution along the dotted white line. (b3), (b4) Images reconstructed using Deep-STORM and DR-Storm, respectively.
(3) STED
STED[301] is a super-resolution fluorescence microscopy technique that leverages the phenomenon of stimulated emission to precisely control the luminous region of fluorescent molecules. This ability allows it to surpass the diffraction limit of classical optical microscopes, achieving higher spatial resolution than traditional optical microscopy. In recent years, with the advancement of science and technology, STED microscopy has gained significant advantages, particularly in the realm of living cell imaging when combined with DL techniques.
The best lateral resolution achievable with STED microscopy is approximately 20 nm. However, when used to observe active cells, its resolution is often reduced due to the effects of phototoxicity. To address this issue, in 2020, Li et al.[302] proposed the super-resolution deep adversarial network (SRDAN). SRDAN is based on the conveying path-based convolutional encoder-decoder (CPCE) architecture and utilizes a Wasserstein GAN (WGAN) framework. By incorporating STED PSF prior knowledge and the cell structure model as training datasets, SRDAN significantly enhances the resolution of STED images from 60 to 30 nm.
![UNet-RCAN architecture and result analysis[304]. (a) UNet-RCAN network. (b) Restoration results of UNet-RCAN, 2D-RCAN, CARE, pix2pix, and deconvolution on noisy 2D-STED images for β-tubulin in U2OS cells in comparison to the ground-truth STED data.](/Images/icon/loading.gif)
Figure 63.UNet-RCAN architecture and result analysis[304]. (a) UNet-RCAN network. (b) Restoration results of UNet-RCAN, 2D-RCAN, CARE, pix2pix, and deconvolution on noisy 2D-STED images for β-tubulin in U2OS cells in comparison to the ground-truth STED data.
To avoid the destruction of cells caused by high-power light sources, Yuan et al.[303] in 2023 actively reduced the light source power by half. They developed fluorescence lifetime imaging based on a GAN (flimGANE). This network is trained to convert low-photon-count fluorescence decay into highly realistic artificial high-photon-count decay, enabling the generation of high-resolution fluorescence images. In order to achieve super-resolution imaging under these circumstances, Ebrahimi et al.[304] proposed a two-step prediction architecture based on a U-Net and a residual channel attention network (RCAN) (UNet-RCAN) in 2023. This approach reduced the pixel residence time in STED by one to two orders of magnitude, thereby minimizing the effects of phototoxicity while enhancing image quality. Figure 63 illustrates the network architecture and its corresponding reconstruction results.
In summary, super-resolution microscopic imaging enables high-resolution observation at the nanoscale by surpassing the optical diffraction limit. However, it faces significant challenges, including high computational requirements, complex sample handling, and expensive equipment costs. The introduction of DL methods has improved imaging resolution and reconstruction efficiency. Nevertheless, the influence of the light source on sample tissue remains a persistent issue, and other challenges, such as the need for large amounts of training data and the high computational costs of models, still need to be addressed. Future research will likely focus on improving the model’s generalization ability, reducing dependence on extensive labeled data, and optimizing computational efficiency.
4.2.3 Holographic image reconstruction
Holography[305] is an optical imaging technology that employs the principles of interference and diffraction to record and reproduce the three-dimensional information of objects. Building on traditional holography, digital holography replaces dry plates with photodetectors to capture holograms and simulates the diffraction process using computer algorithms, thereby reconstructing both the amplitude and phase information of the recorded object[306,307].
With the continuous advancement of optical imaging and computational technology, the application of DL in digital holography—encompassing tasks such as backlight imaging—has been largely realized through CNNs. By mapping input to output and compensating for lost information, CNNs establish a framework specifically suited for digital holography. DL offers significant advantages in handling large-scale datasets, enhancing the generalization ability of models through extensive training. As a result, DL plays a crucial role in holographic imaging, improving both the efficiency and accuracy of image reconstruction.
Complex problems such as autofocus, denoising, and phase unwrapping in holographic image reconstruction can be effectively addressed by incorporating DL techniques. In 2017, Nguyen et al.[308] introduced a novel method that combines supervised DL with CNNs and Zernike polynomial fitting. This method enables automatic background region detection and aberration compensation by calculating the self-conjugated phase. In 2018, Ren et al.[26] developed an autofocus CNN that achieves fast autofocus even when the optical-physical parameters are unknown. To improve computing speed and reduce the number of measurements, Rivenson et al.[25] demonstrated that a trained neural network can generate artifact-free phase and amplitude images using just a single hologram intensity. This method significantly accelerates holographic imaging by rapidly eliminating twin images and spatial artifacts.
Existing holographic image reconstruction methods based on neural networks typically rely on lightweight networks, enabling real-time imaging but limiting the network’s fitting ability. To address this limitation, Yu et al.[309] proposed a non-end-to-end neural network in 2023 that performs real-time imaging through two stages: phase prediction and holographic image coding. This method customizes specific subnetworks for each process, thereby enhancing the network’s fitting ability. In 2022, Liao et al.[310] introduced a denoising convolutional neural network (DCNN) that simplifies the inverse problem of scattering imaging into a denoising issue for degenerated holograms. This approach enables imaging through various scattering media in an off-axis digital holographic framework.
To address the phase unwrapping problem, Wang et al.[311] proposed a deep-learning-based phase unwrapping (DLPU) method in 2019, specifically tailored for phase-type objects. Using a neural network to optimize the phase unwrapping process, they successfully unwrapped the phase of living cells and dynamic objects. This approach demonstrated that complex nonlinear phase unwrapping tasks can be completed directly by a single DNN in one step. The network architecture and corresponding results are shown in Fig. 64.
![Network training process and phase unwrapping results[311]. (a) Training and testing of the network. (b) CNN results for samples obtained from the test image set. Wrapped, real, and unwrapped phase images of the CNN output. (c) Compare the phase heights at both ends of the centerline represented by the real and unwound phases of the CNN output.](/Images/icon/loading.gif)
Figure 64.Network training process and phase unwrapping results[311]. (a) Training and testing of the network. (b) CNN results for samples obtained from the test image set. Wrapped, real, and unwrapped phase images of the CNN output. (c) Compare the phase heights at both ends of the centerline represented by the real and unwound phases of the CNN output.
In the process of holographic image restoration and reconstruction, multi-step reconstruction combined with DL can significantly improve the quality of holographic image reconstruction. It plays a crucial role in phase unwrapping, autofocus, and speeding up the reconstruction process. However, it still faces challenges in removing zero-order and twin images. Digital holography typically requires the removal of these images to enhance clarity. To address this issue, in 2018, Wang et al.[27] proposed an end-to-end-learning-based method for in-line holography reconstruction, called eHoloNet. This network can map holograms end-to-end directly from a single-lens digital hologram to the target wavefront, effectively reconstructing the wavefront of an object while removing the effects of zero-order and twin images. In 2019, Ren et al.[77] introduced another end-to-end holography reconstruction network (HRNet), which can reconstruct noise-free images without any prior knowledge, while simultaneously removing the interference caused by zero-order and twin-images. Unlike traditional phase-retrieval algorithms, which require iterative reconstructions involving multiple autofocus measurements, this approach simplifies the process by leveraging DL. In 2020, Li et al.[312] proposed a new DL method that utilizes the characteristics of autoencoders to reconstruct random single hologram images from image samples without the need for a large number of real sample data pairs for training.
To enhance reconstruction efficiency, Wu et al.[74] proposed a U-Net-based network in 2018, which can simultaneously perform autofocus and phase retrieval. This network enables rapid phase recovery and image reconstruction, improving the overall speed and efficiency of the process. In 2019, Wang et al.[75] introduced a digital holographic image reconstruction method based on a one-to-two DL framework (Y-Net). This compact network, which reduces the number of parameters, is capable of simultaneously reconstructing amplitude and phase information from a single digital hologram, offering a more efficient solution for holographic imaging. In 2021, Wu et al.[313] proposed a holographic encoder based on an autoencoder. This method integrates the confirmed optical propagation model into the network during training, allowing for the rapid reconstruction of holographic images through simulated encoding and decoding, further improving the efficiency and speed of holographic image processing.
In 2021, Di et al.[314] proposed PhaseNet, an optimized structured CNN that enables a deep-learning-based holographic microscope for quantitative phase imaging (QPI). The proposed method significantly enhanced the phase reconstruction process by leveraging DL to extract phase information directly from holographic data. The experimental device setup, network architecture, and corresponding reconstruction results are illustrated in Fig. 65.
![Experimental setup, PhaseNet architecture, and results[314]. (a) The true values. (b) Reconstruction results of PhaseNet. (c) Three-dimensional images of the reconstructed results. (d) Error mapping between the true value and PhaseNet reconstruction results. (e) Deep-learning-based holographic microscope. (f) Detailed schematic of the PhaseNet architecture.](/Images/icon/loading.gif)
Figure 65.Experimental setup, PhaseNet architecture, and results[314]. (a) The true values. (b) Reconstruction results of PhaseNet. (c) Three-dimensional images of the reconstructed results. (d) Error mapping between the true value and PhaseNet reconstruction results. (e) Deep-learning-based holographic microscope. (f) Detailed schematic of the PhaseNet architecture.
In summary, deep-learning-based holographic image reconstruction significantly enhances image quality and processing efficiency. It effectively reduces noise, resulting in clearer images, and enables models to recover image details by learning complex patterns and features. The processing speed is also improved through optimized workflows. However, challenges remain, including the need for large amounts of annotated data for training, varying reconstruction performance across different hologram images, and limited generalization ability of the models. Moving forward, efforts should focus on improving the model’s robustness to noise, reducing reliance on extensive labeled datasets, and further enhancing the quality of image reconstruction.
4.2.4 Quantitative phase imaging based on transport of intensity
With the advancement of computational microscopy techniques, such as the transport of intensity equation (TIE)[315] proposed by Teague, QPI technology has emerged as a means to measure the quantitative phase distribution of unlabeled biological samples without the need for labeling. While these methods strike a balance between resolution and FoV, they grapple with issues of data redundancy and computational efficiency. The recent integration of deep learning technology into QPI has heralded new breakthroughs. In 2020, Wang et al.[316] achieved TIE phase recovery from a single-intensity image through deep learning methods. Concurrently, Wang et al.[317] introduced a physics-enhanced deep neural network (PhysenNet), integrating a physical model to mitigate the reliance on large labeled datasets in traditional computational imaging. This paradigm shift enables direct recovery of phase information from a single measurement, obviating the need for training. These studies underscore the potency of deep learning in extracting phase information from limited data, thereby reducing the necessity for multiple images. In 2024, Jin et al.[318] proposed a neural-field-assisted transport of intensity phase microscopy (NFTPM) method, which introduces adjustable defocus parameters into the neural field, enabling stable QPI from a single defocused intensity image under unknown defocus distances. As depicted in Fig. 66(a), NFTPM leverages a lightweight multilayer perceptron (MLP) to represent phase distributions as neural fields, constrained by the physical prior of forward image formation, as shown in Fig. 66(b), eliminating the requirement for training. This gradient-based iterative algorithm optimizes the MLP using backpropagation, enabling simultaneous adjustment of the defocus distance within the computational graph. NFTPM demonstrates accuracy in phase recovery for non-weak phase objects and adapts to various partial coherence illuminations, offering a robust solution for quantitative phase microscopy in dynamic optical environments. Experiments with HeLa cells, as shown in Figs. 66(d1) and 66(d2), validated the effectiveness of NFTPM, showcasing its potential for advancing live cell biology applications. In summary, the integration of deep learning technology in QPI has not only enhanced imaging speed and data efficiency but also bolstered the precision of phase retrieval, rendering high-resolution live cell imaging a tangible reality. These advances have provided powerful new tools for various fields, including biomedical research, drug discovery, and the identification of disease mechanisms.
![(a) Schematic diagram of NFTPM[318]. (b) The physics prior (forward image formation model) of NFTPM. (c1), (c2) Phases of HeLa cells retrieved by AI-TIE. (d1), (d2) Phases of HeLa cells retrieved by NFTPM.](/Images/icon/loading.gif)
Figure 66.(a) Schematic diagram of NFTPM[318]. (b) The physics prior (forward image formation model) of NFTPM. (c1), (c2) Phases of HeLa cells retrieved by AI-TIE. (d1), (d2) Phases of HeLa cells retrieved by NFTPM.
4.3 Imaging information acquisition upgrade
4.3.1 Three-dimensional shape reconstruction
Three-dimensional (3D) target information recovery is a critical aspect of modern science and technology, impacting various fields such as industrial design, medical diagnosis, and intelligent robotics[319]. Accurately reconstructing the geometry and surface details of objects is essential in these domains. Currently, 3D imaging techniques are categorized based on how they acquire target information. These methods include the time-of-flight (ToF) technique, which uses advanced distance sensing to generate depth maps; structured light and stereo vision systems; and polarization imaging, which characterizes the surface of an object by analyzing target normal information. Recently, DL methods have shown significant success in various 2D computer vision tasks, such as target segmentation[320,321], target tracking[322,323], and target classification[324–326]. The rapid development of these techniques has led to new insights into conventional 3D reconstruction methods, resulting in promising advancements in deep 3D reconstruction techniques.
(1) Structured light method
The structured-light-based 3D reconstruction technique relies on parallax calculations derived from the distortion of an infrared pattern projected onto the object. This enables the estimation of depth information and the acquisition of raw point cloud data for the object to be reconstructed. High-precision 3D reconstruction is then achieved through various processing techniques, such as shape representation[327]. Although this method offers high imaging accuracy, it faces significant challenges in terms of stability, real-time performance, and reliability. Furthermore, the accuracy of the coded stripes decreases with increasing imaging distance, which significantly affects the precision of 3D imaging results[328]. DL methods, leveraging the robust feature extraction capabilities of CNNs, can replace the traditional complex computational processes and streamline the integration of measurement steps. This approach enhances both the precision and speed of measurements, offering a promising solution for structured-light-based 3D reconstruction[329].
Guo et al.[330] proposed a multiscale convolutional parallel method for line-structured light center extraction based on end-to-end DL. This method first uses a network for target detection, then extracts the region of interest (ROI) to identify the line-structured light. In fringe projection profilometry (FPP), to address the contradiction between the measurement efficiency and accuracy of traditional phase retrieval methods, Feng et al.[331] first proposed the use of convolutional neural networks (CNNs) for fringe analysis on a single fringe pattern, as shown in Fig. 67(a). Based on the properly trained framework, CNN outperforms traditional Fourier-transform-based methods in terms of high accuracy and edge protection, as shown in Fig. 67(b). Then, scholars subsequently focused on single-frame fringe projection profilometry (FPP). In 2020, Qian et al.[332] incorporated geometric constraints into the neural network to achieve high-quality phase retrieval on a single-frame projection. In the same year, Qian et al.[333] proposed a single-shot absolute 3D shape measurement method for deep-learning-based color FPP, which can predict high-resolution, motion-artifact-free, crosstalk-free absolute phase directly from a single color fringe image. In 2022, Li et al.[334] demonstrate that the deep neural network can be trained to recover absolute phase directly from a unique fringe image involving spatially multiplexed fringe patterns of different frequencies. The extracted phase does not suffer from spectral aliasing, which is difficult to avoid with conventional spatial multiplexing methods.
![Flowchart of deep-learning-based phase retrieval method and the 3D reconstruction results of different approaches[331]. (a) The principle of deep-learning-based phase retrieval method: first, the background map A is predicted from the single-frame stripe image I by CNN1; then, the mapping of the stripe pattern I and the predicted background map A to the numerator term M and denominator term D of the inverse tangent function is realized by CNN2; finally, a high-precision wrapped phase map can be obtained by the arctangent function. (b) Comparison of the 3D reconstructions of different fringe analysis approaches (FT, WFT, the deep-learning-based method, and 12-step phase-shifting profilometry).](/Images/icon/loading.gif)
Figure 67.Flowchart of deep-learning-based phase retrieval method and the 3D reconstruction results of different approaches[331]. (a) The principle of deep-learning-based phase retrieval method: first, the background map A is predicted from the single-frame stripe image I by CNN1; then, the mapping of the stripe pattern I and the predicted background map A to the numerator term M and denominator term D of the inverse tangent function is realized by CNN2; finally, a high-precision wrapped phase map can be obtained by the arctangent function. (b) Comparison of the 3D reconstructions of different fringe analysis approaches (FT, WFT, the deep-learning-based method, and 12-step phase-shifting profilometry).
Van der Jeught et al.[335] demonstrated that DL could demodulate the depth information from a single stripe, offering the ability to fuse noise reduction, background masking, phase unwrapping, and other optional or mandatory intermediate steps into the final depth computation, making the process a one-step operation. Nguyen et al.[336] showed that the U-Net model outperforms FCNs and autoencoder networks (AENs). A significant amount of stripe data was gathered in a realistic setting, leading to the goal of directly obtaining corresponding 3D height maps from the stripes. The team later introduced a global guided network path with multiscale feature fusion in U-Net[337], achieving better performance, though the measurement accuracy was still inferior to that of traditional 3D imaging methods. To address this issue, Nguyen et al.[338] proposed a method that converts multiple grayscale images with stripe patterns into 3D depth maps, improving reconstruction accuracy by fusing multiple feature maps. Meanwhile, Nguyen et al.[339] explored the effect of structured light patterns on 2D to 3D DL networks. Their findings showed that all six representative structured light patterns could be used and trained to reconstruct 3D shapes with reasonable accuracy, though scatter and low-frequency stripe patterns resulted in reduced precision. Machineni et al.[340] introduced a DL scheme that removes the need for frequency-domain filtering or phase unwrapping. Their method performed multiscale similarity assessments to obtain depth-informed contours from deformed stripe maps with the aid of a CNN. Wang et al.[341] proposed a dual-path hybrid network based on U-Net, which removed the deepest convolutional layer to reduce the total learning parameters of the network. They also added a Swin Transformer path on the decoder to enhance global perception. Jia et al.[342] introduced a depth measurement method based on CNNs that uses a feature pyramid in the encoder to extract multiscale fusion features, along with parallel classification and regression branches at the decoder’s end to predict depth from coarse to fine. The network structures of these different methods can be compared as in Fig. 68.
![(a) Network architecture and result of global guided network path with multiscale feature fusion[338]. (b) Network architecture and result of depth measurement based on convolutional neural networks[342].](/Images/icon/loading.gif)
Figure 68.(a) Network architecture and result of global guided network path with multiscale feature fusion[338]. (b) Network architecture and result of depth measurement based on convolutional neural networks[342].
![Flowchart of DLMFPP: The projector sequentially projects the stripe pattern onto the dynamic scene so that the corresponding modulated stripe images encode the scene at different time[345]. The camera then captures the multiplexed images with longer exposure time and obtains the spatial spectrum by Fourier transform. A synthesized scene consisting of the letters “MULTIPLEX” is used to illustrate the principle.](/Images/icon/loading.gif)
Figure 69.Flowchart of DLMFPP: The projector sequentially projects the stripe pattern onto the dynamic scene so that the corresponding modulated stripe images encode the scene at different time[345]. The camera then captures the multiplexed images with longer exposure time and obtains the spatial spectrum by Fourier transform. A synthesized scene consisting of the letters “MULTIPLEX” is used to illustrate the principle.
Deep neural networks often lack the ability to guarantee provably correct solutions, and their prediction errors are challenging to detect and evaluate in the absence of ground truth data. Feng et al.[343] demonstrate that a Bayesian convolutional neural network (BNN) can be trained to not only retrieve the phase from a single fringe pattern but also produce uncertainty maps depicting the pixel-wise confidence measure of the neural network on its prediction. By integrating a lightweight deep neural network with a learned-enhanced Fourier transform profilometry module, Yin et al.[344] proposed a physics-informed deep learning method (PI-FPA), which demonstrates that challenging issues in optical metrology can be potentially overcome through the synergy of physics-priors-based traditional tools and data-driven learning approaches, opening new avenues to achieve fast and accurate single-shot 3D imaging.
To break through the limitations of the camera’s hardware frame rate, Chen et al.[345] demonstrate a new 3D imaging method, termed deep-learning-enabled multiplexed FPP (DLMFPP), which allows to achieve high-resolution and high-speed 3D imaging at a near-one-order of magnitude higher 3D frame rate with conventional low-speed cameras, as shown in Fig. 69. DLMFPP successfully measures rotating fan blades and bullets fired from a toy gun at 1000 Hz while using cameras operating at around 100 Hz.
(2) Time-of-flight method
The ToF method, based on the principle of time or phase differences in light reflection, facilitates the measurement of depth information for objects within a scene. In 3D imaging, the ToF technique is employed to create a 3D model of an object or scene by capturing distance information from various points. One of the primary applications of the ToF method is in laser radar (LiDAR), which is widely used in fields such as autonomous driving, topography, and environmental monitoring. While ToF-based LiDAR offers high accuracy and resolution for 3D imaging, it is not without its challenges. These include high costs, significant data processing, and storage requirements, sensitivity to environmental conditions (such as weather or lighting), and limited recognition capabilities. The introduction of DL techniques has led to advancements in the processing of LiDAR point cloud data. These advancements have facilitated the classification of point cloud representations into three main categories: projected point clouds, voxel-based point clouds, and raw point clouds, each of which serves different applications and challenges in 3D imaging and analysis.
The projection method involves projecting a three-dimensional (3D) point cloud onto a two-dimensional (2D) image, where it is processed using an existing 2D CNN to identify class labels. After the classification, the 2D image is then projected back onto the corresponding 3D point cloud, with each point being labeled accordingly. This approach enables the use of powerful 2D image processing techniques to handle 3D point cloud data, simplifying the problem and improving computational efficiency. Several projection techniques are commonly used, including spherical projection, bird’s eye view (BEV) projection, and hybrid methods based on projection fusion, as shown in Fig. 70. BEV projection, introduced in 2018 by Beltrán et al.[346], is one of the most widely adopted methods. It projects the point cloud data onto a 2D plane from a bird’s eye perspective, providing a clear view of the scene’s layout. Following this, models like BirdNet and BirdNet+[347] were developed, utilizing height, intensity, and density information to encode the BEV projection for more accurate spatial representations. In an effort to further improve the performance of BEV projection, Barrera et al.[348] modified BirdNet+ and proposed a two-stage model that incorporates the faster R-CNN architecture. This model not only projects the LiDAR point cloud data into the BEV but also applies a density coding method to normalize the projections, allowing for more refined classification and analysis of point cloud data.
![(a) Results obtained using different lidar devices in 360°[346]. (b) Previous framework versus proposed approach for 3D detection[347]. (c) Result of BirdNet+[348].](/Images/icon/loading.gif)
Figure 70.(a) Results obtained using different lidar devices in 360°[346]. (b) Previous framework versus proposed approach for 3D detection[347]. (c) Result of BirdNet+[348].
Voxel-based methods decompose a 3D point cloud into regular 3D cubes, called voxels or volume units, to facilitate processing using CNNs. These methods break down complex 3D scenes into smaller, more manageable components, and apply 3D CNNs to each voxel, which allows for effective feature extraction and pattern recognition across the 3D space. Qi et al.[349] introduced PointNet, a pioneering approach that learns the shapes of points in the 3D point cloud, enabling the network to simplify, classify, and even reconstruct the point cloud back to its original shape. While PointNet demonstrated strong performance for certain applications, it is primarily effective on simple objects at low resolution. To address the limitations of low resolution, Tatarchenko et al.[350] proposed an octree-based network for 3D model reconstruction using voxel representations. This method divides the 3D space into an octree structure, which improves efficiency by focusing on denser regions of interest and enhancing model detail as the network layers progress. As the layers become deeper, the model representations improve with greater voxel-level detail. For large scene reconstructions, Yao et al.[351] introduced MVSNet, as shown in Fig. 71(a), a multi-view stereo network designed for reconstructing 3D models from large-scale scenes. By taking multiple RGB images from various perspectives, the network learns relationships between the images, allowing for robust 3D scene reconstruction. However, this method is computationally expensive and demands significant RAM, as it processes large quantities of data to build comprehensive 3D models. To handle single-object 3D reconstruction, Luo et al.[352] proposed a model that uses sparse RGB-D images (which combine color and depth information) to reconstruct a 3D object, as shown in Fig. 71(b). A voxel filling operation is employed to close gaps or holes in the surface, thus improving the completeness and accuracy of the 3D model. Additionally, the work involves optimizing the texture mapping of the 3D model.
![(a) The network design of MVSNet[351]. (b) The pipeline of reconstructing a 3D model using sparse RGB-D images.](/Images/icon/loading.gif)
Figure 71.(a) The network design of MVSNet[351]. (b) The pipeline of reconstructing a 3D model using sparse RGB-D images.
(3) Stereoscopic vision
Binocular vision, also known as stereoscopic vision, is a method of perceiving the distance and depth of an object based on the parallax difference between the two eyes. This technique mimics the way the human eye perceives the world, providing a pronounced sense of depth and realism. However, it requires sophisticated image processing and computation. The reconstruction of binocular vision can be broadly divided into five stages: image acquisition, camera calibration, image correction, stereo matching, and 3D reconstruction.
Stereo matching is a technique used to derive depth information about an object by calculating the positional difference between corresponding matching pixel points in two images. The accuracy of stereo-matching directly affects the accuracy of 3D reconstruction. Optimizing the stereo matching algorithm can significantly enhance the quality of the entire 3D reconstruction process. Xu et al.[353] proposed a stereo-matching network called ACV-Net, which employs a novel cost-space construction method to generate attentional weights, as shown in Fig. 72(a). This method suppresses redundant information and enhances the matching-related information within the connectome. Weida et al.[354] introduced a novel approach to parallax map reconstruction, as shown in Fig. 72(b), transforming it into a continuous global energy optimization model. By applying PatchMatch and tree dynamic programming strategies, they optimized the energy model at the super-pixel level. This approach has the potential to fundamentally improve the inefficiency and robustness of traditional stereo matching algorithms in parallax map reconstruction, significantly enhancing efficiency and providing notable improvements in 3D reconstruction. Shao et al.[355] proposed DiffuStereo, a novel system for high-quality 3D human reconstruction using only sparse cameras. This system introduces a diffusion-based stereo module into an iterative stereo matching network, facilitating stereo matching and depth estimation, and generating high-precision depth maps. These maps are then converted into high-quality 3D human models through an efficient multi-view fusion strategy. In comparison, Zheng et al.[356] initially proposed the DiffuVolume algorithm, which treats the diffusion model as a volumetric filter and embeds it into a task-specific module.
![(a) The structure of ACV-Net[353]. (b) The structure of proposed Sparse PatchMatch[354].](/Images/icon/loading.gif)
Figure 72.(a) The structure of ACV-Net[353]. (b) The structure of proposed Sparse PatchMatch[354].
4.3.2 Polarization imaging
Polarization, a fundamental physical property of light, can be leveraged to extract detailed information and enhance image quality by utilizing the intrinsic physical properties of the target. The advancement of computer technology has enabled the development of DL methods capable of accurately extracting and analyzing complex polarization information, facilitating precise identification and classification of target objects. These methods are poised to enable high-resolution imaging and target detection in complex environments that are otherwise difficult to observe, thereby significantly advancing the field of polarization imaging. Furthermore, the existing data-driven polarization imaging methods can be categorized into four main groups, as shown in Fig. 73. These categories include: polarization descattering, reflection removal, and enhancement based on the recovery and utilization of polarized light-field information; three-dimensional shape reconstruction, target detection, and semantic segmentation through target polarization feature characterization; and polarization biophotonics and imaging for biomedical applications, including pathology diagnosis.
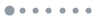
Figure 73.Applications of data-driven polarimetric imaging.
(1) Target polarization characterization
Polarized 3D imaging. Since the 1970s, researchers in the field have been investigating methodologies for three-dimensional shape recovery using polarization information[357–361]. This led to the development of various polarization-based 3D imaging techniques, collectively known as the shape-from-polarization (SFP) method. The SFP method utilizes the polarization information of reflected light to estimate the shape of objects, effectively reducing the loss of surface texture and mitigating the challenges posed by poor lighting conditions[362]. The SFP method offers several advantages, including high accuracy, a long working distance, non-contact operation, and robust anti-interference capabilities. Even in low-illumination environments, the polarization characteristics of the reflected light can still be captured, ensuring reliable shape estimation.
However, accurate target information can be compromised by surface normal ambiguity, necessitating the use of additional constraints or auxiliary information to resolve this issue[363,364]. Koshikawa[357] addressed this challenge by computing the surface normal of the target through the analysis of the change in the polarization state of circularly polarized light reflected from the surface of an insulator. Smith[365] proposed a method for linear depth estimation based on sparse linear equations to resolve the problem of orientation blur, enabling the direct estimation of surface depth from a single polarized image. Mahmoud[366] introduced a polarized 3D imaging technique that incorporates a shadow recovery method to eliminate orientation blur and determine the surface normal of an object from a spectral image. Kadambi et al.[367,368] developed a methodology that combines depth maps acquired with the Kinect device with polarized 3D imaging, achieving high-precision and robust polarized 3D imaging under both indoor and outdoor lighting conditions.
Traditional polarization 3D imaging methods often face challenges such as high computational complexity, limited accuracy, difficulties in parameter adjustment, and sensitivity to noise and interference, especially when addressing the multi-valued surface normal problem. The integration of DL techniques can effectively mitigate these issues by enabling automated and adaptive parameter tuning, thereby enhancing the robustness of the model against noise and interference. This ultimately improves the performance and applicability of polarization 3D imaging. Ba et al.[369] proposed the use of DL networks to address challenges in the computation and reconstruction process of polarization 3D imaging. Their trained model achieved robust surface normal estimation results under varying indoor and outdoor lighting conditions. Kondo et al.[370] introduced a new pBRDF model to characterize the polarization properties of various materials and developed a renderer to generate a large number of realistic polarization images for training the polarization 3D imaging model. Deschainte et al.[371] proposed a method that combines polarization information with DL to efficiently obtain high-quality shape estimates and spatially varying reflectance of 3D objects under flashes of light.
Most existing SFP methods are primarily focused on estimating the normal of a single object, rather than handling the complexities of estimating normals in an FoV with multiple objects. To address this limitation, Lei et al.[230] proposed a novel data-driven polarized 3D imaging method that incorporates physical priors for scene-level normal estimation from polarized images. Similarly, Hur et al.[372] employed a single CNN to derive the 3D structure of a scene from two temporally contiguous images. Han et al.[373] introduced a learning-based passive 3D face polarization reconstruction method, as shown in Fig. 74(a). This method derives the blurred normal of each microfacet of the face at the pixel level using diffuse polarization, with a CNN-based 3D deformable model (3DMM) generating an initial depth map of the face from a directly captured polarization image. This depth map is then used to correct the blurred polarization normals and reconstruct an accurate 3D face using the Frankot-Chellappa 3D surface recovery function. In 2023, Wu et al.[374] designed a network that not only separates specular and diffuse reflection components but also optimizes the polarization input using Stokes vector-based parameters. This method effectively reduces the impact of background noise, facilitates the extraction of more relevant polarization features from the target, and provides more accurate information for surface normal recovery. In the same year, Li et al.[375] proposed a method for enhanced multi-target 3D reconstruction using DNNs. This approach accurately provided spatial information by modeling the relationship between blur, distance, and sharpness of the target, while minimizing inaccuracies caused by continuous models. Huang et al.[376] pioneered the use of stereo polarization information and DL by designing a mask prediction module, as shown in Fig. 74(b). This module reduces the impact of polarization information blurring based on the consistency of normal and parallax, achieving high-precision imaging.
![(a) Passive 3D polarization face reconstruction method[373]. (b) Overview of Huang’s approach[376].](/Images/icon/loading.gif)
Figure 74.(a) Passive 3D polarization face reconstruction method[373]. (b) Overview of Huang’s approach[376].
Target detection. Polarization information can be utilized to accurately characterize a range of critical physical properties, such as geometry, material characteristics, and surface roughness. This is particularly valuable in scenarios where the object is located in a complex low-light environment or is camouflaged, as polarization data can effectively overcome the limitations of these conditions[377–382]. It is common for the polarization properties of targets and backgrounds to differ, and polarization imaging serves as an effective method for identifying these differences[383,384]. As a result, it plays a significant role in target detection[385,387]. In target classification or detection tasks, data-driven methods based on polarization provide a robust feature extraction capability. These methods can automatically learn and extract intricate polarization features from images, as well as effectively fuse multimodal data, thereby enhancing overall detection performance.
Polarization technology plays a crucial role in enhancing the safety and reliability of unmanned systems. It achieves this by improving sensing capabilities, enhancing detection performance in adverse weather conditions, enabling material identification and classification, increasing environmental adaptability, and optimizing sensor fusion. Fan et al.[388] were the first to propose using polarization-complementary intensity information to improve vehicle detection accuracy. During the feature selection process, the most informative polarization features are chosen, and a fusion rule based on the polarization model is applied for final detection. Pang et al.[389] explored the advantages of polarization parameters in distinguishing targets and leveraged DNNs to extract features for detecting road scene content under challenging conditions. This approach enhanced detection performance in foggy, rainy, and low-light conditions by approximately 27%. Tan et al.[390] proposed a polarization image fusion method using grouped convolutional attention networks (GCAnets) to improve the detection of cars and pedestrians in foggy road scenes, significantly boosting image recognition accuracy.
It is evident that, in addition to the unmanned systems field, target detection techniques based on polarization and DL have a wide range of applications in other areas. For instance, Tian et al.[391] proposed a face anti-spoofing method for real-world scenarios that combines CNNs and SVMs to extract distinctive polarization features from faces for classification. Usmani et al.[392] introduced a unified approach for detecting and classifying polarized targets in degraded environments using 3D polarized integral imaging data. The deep-neural-network-based 3D polarization images were shown to be effective in detecting and classifying polarized targets under various conditions, including shimmering and occlusion. Huang et al.[393] developed a hybrid computational imaging technique based on holography and polarization to rapidly and accurately identify microplastic contamination in turbid water. The polarization characteristics of the objects were shown to serve as a novel discriminative feature for identifying microplastic materials. Wang et al.[394] proposed a target detection and classification algorithm, as shown in Fig. 75, based on the enhanced YOLOv5 for identifying and classifying foreign fibers. This method was demonstrated to be applicable in practical production line vision systems for detecting foreign fibers in cotton.
![Network model of cotton foreign fiber detection[394].](/Images/icon/loading.gif)
Figure 75.Network model of cotton foreign fiber detection[394].
Semantic segmentation. Image segmentation is a core operation in computer vision, aiming to partition an image into distinct regions that share similar characteristics. This process is essential for more advanced image analysis and interpretation tasks. The integration of polarization data with DL techniques allows for a broader range of feature extraction, reduces noise interference, and enhances detail recognition capabilities. As a result, this integration significantly improves the accuracy and robustness of semantic segmentation.
Yang et al.[395] proposed that the prediction of polarization information from monocular RGB images could enhance RGB-based pixel-level semantic segmentation, particularly benefiting real-world wearable assisted navigation systems. Similarly, Zhang et al.[396] and Blanchon et al.[397] applied various architectural approaches to improve the reconstruction of outdoor environments, laying the groundwork for autonomous navigation and relational reasoning through detailed scene analysis. The polarization texture of transparent objects offers additional distinctive information about the target, distinguishing it from the background and presenting new opportunities for transparent object segmentation. Qiao Y et al.[398] introduced the first polarization-guided glass segmentation propagation solution (PGVS-Net), which effectively and coherently segments glass targets in RGB-P video sequences. By combining spatio-temporal polarization with multi-view color information, this method reduces the visual dependence of single-input intensity variations on glass objects. Liu et al.[399] developed an unsupervised algorithm called NSAE-WFCM, shown in Fig. 76, for distinguishing wet and dry snow using various polarization features derived from snow. This algorithm improves the classification of wet and dry snow based on dual-polarization features, overcoming the limitations of existing methods that rely on quadru-polarized synthetic aperture radar (SAR) data. Additionally, it minimizes manual intervention by leveraging unsupervised clustering. Moreno et al.[400] employed DL techniques to establish a robust monitoring procedure, utilizing the supplementary polarization characteristics of vertically and horizontally received satellite images. Their study developed the first mangrove dataset covering a key region in southeastern Brazil, pioneering the use of polarization DL for mangrove mapping.
![Flowchart of the algorithm NSAE-WFCM[399]. NSAE represents the pixel neighborhood-based SAE with several hidden layers, and WFCM denotes the feature-WFCM under various land cover types.](/Images/icon/loading.gif)
Figure 76.Flowchart of the algorithm NSAE-WFCM[399]. NSAE represents the pixel neighborhood-based SAE with several hidden layers, and WFCM denotes the feature-WFCM under various land cover types.
(2) Polarized light-field information recovery and utilization
Polarization reflection removal. The capture of images through semi-reflective surfaces often results in a decline in visual quality, which can negatively affect the performance of computer vision algorithms. Consequently, the removal of image reflections has become a crucial research area in computer vision. Due to the inherently ill-posed nature of this problem, early approaches involved rotating the polarizer[401,402]. DL methods, however, leverage the polarization properties of reflected light, allowing the network to learn intricate image features by identifying the optimal solution from the image formation process and its physical attributes. These features are embedded within DL networks, significantly enhancing their modeling capabilities and improving the effectiveness of reflection removal[233,403].
Over a decade ago, researchers discovered the significant role that polarization plays in eliminating image reflections. Schechner et al.[402] and Bronstein et al.[404] successfully separated reflected and transmitted images by combining independent component analysis with the principle of polarization. Assuming a non-polarized light source, Kong et al.[405] proposed an optimization method to automatically identify the optimal separation of reflective and transmissive layers. Wieschollek et al.[406] introduced a novel approach by integrating DL with a polarization-based reflection removal technique. Lyu et al.[233] employed pairs of unpolarized and polarized images as physical constraints to distinguish between reflection and transmission images. To overcome the limitation of using a non-polarized light source, Lei et al.[407] developed a two-stage framework for removing reflections from polarized images. Their method infers the transmitted image from estimated reflections, achieving superior performance across various metrics with strong generalization ability. Pang et al.[389] proposed a progressive polarization-based reflection removal network, as shown in Fig. 77, which first generates a preliminary estimate of the transmitted image and then corrects it through final reflection removal, effectively leveraging pre-trained features as prior information.
![Pang’s network architecture and result[389].](/Images/icon/loading.gif)
Figure 77.Pang’s network architecture and result[389].
Polarization information recovery and enhancement. In low-light environments, imaging is often hindered by a low SNR, which adversely affects image quality and poses a challenge for microlight imaging. Polarization information enhancement technology leverages DL to extract more detailed information about the observed object by analyzing and processing the polarization state of light. This technology has made significant strides in areas such as image segmentation, classification, noise reduction, enhancement, multimodal fusion, and automated analysis[248]. These advancements have not only improved the accuracy and efficiency of image processing but have also expanded the technology’s application areas, including intelligent surveillance and augmented reality.
Inspired by residual-dense networks, Hu et al.[248] proposed a one-to-three intensity-polarization image enhancement hybrid network to improve image quality by utilizing both intensity and polarization information. However, this network generates a large number of parameters, which significantly reduces operational efficiency and leads to inaccurate color representation. To address these limitations, Xu et al.[408] employed the ColorPolarNet network for preliminary denoising and color bias correction of four polarization-directed images. They then used the polarization difference network to enhance grayscale details and polarization information. This method exhibited improved processing speed, superior signal fidelity, contrast enhancement, and better color reproduction. Zhang et al.[231] proposed a deep convolutional demosaicing network for polarized images. The network enhances performance by eliminating unnecessary connections and customizing the loss function. This represents a novel approach to demosaicing design. Sargent et al.[409], Sun et al.[410], and Pistellato et al.[411] also proposed data-driven demosaicing techniques to ensure the fidelity of the loss function relevant to AoLP reconstruction. The difference between the two demosaicing networks is shown in Fig. 78. These contributions led to the development of a two-stage, lightweight methodology for real-time reconstruction of intensity and polarization information. Taking advantage of the capabilities of CNNs, Zhang et al.[235] introduced an unsupervised deep network, PFNet, to integrate grayscale and polarization images. The feature extraction module uses two dense blocks to transform the polarization image into a high-dimensional nonlinear feature map, which is then fused using a splicing operator. The fused image is reconstructed through the network’s reconstruction module. Additionally, Duan et al.[412] designed a new polarization image fusion model called GM-PFNet. This method combines the target geometry and material polarization properties with the initial polarization properties of different materials. The approach successfully enhanced polarization image fusion results by extracting more favorable polarization features.
![(a) Network architecture and result of PFNet. (b) Network architecture and result of Sun’s network[410].](/Images/icon/loading.gif)
Figure 78.(a) Network architecture and result of PFNet. (b) Network architecture and result of Sun’s network[410].
(3) Polarized biomedical imaging
Polarized light provides significant advantages in biomedical imaging and pathology diagnosis, mainly due to its ability to extract information that is difficult to obtain with conventional imaging techniques and its potential for noninvasive, high-resolution imaging. Since the morphological diagnostic information of tissue specimens is predominantly encoded in their Mueller matrix[413–417], it is not surprising that biomedical imaging and pathology diagnostic methods based on the polarization features of the Mueller matrix have emerged as promising technologies. This method eliminates the need for markers and invasive manipulation, making it particularly useful for characterizing the anisotropic features of biological tissue microstructures. The rapid development of DL has already shown great promise across a wide array of scientific applications[418–424] and cancer classification[425–433], further enhancing the potential of polarization-based techniques in these fields.
In the field of biological tissue classification, Li et al.[434] first introduced a Mueller-matrix-based imaging system that extracts features from the Mueller matrix and uses CNN for the classification of morphologically similar algae. Their approach achieved a classification accuracy of 97%. Later, they incorporated a distance metric learning method, Siamese, which learns an optimal distance metric for Mueller matrix images of algae in a low-dimensional feature space[435]. The integration of Mueller matrix imaging and Siamese networks has proven to be an effective solution for the automatic classification of morphologically similar algae. Additionally, Bi et al.[436] proposed a classification method that combines impulse feature enhancement with polarized light. This method is particularly effective for classifying datasets of algae, enhancing the robustness and feasibility of the algorithm, and holds significant potential for aquatic environment detection. The methods are structured as shown in Fig. 79.
![(a) Mueller matrix microscope and the schematic of Li’s system[434,435]. (b) Results of classification experiments on algal samples of Ref. [434]. (c) Results of classification experiments on algal samples of Ref. [401]. (d) Network architecture and results of classification experiments on algal samples of Ref. [436].](/Images/icon/loading.gif)
Figure 79.(a) Mueller matrix microscope and the schematic of Li’s system[434,435]. (b) Results of classification experiments on algal samples of Ref. [434]. (c) Results of classification experiments on algal samples of Ref. [401]. (d) Network architecture and results of classification experiments on algal samples of Ref. [436].
Conversely, the Mueller matrix has shown significant promise in characterizing the microstructure of various pathological tissues[437–442]. Deyan et al.[408] combined polarimetry with supervised regression techniques, such as logistic regression, random forests, and SVMs, to develop a polarimetric measurement model capable of distinguishing between healthy and tumorous colon tissue. This approach enhanced the sensitivity and specificity of existing diagnostic methods. In another study, Yang et al.[443] proposed a dual-mode machine learning framework for the quantitative diagnosis of cervical precancerous lesions. This framework, shown in Fig. 80, integrates polarimetric imaging with traditional microscopic imaging to construct a machine learning model based on polarimetric imaging. The model assists in the accurate and quantitative diagnosis of cervical precancerous lesions, offering new possibilities for image-intensive digital pathology.
![Polarization-imaging-based dual-mode machine learning framework for quantitative diagnosis of cervical precancerous lesions[443].](/Images/icon/loading.gif)
Figure 80.Polarization-imaging-based dual-mode machine learning framework for quantitative diagnosis of cervical precancerous lesions[443].
4.3.3 Spectral imaging
Three classical spectral imaging methods include spectroscopic imaging, Fourier transform imaging, and computational spectral imaging (CSI). The direct beam-splitting method divides the light using dispersive elements or filters, which is simple in principle and has a high level of technological maturity. The Fourier transform method uses coherent imaging of fluctuating optics to capture an interferometric image, which is then transformed by a Fourier transform to obtain the spectral image. CSI, on the other hand, typically projects three-dimensional information onto a two-dimensional detector by encoding an aperture and then reconstructs the image and spectral information of the target through a corresponding reconstruction method. Compared to conventional scanning systems, CSI has enabled the development of snapshot optical systems. In CSI, each pixel in a spectral image corresponds to a specific spectrum, and the data files are often large and complex, which can make interpretation challenging. The application of DL in CSI can significantly enhance the reconstruction of spectral images and facilitate advanced tasks such as spectral classification, spectral unmixing, anomaly detection, and spectral reconstruction.
(1) Spectral classification
One of the main applications of spectral imaging is the identification of materials in a scene. Matthew[444] proposed an adaptive spectral classification method using dual-disperse coded aperture snapshot spectroscopic imaging (DD-CASSI) and Bayesian frameworks. This system allows for direct categorization in spatial scenes. In 2019, Hinojosa[445] presented a spectral–spatial image classification method that performs all processing tasks directly on multisensor compressive measurements. Subsequently, in 2022, Carlos[446] proposed a method for classifying underlying spectral images by directly utilizing compressed single-pixel camera (SPC) measurements.
However, all of these methods face challenges in feature selection, as different features can have varying impacts on the results. Additionally, they struggle with handling complex nonlinear relationships and have limited generalization ability. DL approaches offer new solutions to these problems. Xu et al.[447] designed a new CNN architecture to effectively classify 2D spectral data of five samples. Specifically, a dropout layer is added after each convolutional layer of the network to randomly discard some useless neurons to effectively improve feature extraction. In this way, the features mined by the network become stronger, which ultimately reduces the complexity of calculating the network's architectural parameters and thus improves the accuracy of spectral classification. Figure 81(a) shows the optimized network and results. In Ref. [448], an end-to-end method was proposed that optimizes both the encoding aperture and DL model parameters. This method was applied to classify Citrus latifolia, achieving a classification accuracy of 99%. Zhang et al.[449] proposed a three-dimensional coded CNN (3D-CCNN) for hyperspectral classification of compressed measurements, as shown in Fig. 81(b). This approach combines hardware-based coded apertures with software-based 3D-CNNs in a unified framework, which is then co-optimized through end-to-end training to improve the optimization degrees of freedom.
![(a) CNN training network and the maximum testing precision of each sample under four different algorithms[447]. (b) Classification results of the training process (blue arrows) and testing process (red arrows) of 3D-CCNN for spectral data from the University of Pavia[449].](/Images/icon/loading.gif)
Figure 81.(a) CNN training network and the maximum testing precision of each sample under four different algorithms[447]. (b) Classification results of the training process (blue arrows) and testing process (red arrows) of 3D-CCNN for spectral data from the University of Pavia[449].
In addition to spectral information extraction, DL is also widely employed for various spectral image processing tasks[450]. Chen[451] proposed a novel strategy that combines Gabor filtering with CNNs, called Gabor-CNN. Gabor filtering effectively extracts spatial information such as edges and textures, thereby reducing the feature extraction burden on CNNs and alleviating the overfitting problem. Zhu utilized GANs[452], which consist of competing generative and discriminative networks. This method uses generated adversarial samples in combination with real training samples to fine-tune the discriminative CNNs, thereby enhancing the final classification performance. Liu[453] introduced a supervised deep feature extraction method based on the Siamese CNN (S-CNN). S-CNN is trained to improve the separability between different classes, and experimental results on several hyperspectral datasets show that the features extracted by S-CNN significantly improve the performance of hyperspectral image classification.
DL techniques can automatically learn effective features from data without the need for manual design. These techniques are particularly effective in processing and extracting important features from high-dimensional spectral data, benefiting from their strong nonlinear modeling capabilities. As a result, DL methods often achieve higher classification accuracies compared to traditional methods. Additionally, DL models exhibit strong generalization capabilities, enabling them to effectively apply to unseen data after being trained.
(2) Spectral demixing and anomaly detection
Spectral unmixing and anomaly detection are essential techniques in the field of satellite remote sensing, playing a crucial role in remote sensing data processing. Since each pixel in a remote sensing image may contain a mixture of spectra from multiple substances, spectral unmixing aims to decompose the mixed spectrum into individual end-member spectra and their corresponding abundance distributions. On the other hand, spectral anomaly detection involves identifying pixels or targets in remote sensing images that significantly differ from the background. These anomalous pixels often correspond to rare or unexpected targets, such as pollutants, illegal constructions, or unusual vegetation conditions[454].
Over the past three decades, a variety of demixing methods have been proposed and applied to decompose mixed pixels in hyperspectral imaging[455,456]. These methods include the Bilinear–Fan model (BFM), Polynomial Post-Nonlinear Model (PPNM)[457–459], SVM[460–462], and others. Currently, the three primary architectures used for hyperspectral unmixing are deep autoencoders, deep CNNs, and deep generative models[463]. These techniques have significantly advanced the field by improving the accuracy and efficiency of spectral unmixing, enabling more precise analysis of complex remote sensing data.
In 2023, Jin[464] proposed a graph-attention convolutional self-encoder architecture for hyperspectral unmixing, which introduces graph-attention convolution into auto-coding. In this approach, the hyperspectral image is first segmented into hyperpixels to construct the structure shown in Fig. 82. The graph-attention convolution is then applied to extract pixel information from the resulting graph structure. In the spectral dimension, the mapped graph structure information is concatenated with the original hyperspectral image and fed into the autoencoder for further processing. Experimental results demonstrate that this method exhibits strong demixing performance, effectively improving the accuracy and efficiency of hyperspectral unmixing tasks.
![Overall structure of the graph-attention convolution into auto-coding and estimated abundance maps for the Samson dataset[464].](/Images/icon/loading.gif)
Figure 82.Overall structure of the graph-attention convolution into auto-coding and estimated abundance maps for the Samson dataset[464].
Deep CNNs have been applied successfully in visual computing tasks, and numerous studies have leveraged CNNs for hyperspectral unmixing. Arun trained a two-stage end-to-end CNN[465], which enables feature extraction and mapping in separate stages. This approach enhances the efficiency and accuracy of hyperspectral unmixing by progressively processing the data. Later, Hua proposed an improved deep spectral CNN[466], incorporating a polynomial mixture model to refine the abundance estimation. Additionally, parameter optimization using a GAN addressed the end-member variability problem, improving the network’s performance. Bashetti introduced deep generative models for unsupervised spectral separation[467], trained using pure pixel information extracted directly from the data. This model utilizes a nonlinear least-squares method within the low-dimensional potential space of the generative model to optimize abundance estimation, significantly enhancing the quality of the estimated abundance maps.
(3) Spectral reconstruction
Computational spectral reconstruction is a technique used to recover high-dimensional or high-quality spectral image data from low-dimensional or low-quality input images using computer algorithms. This approach reduces hardware costs and time consumption while enhancing system performance and flexibility. The process typically involves two components: the encoder and the decoder. The encoder is the hardware device responsible for encoding or modulating the input light, whereas the decoder is a software algorithm that reconstructs the spectral image data from the encoded image data. Based on the type of encoder, computational spectral reconstruction methods can be divided into three categories: amplitude encoding, phase encoding, and wavelength encoding[6]. Recently, DL techniques have been incorporated into CSI, showcasing the significant potential for achieving fast reconstruction speeds, high reconstruction quality, and substantial reductions in system size. In the following sections, we will review the application of DL in spectral reconstruction, focusing on amplitude-coded reconstruction, phase-coded reconstruction, and wavelength-coded reconstruction.
Amplitude-coded reconstruction. Amplitude coding methods utilize coded apertures and dispersive elements to enable compressed spectral imaging. A well-known example of such a system is the CASSI, where spectral bands are spatially encoded by the coded aperture, then spectrally encoded by dispersive elements, and finally imaged. DL algorithms can be used for fast spectral reconstruction, with end-to-end CNN-based approaches being particularly effective. Research has shown that encoder-decoder architectures with skip-connected CNNs often lead to high-quality reconstruction results[1,468]. Qiao improved these results by integrating U-Net with GAN and a self-attention mechanism[469]. The proposed -net operates in two phases: the first phase (reconstruction phase) generates the 3D hyperspectral cube from the mask and measurements, while the second phase (refinement phase) further refines the hyperspectral image for each channel. As shown in Fig. 83(a), only the -net is capable of recovering the final channel of the hyperspectral image.
![(a) λ-net network structure and bird reconstruction data[469]. (b) Actual data for constructing a generic framework and wheel by connecting each stage sequentially[471].](/Images/icon/loading.gif)
Figure 83.(a) -net network structure and bird reconstruction data[469]. (b) Actual data for constructing a generic framework and wheel by connecting each stage sequentially[471].
However, end-to-end CNNs often lack flexibility, which is a significant limitation in certain applications. To address this, the PnP (plug-and-play) algorithm, which uses pre-trained deep denoising networks as a priori, has been integrated into iterative algorithms. This integration allows the algorithm to be more flexible and adaptable to various applications and systems[470]. Rather than relying on large, monolithic end-to-end CNNs[471], the deep-unfolding-based and PnP algorithms employ a series of smaller CNNs, each referred to as a “stage.” As shown in Fig. 83(b), an optimization-based update rule connects the stages.
End-to-end reconstruction involves sending the measurements directly to a DNN, which then outputs the reconstruction results. In 2019, Fu et al.[472] proposed a convolutional-neural-network-based coded hyperspectral imaging (HSI) reconstruction method. This method learns the depth prior from an external dataset and integrates the internal information of the input-coded image, incorporating spatial–spectral constraints. The network utilizes a CNN-based reconstruction framework with channel attention, employing deep convolutional neural networks to model the spatial–spectral correlation of multilayer nonlinear transform pairs, with dense connectivity and channel attention, as illustrated in Fig. 84(a). In 2018, Wang et al. introduced a CNN that improves reconstruction accuracy by jointly optimizing the coded aperture and reconstruction methods[473]. As shown in Fig. 84(b), their approach connects and optimizes coded aperture design and image reconstruction through a unified DL framework, enhancing the overall reconstruction quality.
![(a) Joint optimization framework for coded aperture and HSI reconstruction[472]. (b) The reconstruction results contain PSNR and spectral angular map SAM[473].](/Images/icon/loading.gif)
Figure 84.(a) Joint optimization framework for coded aperture and HSI reconstruction[472]. (b) The reconstruction results contain PSNR and spectral angular map SAM[473].
An unfolding network is a reconstruction process based on iterative optimization, which is “unfolded” into a neural network structure. Wang et al.[474] proposed an a priori network specifically designed for hyperspectral image reconstruction, adapting it to the iterative reconstruction problem. They derived an iterative optimization formulation based on half quadratic splitting (HQS) and solved the problem by using network layers to learn the optimization process. Deep non-local unrolling (DNU)[475] further simplifies the iterative optimization formulation, making the network more efficient. Literature[476] suggests that the structure of the generative network is sufficient to capture the a priori knowledge required for image reconstruction. More specifically, this approach encompasses all common spectral images to be recovered, eliminating the need for pre-training and offering high generalization capabilities.
Amplitude coding is a method of data compression that works by modulating the spectrum across different bands. The application of various coding methods is greatly enhanced by the flexible modulation of the coding aperture. DL, with its powerful data-driven capabilities, has been extensively applied to spectral reconstruction. Different DL networks offer innovative solutions for amplitude-coded spectral reconstruction.
Phase-coded reconstruction. The core concept of phase coding involves encoding the spectral information of different bands onto specific modes in the imaging plane by incorporating phase modulation elements into the imaging system. This method results in an image that contains spectral information altered through phase modulation. By designing an appropriate decoding algorithm, the spectral information can then be reconstructed from the encoded image. Typically, a diffractive optical element, often referred to as a diffuser, serves as the phase modulation element, enabling the encoding of the spectral data onto the image.
A well-designed PSF is crucial for effective phase encoding, which in turn improves the accuracy of spectral reconstruction. Jeon et al.[477] designed a spectrally varying PSF that rotates with the wavelength, providing an efficient method for encoding spectral information. Hauser introduced a novel DL algorithm, DD-Net, using monochromatic cameras with 1D or 2D diffusers to reconstruct spectral cubes in simulations for high-quality reconstruction. The corresponding architectures, known as DD1-Net and DD2-Net[478], are accompanied by optical system and experimental block diagrams, as shown in Fig. 85. Furthermore, Monakhova et al. developed the spectral DiffuserCam[479], which utilizes a diffuser to extend the point source and a tiling filter array to further enhance spectral encoding.
![(a) Schematic diagram of the optical system[478]. (b) Simplified block diagram of the experiment. (c) Reconstructed images obtained by DD1-Net and DD2-Net.](/Images/icon/loading.gif)
Figure 85.(a) Schematic diagram of the optical system[478]. (b) Simplified block diagram of the experiment. (c) Reconstructed images obtained by DD1-Net and DD2-Net.
The phase-encoded spectral reconstruction technique integrates optical modulation with advanced reconstruction algorithms, offering several advantages, including a simple system structure, high data acquisition efficiency, and improved reconstruction accuracy. Compared to amplitude-encoded spectral imaging, phase encoding significantly enhances optical flux and the SNR. With the ongoing advancements in technologies such as CS and DL, phase-encoded spectral reconstruction is poised to deliver substantial potential and application value across a wide range of fields.
Wavelength-coded reconstruction. Wavelength-encoded spectral imaging utilizes filters to encode spectral features along the wavelength axis. In this method, RGB images encoded with narrowband filters along the red, green, and blue channels are primarily used, as RGB images are commonly employed in display technologies. These spectral images form the basis for rendering scenes on a display. Initially, wavelength-encoded spectral reconstruction was performed pixel by pixel on RGB images. Later, researchers extended the modulation range by using custom-designed broadband filters, allowing for a more comprehensive capture of spectral information.
In 2015, Kelly reconstructed image spectral irradiance from a set of prototypes extracted from a training set[480]. Building on the potential of CNNs to perform complex feature extraction using multiple convolutional operators, Galliani et al.[481] proposed a method for learning spectral super-resolution. This end-to-end approach used CNNs for RGB-to-spectral image reconstruction, achieving impressive spectral reconstruction accuracy on several open spectral datasets. Their work has significantly influenced subsequent CNN-based spectral reconstruction efforts, driving further advancements in the field.
Due to the limited information in RGB images, researchers often enhance the data before reconstruction. A common strategy is to use self-designed broadband filters to extend the modulation range. Nie et al.[482] leveraged the similarity between camera filter arrays and convolutional layer kernels, using camera filters as a hardware implementation layer within the network. By doing so, they optimized the filter response functions and spectral reconstruction mapping, as illustrated in Fig. 86(a). Additionally, the power of DL has enabled researchers to refine spectral imaging parameters, optimizing them for improved spectral reconstruction. Song et al.[483] proposed a joint learning framework for broadband filter design, called a parameter constrained spectral encoder and decoder (PCSED), as shown in Fig. 86(b). This framework combines target spectral response definitions with optical design procedures, linking mathematical optimization with the practical limits imposed by available fabrication techniques, thereby transforming the training process into an optimization problem.
![(a) Example results for end-to-end network architecture and CAVE database[482]. (b) Schematic of the PCSED framework and example results from the CAVE database[483].](/Images/icon/loading.gif)
Figure 86.(a) Example results for end-to-end network architecture and CAVE database[482]. (b) Schematic of the PCSED framework and example results from the CAVE database[483].
Since spectral reconstruction is an image-to-image task, it incorporates many related techniques, such as the U-Net architecture[484], segmentation tasks, and sub-pixel convolutional layers[485]. To extract spectral features from RGB data, DL algorithms either map these features to a streaming space or explore inherent spatial–spectral correlations. Recently, several custom-designed broadband filters for wavelength encoding have been developed, which offer advantages in spectral reconstruction accuracy. However, these methods are sensitive to filter fabrication errors and imaging noise. It is expected that new DL networks and algorithms will emerge in the future to address these challenges and improve reconstruction performance.
5 Image Processing and Enhancement
Vision is the highest level of human perception, and images are the primary source through which humans acquire and exchange information. As such, images play an indispensable role in human perception. Computational processing, as the final link in the computational imaging chain, is characterized by high processing accuracy, good reproducibility, high flexibility, and wide applicability. It plays an increasingly critical role in obtaining high-quality images, enhancing cognitive abilities, and supporting decision-making processes. Computational imaging techniques are widely used in fields such as biomedical engineering, military and public safety, aerospace, remote sensing, and more. With advancements in computer technology and image processing, the scope of computational processing applications continues to expand. Common computational processing techniques include image fusion, image denoising, image restoration, image compression, and image enhancement. The main application areas of computational processing are shown in Fig. 87.
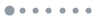
Figure 87.Main application areas of computational processing.
5.1 Image fusion
Due to the theoretical and technical limitations of hardware equipment, images captured by a single sensor or in a single shot often fail to effectively and comprehensively represent the scene being imaged[486]. To improve the quality of the real-world visual signal, it is typically necessary to acquire corresponding images through various fusion methods, such as multi-focus, multi-exposure, multi-spectrum, and multimodal fusion. Image fusion refers to the process of combining information from multiple images to generate a new image that contains all the essential features and details from the original images[487], thereby enhancing the overall quality and informativeness of the image. Generally, based on the differences in target sources and imaging techniques, image fusion can be classified into three categories: digital photography image fusion, multimodal image fusion, and remote sensing image fusion. The schematic diagram of different image fusion scenarios is shown in Fig. 88.
![Schematic diagram of image fusion scene[488].](/Images/icon/loading.gif)
Figure 88.Schematic diagram of image fusion scene[488].
Traditional image fusion algorithms include methods based on multiscale transforms, sparse representations, subspaces, significance, and variational models. However, these methods often overlook the feature differences between different source images, and their feature extraction abilities lack diversity[489]. As a result, the fused images may exhibit shortcomings such as low contrast, blurring, and artifacts[7]. Currently, with the powerful feature extraction, characterization, and reconstruction capabilities of end-to-end DL networks, DL technology has become the mainstream approach in image fusion research.
5.1.1 Digital photography image fusion
In the imaging process, due to the performance limitations of imaging equipment, digital sensors often cannot meet the requirements for handling large exposure differences, and the depth of field of optical lenses limits the range of focus in the image. As a result, actual imaging often fails to capture the complete details of a scene, with objects outside the depth of field appearing blurred. The digital photography image fusion method can address this issue by combining multiple images taken under different settings to generate full-focus images with a high dynamic range. Digital photography image fusion methods can be classified into multi-focus image fusion (MFIF) and multi-exposure image fusion (MEF), depending on the imaging purpose and technology.
(1) Multi-focus image fusion
The limitation of the depth of field of optical lenses makes it impossible to focus on all objects at different focal points clearly during the imaging process. The MFIF method addresses this issue by combining images from different focal areas using a fusion technique, resulting in clear images with a larger depth of field. Generally, MFIF based on DL can be divided into two categories: decision-graph-based fusion methods and global-reconstruction-based fusion methods, depending on the fusion strategy used.
MFIF method based on decision graph. The MFIF method based on decision graphs treats the fusion process of multi-focus images as a binary classification problem[490]. In this approach, a CNN evaluates each pixel of the source images, categorizing them as either focused or unfocused pixels. It then generates a fusion decision graph and selects and combines pixels accordingly to produce a full-focus fused image. In 2017, Liu et al.[491] introduced the first MFIF method based on decision graphs implemented by CNNs. This method used neural networks to solve the pixel binary classification problem, significantly improving computing speed. Later that year, Guo et al.[492] proposed the FuseGAN model, which utilizes a generator to receive multiple source images and create fusion decision graphs. These decision graphs are then matched with real decision graph labels to enhance their accuracy, resulting in focused fusion images with improved quality.
However, the training data generated by Gaussian fuzzy kernels or binary mask methods cannot effectively simulate the defocusing diffusion effect commonly seen in real multi-focus images[493,494]. In 2020, Ma et al.[495] proposed a defocusing model based on the alpha-matte boundary, which generates realistic training data with accurate defocusing diffusion effects. This model achieves clearer fusion results at the focused/defocused boundary (FDB). In 2023, to address issues such as color distortion, boundary artifacts, and high computational costs in existing fusion methods, Zhang et al.[496] proposed a convolutional neural network, MSTMCNN, which combines CNN and transformer architectures. The network employs three different convolutional kernels to extract features from the source images and introduces an initialization module with a down-sampling method, enhancing network performance while accelerating model training. The network model framework and corresponding fusion results are shown in Fig. 89.
![MMF-Net[495]. (a) Model framework. (b) Source image A. (c) Source image B. (d) Fused image.](/Images/icon/loading.gif)
Figure 89.MMF-Net[495]. (a) Model framework. (b) Source image A. (c) Source image B. (d) Fused image.
MFIF method based on direct reconstruction. The MFIF method based on direct reconstruction leverages the learning and fitting capabilities of neural networks to directly reconstruct the full-focus fusion image based on the features of the source images. The network is then continuously optimized by constructing a loss function or conducting adversarial training, forcing the fused image to approach the expected probability distribution at either the pixel level or feature level, ultimately producing the full-focus fusion image. In 2019, Li et al.[497] proposed a direct reconstruction method for MFIF using a U-Net architecture. The source images were first converted into the YCbCr color space, then the brightness components were fused using the U-Net network, while the Cb and Cr components were fused using a weighted strategy. In 2020, Yan et al.[498] introduced an end-to-end CNN-based image fusion framework that eliminates the requirement for ground-truth fused image labels. The method directly utilizes defocused source images while constructing a loss function incorporating structural similarity (SSIM) index measurements to generate all-in-focus output images through end-to-end training. The network structure and fusion results are shown in Fig. 90.
![Multi-focus image fusion network[498]. (a) Model framework. (b) The “Model Girl” source image pair and their fused images obtained with different fusion methods.](/Images/icon/loading.gif)
Figure 90.Multi-focus image fusion network[498]. (a) Model framework. (b) The “Model Girl” source image pair and their fused images obtained with different fusion methods.
However, placing too much emphasis on the fusion accuracy of individual pixels may overlook the integrity of the target and the significance of shallow features, potentially leading to internal errors and boundary artifacts. In 2020, Huang et al.[499] proposed ACGAN, which utilizes an adaptive weight module to assess whether pixels are in focus based on image gradient information. Additionally, the method incorporates a loss function that constrains the fused image to maintain the same distribution as the focused areas in the source image. This approach enables end-to-end generation of the fused image. The effects of some typical algorithm models on MFIF are shown in Fig. 91.
![Effects of different multi-focus image fusion networks[500–503" target="_self" style="display: inline;">–503].](/Images/icon/loading.gif)
Figure 91.Effects of different multi-focus image fusion networks[500–503" target="_self" style="display: inline;">–503].
It can be observed that the reconstruction results of some images exhibit regional blurring [Fig. 91(f)] and edge artifacts [Fig. 91(e)]. This is because the end-to-end direct reconstruction method is unable to fully preserve every true pixel value and color from the source image[504,505]. Therefore, most models tend to adopt a decision graph or combine decision graphs with end-to-end reconstruction for image fusion[506]. Additionally, some approaches have not fully accounted for the defocus diffusion effect. Therefore, improving the modeling of the defocus diffusion effect and enhancing the visual quality of the fused image, while ensuring the generation of a full-focus image with clear details, remain key areas for further exploration and research.
(2) Multi-exposure image fusion
Light intensity in natural scenes exhibits a large dynamic range[507]. However, due to the limitations of digital sensors, images captured by digital cameras often fail to record all the details of a scene due to overexposure or underexposure. To address this issue, MEF is typically used, where images taken under different exposure settings are fused together to create high-dynamic-range (HDR) images. This method allows for a broader exposure range and a richer representation of the scene’s details.
In 2017, Kalantari et al.[508] proposed the first supervised MEF framework based on CNNs. This method involved registering three images with different low exposures using the optical flow method and then obtaining fusion weights through a CNN network to generate the final fusion image. In 2018, Wang et al.[509] introduced a lightweight fusion framework called EFCNN. This framework obtained multiple sub-images from source images and convolved their neighborhood information to achieve better image fusion results. The network structure and fusion results of this method are shown in Fig. 92.
![EFCNN[509]. (a) Network structure. (b) Source images. (c) Fusion image.](/Images/icon/loading.gif)
Figure 92.EFCNN[509]. (a) Network structure. (b) Source images. (c) Fusion image.
In supervised MEF algorithms, it is typically challenging to directly obtain a large number of real HDR images to create training datasets. In 2020, Pan et al.[510] introduced the information content enhancement network (ICEN), which uses manually selected images with good exposure as supervisory information for network training. This method can quickly generate high-quality HDR images for specific scenes. However, it still faces limitations in effectively recovering all information from shadowed or high-exposure areas in different application scenarios. The use of manually selected exposure images for generating supervisory information introduces a significant amount of subjectivity, which inevitably limits the performance of such approaches[511]. As a result, unsupervised methods are often more suitable for MEF tasks.
Unsupervised methods typically optimize the network fusion effect by constructing a loss function with non-reference indicators (such as MEF-SSIM, etc.). In 2017, Prabhakar et al.[512] introduced DeepFuse, the first unsupervised MEF network. This method first converted images to the YCbCr color space, then used a CNN to extract features from the Y channel, while the Cb and Cr channels were simply weighted before being combined. Finally, the three channels were converted back into RGB fusion images. The network structure and fusion effect of DeepFuse are shown in Fig. 93.
![Unsupervised multi-exposure image fusion network DeepFuse[512]. (a) Network architecture. (b) Underexposed image. (c) Overexposed image. (d) Fusion result.](/Images/icon/loading.gif)
Figure 93.Unsupervised multi-exposure image fusion network DeepFuse[512]. (a) Network architecture. (b) Underexposed image. (c) Overexposed image. (d) Fusion result.
However, the loss function based on MEF-SSIM only focuses on the exposure level of the fused image and overlooks its color information, which can result in pale or distorted colors in the final fused image. In 2020, Xu et al.[513] introduced the MEF-GAN, which utilizes the dataset proposed by Cai et al.[514] to establish an adjunct relationship and incorporate a self-attention mechanism. This enables the probability distribution of the fused images to closely resemble that of high-quality reference label images. In 2021, Yang et al.[515] proposed GANFuse, which introduces the sum of correlation differences (SCD) loss to create an antagonistic game relationship between the source images and the fused image from an information-combination perspective. In static scenes, this method achieves a significant improvement in MEF, as demonstrated in its network framework and results shown in Fig. 94.
![GANFuse image fusion network[515]. (a) Network architecture. (b) Overexposure image. (c) Underexposer image. (d) Fusion result.](/Images/icon/loading.gif)
Figure 94.GANFuse image fusion network[515]. (a) Network architecture. (b) Overexposure image. (c) Underexposer image. (d) Fusion result.
To summarize, the unsupervised fusion method does not require real labels, and its performance largely depends on the ability of non-reference indicators and loss functions to effectively characterize the quality of the fusion results and align well with the fusion process. As a result, its fusion effect is generally quite good. However, MEF technology still faces several challenges due to limitations such as sensor noise, camera shake, object motion, and computational complexity[516]. Future research will need to focus on establishing robust non-reference indices, considering the correlation between MEF and subsequent tasks when constructing loss functions, effectively utilizing the valuable prior information in the source images, and adapting these methods to multi-scene and dynamic environments.
5.1.2 Multimodal image fusion
It is often challenging for a single type or setting of image sensor to fully extract target information from a scene independently[517]. However, with the rapid advancements in information technology, sensing, and measurement technologies, multimodal images—acquired by using multiple sensors to collect complementary information of the same scene—provide a more comprehensive representation of the imaging environment. Multimodal image fusion addresses the redundancy, mode registration, characterization, and fusion of source images containing different modal information, ultimately producing a fused image with enriched content. Common applications of multimodal image fusion include the fusion of infrared and visible images, medical image fusion, and multispectral image fusion, among others.
(1) Infrared and visible image fusion
Infrared imaging is primarily based on the theory of thermal radiation, offering significant advantages such as resilience against environmental factors like adverse weather, darkness, rain, fog, and obstacle occlusion. It enables the detection of hidden targets. However, infrared images often suffer from drawbacks like low resolution, poor contrast, and blurred background textures. On the other hand, visible light imaging, which aligns with human visual perception, provides high resolution and rich texture details, accurately reflecting scene information. However, it is susceptible to environmental influences, such as weather conditions and smoke. By fusing infrared and visible images, it is possible to combine the strengths of both modalities, producing a fusion image that showcases a bright target with a richly detailed background.
In 2020, Jian et al.[518] proposed the SEDRFuse network, which utilizes a feature extractor to separately extract intermediate and compensation features from the input source images during the fusion stage. These features are then combined by multiplying the attention map with the intermediate features. Finally, the network decodes and reconstructs the intermediate and compensation features to obtain the fused image. However, implicit feature extraction limits the ability to fully integrate essential aspects such as contrast and structural information from the source images into the final fused image. To address this issue, Xu et al.[519] proposed a fusion method based on multi-scale feature cascades and non-local attention in 2024. In the designed network, the encoder employs a multi-branch cascade structure, and these convolution branches with different kernel sizes provide the encoder with an adaptive receptive field to extract multi-scale features. In addition, the fusion layer incorporates a non-local attention module that is inspired by the self-attention mechanism. The network structure and fusion results are illustrated in Fig. 95.
![Image fusion network based on multi-scale feature cascades and non-local attention[519]. (a) Network architecture. (b) Infrared image. (c) Visible image. (d) Fusion result.](/Images/icon/loading.gif)
Figure 95.Image fusion network based on multi-scale feature cascades and non-local attention[519]. (a) Network architecture. (b) Infrared image. (c) Visible image. (d) Fusion result.
In 2023, Tang et al.[520] introduced a fusion method called “dark non-sensation,” named DIVFusion, which employs the Scene Illumination Depart Network (SIDNet) to remove light degradation from nighttime visible light images while preserving valuable features of the source images. Additionally, the Texture Contrast Enhancement Fusion Network (TCEFNet) is used to integrate complementary information, enhance the contrast, and improve texture details of the fused features. This method produces end-to-end fused images with true color and significant contrast.
In 2020, Li et al.[521] proposed an unsupervised fusion network based on the pre-trained deep network DenseNet, addressing the lack of real image labels in the image fusion process. The network adopts a dense connection structure combined with an attention mechanism, using cross-dimensional weighting and aggregation to efficiently extract features and perform image fusion. The network structure is shown in Fig. 96. In 2024, Zhou et al.[522] introduced an image fusion network that incorporates an adaptive visible light exposure adjustment module. This method uses Gaussian and Laplace pyramids to decompose, reconstruct, and guide the filtering of feature weight maps, ultimately generating the fused image.
![Unsupervised infrared and visible image fusion network based on DenseNet[521]. (a) Network structure. (b) Infrared image. (c) Visible image. (d) Fusion image.](/Images/icon/loading.gif)
Figure 96.Unsupervised infrared and visible image fusion network based on DenseNet[521]. (a) Network structure. (b) Infrared image. (c) Visible image. (d) Fusion image.
In order to overcome the complexity of manually designing fusion rules with the AE method and reduce the limitations in terms of calculation difficulty and cost, Ma et al.[523] proposed the FusionGAN model in 2019. This model frames the fusion process as an adversarial game that retains infrared thermal radiation information and visible detail texture information. It also introduces detail loss and target edge enhancement loss, ensuring the detection of the target while preserving clearer details of the target’s edge texture. However, a single adversarial model can lead to an unbalanced fusion effect. To address this issue, in 2020, Ma et al.[524] proposed the DDcGAN, which features a dual discriminator structure that can fuse source images with different resolutions, ensuring information balance across the different modes.
Existing GAN-based fusion methods often overlook the global information of the image, leading to inconsistencies in the overall infrared intensity of the fused image. In 2024, Wu et al.[525] proposed the GAN-GA network, where the detail branch and content branch extract local and global information, respectively. By assigning weights based on their importance for fusion, this method better preserves local textures and infrared intensity. However, fusion methods based on GAN still face challenges in achieving a balance between the two different losses. This imbalance leads to an unstable optimization process, making the training relatively difficult and often causing distortions in the fused image[524].
In summary, while neural networks demonstrate strong generalization capabilities, several challenges remain, including significant loss of visible image detail, high image noise, inadequate emphasis on infrared target information, low resolution of fused images, and poor real-time performance of the algorithms. Addressing these issues will require further targeted improvements in the future to enhance the effectiveness and efficiency of image fusion methods.
(2) Multimodal medical image fusion
Multimodal medical images offer a more comprehensive description of medical conditions, which is crucial for clinical applications such as disease prevention, medical diagnosis, and surgical navigation. These images typically include data from computed tomography (CT), magnetic resonance imaging (MRI), positron emission tomography (PET), and single-photon emission computed tomography (SPECT). CT images are known for their short scanning time and high resolution, but they have limited tissue characterization capabilities. MRI images provide excellent soft tissue definition and high spatial resolution, but lack dynamic information like body metabolism. PET images are highly sensitive but suffer from low resolution. SPECT images are used to characterize biochemical, physiological, functional, and metabolic activities in vivo. Examples of medical images from these different modalities are shown in Fig. 97[526].
![Medical images of different modes[526].](/Images/icon/loading.gif)
Figure 97.Medical images of different modes[526].
Medical images obtained from a single modality can only provide local pathological information, which is often insufficient for a comprehensive diagnosis of diseases. Multimodal medical image fusion, on the other hand, integrates complementary information from multiple image modalities, enhancing the overall imaging quality. This fusion helps doctors better diagnose lesions and make more informed decisions[527]. In 2017, Liu et al.[528] proposed a fusion method based on CNN, which considered the imaging patterns of different medical modalities and applied a multiscale approach through an image pyramid. This method made the fusion process more aligned with human visual perception and achieved notable improvements in visual quality and objective evaluation. Recognizing the limitations of single-network performance, Fu et al.[529] proposed the multiscale residual pyramid attention network (MSRPAN) in 2021. This approach extracts high-dimensional features from the original multimodal images and fuses them using a features-energy ratio strategy. These fused features are then processed by a reconstructor to produce the final fused images.
In 2024, Do et al.[530] introduced an enhanced version of VGG19, called TL_VGG19, based on transfer learning. This model extracts both the base layer and detail layer of images using low-pass filters. The base layer is then fused with adaptive rules created through the balance optimization algorithm (EOA), which helps maintain image quality while retaining crucial details. In the same year, Liang et al.[531] proposed a novel deep learning-based medical image fusion method using a deep convolutional neural network (DCNN). The method extracts principal and salient components from the source images through low-rank representation decomposition and then reconstructs them to obtain the fused image. The experimental results demonstrate that the proposed method outperforms several state-of-the-art medical image fusion approaches in terms of both objective indices and visual quality. The network structure and fusion results are illustrated in Fig. 98.
![Medical fusion method[531]. (a) Network architecture. (b) Fusion results for CT and MRI images. (c) Fusion results for MRI and PET images. (d) Fusion results for MRI and SPET images.](/Images/icon/loading.gif)
Figure 98.Medical fusion method[531]. (a) Network architecture. (b) Fusion results for CT and MRI images. (c) Fusion results for MRI and PET images. (d) Fusion results for MRI and SPET images.
Simple CNN networks are often insufficient for high-quality and detailed texture fusion in medical images. To address challenges like the loss of detail and weak edge strength in the fusion process, In 2024, Zhou et al.[532] proposed a novel image fusion framework, target information enhanced image fusion network (TIEF), using cross-modal learning and information enhancement techniques. The framework consists of a multi-sequence feature extraction block, a feature selection block, and a fusion block. TIEF can effectively achieve cross-domain information acquisition and fusion and has robustness and generalization ability. The network structure and fusion results are shown in Fig. 99.
![TIEF[532]. (a) Network architecture. (b) Fusion image.](/Images/icon/loading.gif)
Figure 99.TIEF[532]. (a) Network architecture. (b) Fusion image.
In summary, while deep-learning-based medical image fusion methods have achieved high-quality and robust multimodal fusion, challenges such as the lack of comprehensive datasets, poor network generalization, failure to effectively model prior information of imaging sensors, and high computational costs remain prevalent. Additionally, issues like difficulties in application deployment and the discrepancy between quantitative evaluation metrics and human perception must not be overlooked[533]. Addressing these challenges in the future will be crucial for enhancing the fusion quality of multimodal medical images, which holds significant potential for improving clinical diagnosis and disease treatment.
5.1.3 Remote sensing image fusion
In order to obtain high-quality remote sensing images that offer both high spatial resolution and spectral resolution, optical remote sensing satellites typically employ two types of sensors: multispectral (MS) and panchromatic (PAN) sensors[534]. Multispectral sensors require a large instantaneous FoV (IFoV) to meet the SNR requirements, but this compromises the spatial resolution of the images. On the other hand, panchromatic images have a higher spatial resolution but lower spectral resolution. The goal of remote sensing image fusion is to combine the low-resolution multispectral image (LR-MSI) with the high-resolution panchromatic image (HR-PAN) through a fusion algorithm, resulting in a high-resolution multispectral image (HR-MSI).
In 2016, Masi et al.[535] proposed the multispectral and panchromatic image fusion method PNN based on CNN, marking the first use of CNN for information extraction and fusion of multispectral and panchromatic images. The network model for this approach is shown in Fig. 100. However, the network’s structure was too simple, leading to limited nonlinear fitting capabilities and resulting in some spectral distortion. In 2021, Cai et al.[536] introduced the SRPPNN method, which combined the super-resolution module with a progressive learning approach. This enhanced network was able to capture spatial details at different scales and progressively inject these details into the up-sampled multispectral images, improving the quality of the fused image.
In 2020, Guo et al.[537] introduced a method called blur kernel learning (BKL) to eliminate the network’s dependence on reference images. The method estimates both spatial and spectral blur kernels separately and combines interpolation sampling to model spatial and spectral degradation, ensuring consistency with the degradation process. In 2023, Liu et al.[538] proposed a supervised-unsupervised combination fusion network designed to address the issue of insufficient utilization of spectral information due to the scale-invariant assumption.
![Basic CNN architecture for PNN sharpening[535].](/Images/icon/loading.gif)
Figure 100.Basic CNN architecture for PNN sharpening[535].
In 2021, Liu et al.[539] introduced PSGAN, a remote sensing image fusion framework based on GAN, which uses continuous adversarial learning to ensure that the fusion results progressively align with the reference image’s distribution. This approach effectively generates high-quality fusion results, preserving fine spatial details and high-fidelity spectral information. To address issues such as single-scale fusion, feature neglect, and inadequate suppression of redundant information, Wu et al.[540] modeled an unsupervised generative adversarial network (GAN) with recursive mixed feature fusion for pan-sharpening, termed RMFF-UPGAN. The network consists of a generator and two U-shaped discriminators, leveraging a recursive mixed-scale feature fusion network to integrate multi-scale features. Experimental results demonstrated its superior performance compared to popular methods in both visual assessment and objective metrics. The network structure and fusion results are shown in Fig. 101.
![RMFF-UPGAN[540]. (a) Network architecture. (b) EXP. (c) D_P. (d) Fusion result. (e) Ground truth.](/Images/icon/loading.gif)
Figure 101.RMFF-UPGAN[540]. (a) Network architecture. (b) EXP. (c) D_P. (d) Fusion result. (e) Ground truth.
In 2020, Ma et al.[541] introduced PAN-GAN, which effectively utilizes the detailed information contained in full-resolution panchromatic images that are often overlooked. PAN-GAN uses a single generator with both a spectral discriminator and a spatial discriminator, ensuring that the spectral information from multispectral images and spatial information from panchromatic images are preserved simultaneously. Building on this approach, in 2024, He et al.[542] proposed an interactive network that incorporates cross-domain correlation information, achieving deep fusion of spatial and spectral features for improved image fusion performance.
In summary, with the continuous development of DL technology, various improved algorithms based on CNN and GAN have significantly enhanced the integration of MS and PAN images. However, existing methods still face challenges such as weak network transferability, inadequate consideration of the synergy between model-driven and data-driven approaches, and high network complexity. Moving forward, it is crucial to fully leverage the feature information from both MS and PAN images, improve the utilization of spectral and spatial information in fused images, and optimize the fusion effect. This will have substantial implications for applications such as environmental monitoring, natural disaster prevention and control, military reconnaissance, and climate prediction.
5.2 Image denoising
Due to the inherent physical limitations of various recording devices, random noise often arises during image acquisition, compression, and transmission, which impedes image observation and information extraction, thereby deteriorating the visual quality of images. Image denoising is the process of recovering an estimate of the underlying clean image by removing noise artifacts. From a mathematical perspective, image denoising with multiple noise superpositions is an inverse problem, which typically has non-unique solutions[543]. With the availability of large datasets, rapid advancements in computing power, and the development of neural network optimization algorithms, the performance of image denoising technology has seen substantial improvements in recent years[544]. Image denoising methods based on DL can be broadly categorized into supervised and unsupervised methods, depending on the learning paradigm used.
5.2.1 Supervised image denoising methods
Supervised image denoising, according to the definition of supervised DL, involves using a paired dataset consisting of noisy images and their corresponding clean images for supervised learning during the training process of neural networks. In recent years, with the continuous introduction of various synthetic noise datasets, supervised deep-learning-based image denoising methods have become dominant in the field of image denoising.
In 2016, Mao et al.[545] first proposed RED-Net, an image denoising network with a coding-decoding structure based on a residual model, addressing the issue of large parameters and high training and computation costs associated with most image denoising networks. RED-Net introduced a skip-layer connection between the convolution and deconvolution layers, enabling rapid convergence of the network and solving the gradient disappearance problem. Similarly, in 2017, Wang et al.[546] proposed the dilated residual CNN for removing Gaussian noise from images. This network utilizes dilated convolutions to expand the receptive field and sets a specific dilation factor, which enhances both quantitative and visual denoising results.
Most image denoising methods learn specific models for each noise level and use multiple models to handle images with different noise levels. However, this lack of flexibility in dealing with spatially varying noise limits their applicability in real-world noise reduction scenarios. In 2019, Guo et al.[547] proposed a blind denoising network, CBDNet, trained on noisy-clean image pairs. The network employs an asymmetric loss function and achieves interactive denoising by adjusting the noise level map, effectively suppressing image noise. The framework of this denoising model is shown in Fig. 102.
![FFDNet network[547]. (a) Network structure. (b) Noisy image. (c) Denoised image.](/Images/icon/loading.gif)
Figure 102.FFDNet network[547]. (a) Network structure. (b) Noisy image. (c) Denoised image.
As networks deepen, the number of parameters and denoising time also increases, making it challenging to apply these models to real-world denoising scenarios. To address this issue, many researchers have worked on improving the denoising ability of the network by broadening its structure. In 2020, Tian et al.[548] proposed BRDNet, a batch-renormalization denoising network, which combines two networks to increase the width of BRDNet and capture more image denoising features. The model improves denoising performance by integrating dilated convolutions, batch-renormalization (BRN), and residual learning. In 2024, Rezvani et al.[549] proposed ERDF, a zero-shot learning-based fast residual denoising network, which first generates six distinct images through downsampling from noisy inputs. The network effectively utilizes a hybrid loss function, including residual, regularization, and guidance losses, to produce high-quality denoised images. This methodology demonstrates particular applicability in scenarios constrained by limited data availability and computational resources. Its network structure and fusion effect are shown in Fig. 103.
![ERDF network[549]. (a) Architecture of the proposed lightweight zero-shot network. (b) Qualitative comparison of denoising for different methods along with the corresponding PSNR.](/Images/icon/loading.gif)
Figure 103.ERDF network[549]. (a) Architecture of the proposed lightweight zero-shot network. (b) Qualitative comparison of denoising for different methods along with the corresponding PSNR.
The embedded attention mechanism in DL networks often allows the network to focus on the most important image features, improving the denoising effect. In 2024, Wu et al.[550] proposed the dual residual attention network (DRANet), which consists of two different subnetworks: the residual attention block (RAB) and the hybrid dilated residual attention block (HDRAB). These subnetworks work together to extract complementary features, enhancing the model’s learning ability. The network structure and fusion effect are shown in Fig. 104.
![DRANet network[550]. (a) Network structure. (b) Ground truth A. (c) Noisy image A. (d) Denoised image A. (e) Ground truth B. (f) Noisy image B. (g) Denoised image B.](/Images/icon/loading.gif)
Figure 104.DRANet network[550]. (a) Network structure. (b) Ground truth A. (c) Noisy image A. (d) Denoised image A. (e) Ground truth B. (f) Noisy image B. (g) Denoised image B.
Because real images containing noise are the only ones obtainable in real-world scenarios, constructing paired training datasets for image denoising is very challenging, which limits the development of denoising technologies. In 2018, Chen et al.[551] creatively proposed the GCBD network model. This model first used a GAN-based generation network to estimate the noise distribution of input images and generate noise samples to create paired training datasets. These datasets were then used to train a CNN for image denoising. In 2024, Wang et al.[552] introduced the RCA-GAN, which combines a residual structure with a collaborative attention mechanism to enhance the representation of edge and texture features, further improving the retention of details in complex images. The network structure is shown in Fig. 105.
![RCA-GAN[552]. (a) Network structure. (b) The ground truth. (c) Noisy images. (d) Denoised images.](/Images/icon/loading.gif)
Figure 105.RCA-GAN[552]. (a) Network structure. (b) The ground truth. (c) Noisy images. (d) Denoised images.
5.2.2 Unsupervised image denoising methods
Although a large number of algorithms have been proposed in the field of supervised image denoising and satisfactory denoising results have been achieved, there is still a challenge in obtaining real or synthetic image denoising datasets, especially in application scenarios such as hyperspectral remote sensing and medical imaging. These fields often lack accessible datasets for studying image denoising algorithms. As a result, unsupervised image denoising algorithms have become one of the main branches of research in the field of image denoising.
In 2018, Lempitsky et al.[553] proposed the deep image prior (DIP) method, an unsupervised technique that introduced a novel approach to regularizing imaging inverse problems. In 2019, Mataev et al.[554] developed a regularized denoising (RED) network, DeepRED, based on the DIP method. This approach combined DIP with RED to create an efficient unsupervised restoration process, enhancing the regularization effect by incorporating explicit priors, which improved image restoration outcomes. In 2023, Wang et al.[555] introduced the asymmetric Laplace noise modeling depth image prior (ALDIP) method, combined with the spatial–spectral total variation (ALDIP-SSTV) term. This method effectively leveraged the spatial–spectral correlation to achieve efficient denoising of hyperspectral images. The denoising framework and results are shown in Fig. 106.
![ALDIP-SSTV network[555]. (a) Network structure. (b) Noisy image A. (c) Denoised image A. (d) Noisy image B. (e) Denoised image B.](/Images/icon/loading.gif)
Figure 106.ALDIP-SSTV network[555]. (a) Network structure. (b) Noisy image A. (c) Denoised image A. (d) Noisy image B. (e) Denoised image B.
Although DIP methods eliminate the need for paired training data sets, their performance generally lags behind traditional BM3D image denoising algorithms. In digital images, the noise pixels at different locations are independent, given a specific pixel value[544]. In 2018, Lehtinen et al.[556] introduced the Noise2Noise method, which learns the mapping function between two independent noisy images. By sharing the features of their underlying clean images, it enables image denoising through clean image features and minimization of MSE loss. A significant drawback of the Noise2Noise method is that it requires pairs of noisy images. In 2019, Laine et al.[557] proposed a novel approach using a network with “blind spots” in the receptive field to eliminate the need for clean images. The method involves rotating the input images into four noise reduction network branches for training and learning, significantly improving training efficiency and image quality. The network framework for this method is shown in Fig. 107.
![Four-branch image noise reduction network[557]. (a) Network structure. (b) Noisy image A. (c) Denoised image A. (d) Noisy image B. (e) Denoised image B.](/Images/icon/loading.gif)
Figure 107.Four-branch image noise reduction network[557]. (a) Network structure. (b) Noisy image A. (c) Denoised image A. (d) Noisy image B. (e) Denoised image B.
In 2018, Soltanayev et al.[558] introduced SDA-SURE, a method that combines Stein’s unbiased risk estimator (SURE) and stacked denoising autoencoder (SDA). This deep-neural-network-based noise canceller, trained with the SURE criterion, achieves high noise-reduction performance, even when real data without noise is used for training. In 2019, Zhussip et al.[559] extended this approach by proposing eSURE, an enhancement to the original SURE method. By training deep Gaussian noise cancellers with eSURE, they demonstrated improved performance, achieving results comparable to Noise2Noise methods.
In summary, deep-learning-based image denoising methods exhibit strong learning and representation capabilities. By training on large datasets, these models can accurately capture image features. However, challenges remain in the field of image denoising, such as ensuring the universality and robustness of denoising algorithms across different noise sources and levels. Additionally, issues related to model representation and result interpretability, balancing network performance with computational efficiency, improving software and hardware compatibility, enhancing the integration of local and global information, and improving the memory capability from shallow to deep layers need to be addressed. Furthermore, increasing the generalization ability of the model to handle real-world noise and video noise remains an ongoing challenge. Future advancements in network improvement and optimization will be crucial for enhancing the performance of deep-learning-based image denoising methods.
5.3 Image enhancement
Under normal circumstances, digital images acquired by various imaging devices often exhibit issues such as blurring, unbalanced contrast, and uneven brightness distribution. These factors can negatively impact both the visual perception of the image and the subsequent processing tasks. Image enhancement refers to the process of adjusting properties such as brightness, contrast, and color balance to improve the visual quality of the image and enhance its interpretability from a human perception perspective.
Traditional image enhancement algorithms primarily rely on spatial-domain and frequency-domain processing. These methods typically suffer from high computational complexity and lack robustness and generalization. In recent years, DL has emerged as a powerful approach for image enhancement. By leveraging large datasets and complex neural network architectures, DL models can learn patterns and features in images to achieve outstanding enhancement results. Common application scenarios for image enhancement include low-light image enhancement, underwater image enhancement, and medical image enhancement.
5.3.1 Low-light image enhancement
For the low-light image enhancement task, inspired by Retinex theory, Zhang et al.[560] proposed the deep network KinD in 2019. This network decomposes the image into reflectance and illumination components, allowing for arbitrary adjustment of the image contrast to achieve better low-light enhancement results. In 2024, Xiang et al.[561] proposed a deep learning network based on wavelet transform to address issues commonly found in low-light images captured at night, such as improper exposure, color distortion, and noise, as shown in Fig. 108. The proposed network comprises a low-frequency restoration subnet and high-frequency reconstruction subnet that uses an optimal coefficient of wavelet decomposition to construct a frequency pyramid. The proposed approach quantitatively and qualitatively surpasses state-of-the-art approaches, particularly for real and complex low-light scenarios.
![Low-light image enhancement network[561]. (a) The network structure. (b) Input image and enhancement results.](/Images/icon/loading.gif)
Figure 108.Low-light image enhancement network[561]. (a) The network structure. (b) Input image and enhancement results.
Generally, low-light image enhancement methods based on CNN focus on decomposing the image into illumination and reflection components based on Retinex theory. However, these methods often fail to fully address noise control during the enhancement process and perform poorly under complex lighting conditions[562]. In 2021, Liu et al.[563] proposed the PD-GAN, which uses residual-dense blocks and a coding-decoding architecture to enhance extremely low-light images. This method achieves good denoising while preserving texture details. Recognizing that low-light images with strong noise may interfere with enhancement effectiveness. In 2024, Han et al.[564] proposed a low-light image enhancement method called UM-GAN, as shown in Fig. 109, based on a generative adversarial network (GAN). Initially, a generator network adopting an encoder-decoder structure is developed. Subsequently, a novel strategy is introduced to merge information by utilizing inverted greyscale images and low-light images as inputs. Experimental results show that using a GAN, UM-GAN can effectively restore image details and improve the overall image quality.
![Improved UM-GAN network[564]. (a) Network structure. (b) Low-light inputs and enhancement images.](/Images/icon/loading.gif)
Figure 109.Improved UM-GAN network[564]. (a) Network structure. (b) Low-light inputs and enhancement images.
Although the methods mentioned above have achieved good results in low-light image enhancement, they do not fully consider the key features of the image, leading to insufficient detail enhancement. In 2017, Gharbi et al.[565] proposed a depth bilateral image enhancement method based on multilayer feature fusion. This method uses three strategies to integrate features at different levels, enabling the model to learn full-resolution, non-scale-invariant effects that effectively enhance low-brightness images. Recognizing that existing methods fail to address problems like brightness, contrast, and noise simultaneously, Lv et al.[566] introduced a multi-branch low-light image enhancement network (MBLLEN) in 2018. The network consists of a feature extraction module (FEM), an enhancement module (EM), and a fusion module (FM), which extracts image features at various levels through multiple subnetworks. The network structure and the enhancement effect of the image after multi-branch fusion are shown in Fig. 110.
![MBLLEN network[566]. (a) Network structure. (b) Input image. (c) Enhancement result.](/Images/icon/loading.gif)
Figure 110.MBLLEN network[566]. (a) Network structure. (b) Input image. (c) Enhancement result.
5.3.2 Underwater image enhancement
In recent years, numerous excellent algorithms have been developed for typical underwater image enhancement tasks. In 2017, Perez et al.[567] proposed the first underwater image enhancement method based on CNN. This method used pairs of clear and fuzzy images to train an end-to-end enhancement network, successfully enhancing underwater images with strong generalization ability. In 2020, Cao et al.[568] introduced NUICNet to address the issue of uneven illumination in underwater images. The network directly separated the illumination layer and the ideal image from the original image, achieving impressive visual effects. The network structure and enhancement results are shown in Fig. 111. In 2023, Li et al.[8] proposed UAUIR-Net, an unsupervised underwater image enhancement method composed of four sub-modules. This method improves the accuracy of image recovery by utilizing advanced attention mechanisms and self-supervised learning, while also reducing noise and other adverse effects, enhancing the robustness and generalization of the algorithm.
![UWGAN[568]. (a) Network structure. (b) Input image A. (c) Enhancement image A. (d) Input image B. (e) Enhancement image B.](/Images/icon/loading.gif)
Figure 111.UWGAN[568]. (a) Network structure. (b) Input image A. (c) Enhancement image A. (d) Input image B. (e) Enhancement image B.
With the in-depth research of CNN in the field of underwater image applications, the challenge of limited datasets has gained increasing attention. In 2017, Li et al.[569] proposed the WaterGAN, which synthesized underwater images from natural images and depth images using a generator. The network used a cycle-based approach with real underwater images and generated images being passed through a discriminator to obtain pairs of underwater and real images, enabling the learning of underwater image restoration tasks through SegNet. However, due to the presence of color noise in the images generated by WaterGAN, Wang et al.[570] proposed UWGAN in 2019. This method uses color images and their depth maps as inputs, generates learning parameters for adversarial training, and synthesizes real underwater images. The network structure and image enhancement results are shown in Fig. 112. In 2023, Liu et al.[571] introduced WSDS-GAN, a two-stage double-supervised learning network. This method combines strong-weak supervised learning with cyclic consistency loss to improve the resolution and quality of underwater image reconstruction.
![UWGAN. (a) Network structure[570]. (b) Input image A. (c) Enhancement image A. (d) Input image B. (e) Enhancement image B.](/Images/icon/loading.gif)
Figure 112.UWGAN. (a) Network structure[570]. (b) Input image A. (c) Enhancement image A. (d) Input image B. (e) Enhancement image B.
To sum up, whether for low-light image enhancement or underwater image enhancement scenarios, obtaining real clear images for supervised learning is a significant challenge, which limits the practical enhancement results. The unsupervised method, which does not require real images as training labels, significantly improves the enhancement performance. Moving forward, combining design optimization loss functions, modifying the attention mechanism of the network, combining Retinex theory, and utilizing adaptive perceptual learning for multi-stage feature extraction can further enhance the quality and visual effect of the enhanced images.
5.4 Other image processing methods
Similarly, for other classic problems in image processing, such as image restoration and image compression, researchers have also proposed many efficient and robust solutions based on DL methods.
5.4.1 Image restoration
Image restoration is the process of recovering a clean underlying image from degraded observations. This task is an inverse problem where the goal is to reverse the degradation process. The challenge arises from the infinite possible mappings between the multi-dimensional degraded observations and the recovered images, making it a pathological problem. Since the inverse mapping is typically unknown, the solution space is vast, and regularization techniques are required to find feasible and optimal solutions. As a result, much of the research in image restoration focuses on developing effective analytical models and learning schemes to approximate the true mapping and restore degraded images. Common restoration operations include denoising, defogging, deblurring, and super-resolution.
The presence of haze, caused by turbidity media such as fog and dust, leads to poor image visibility and introduces complex nonlinear noise, making haze removal a highly challenging and pathological problem. In 2017, Li et al.[572] proposed AOD-Net, an all-in-one image defogging network that directly generates defogged images by employing a lightweight CNN. In 2018, Ren et al.[573] introduced a method that processes input images with white balance (WB), contrast enhancement (CE), and gamma correction (GC) before training a multiscale network to remove fog. In 2019, Li et al.[574] proposed a double-branch image defogging algorithm that incorporated both supervised and unsupervised learning, using a supervised loss function and the dark channel prior to constraining the network and achieving efficient end-to-end defogging. The network framework and resulting defogging effect are shown in Fig. 113. In 2020, Park et al.[575] combined CycleGAN and cGAN to form a heterogeneous GAN for image defogging.
![Semi-supervised image de-fogging network[574]. (a) Network architecture. (b) Image containing fog. (c) Fog removal image.](/Images/icon/loading.gif)
Figure 113.Semi-supervised image de-fogging network[574]. (a) Network architecture. (b) Image containing fog. (c) Fog removal image.
The method of improving image resolution through hardware enhancements often leads to unintended effects, such as a reduction in the SNR and increased manufacturing costs. To overcome the limitations of sensor and optical manufacturing technologies, the approach of obtaining high-resolution (HR) image restoration from multiple low-resolution (LR) images using signal processing techniques has become one of the most widely researched areas.
In 2015, Dong et al.[576] proposed the single-image super-resolution method (SRCNN), which added a nonlinear mapping layer to expand the network’s deeper structure. This extension enabled the SRCNN to process all three color channels simultaneously, improving both operation speed and restoration quality. In 2017, Ledig et al.[577] introduced the SRGAN, the first application of GAN in the field of super-resolution reconstruction. SRGAN combines adversarial loss and content loss functions into a perceptual loss function, achieving a super-resolution improvement for input images. However, treating low-frequency and high-frequency components in feature maps similarly led to issues like inadequate feature information utilization. In 2021, Chen et al.[578] proposed an image super-resolution method based on a feature attention mechanism, combining multiple information extraction modules with a feature map attention mechanism and transmitting them between feature channels. The channel characteristics were adaptively adjusted using interdependence, which helped restore more image details. In 2022, Tu et al.[579] proposed the remote sensing image super-resolution network (SWCGAN), which uses a residual-dense Swin Transformer module to extract depth features and up-sample them to generate HR images. A simplified Swin Transformer was also used as a discriminator for counter-training, further enhancing the super-resolution quality of remote sensing images. The network structure and super-resolution restoration results are shown in Fig. 114.
![SWCGAN. (a) Network structure[579]. (b) Low-resolution image. (c) Super-resolution recovered image.](/Images/icon/loading.gif)
Figure 114.SWCGAN. (a) Network structure[579]. (b) Low-resolution image. (c) Super-resolution recovered image.
Due to the pathological nature of image restoration tasks, traditional noise reduction methods relying on conventional mechanisms and models have inherent limitations. These methods often involve relatively simple degradation mechanisms and noise models. In contrast, DL approaches can better represent the complex nonlinear inverse problems involved in image restoration. This makes DL more suitable for tasks such as image deblurring, denoising, defogging, and super-resolution restoration. Moving forward, an important direction for improving network performance will be to enhance the applicability of DL networks across various scene image restoration tasks. This includes reducing the reliance on supervised image data and improving the overall quality of image restoration.
5.4.2 Image compression
Image compression aims to reduce the data size of an image while retaining as much relevant information as possible, enabling efficient storage and transmission. Traditional image compression methods, such as discrete cosine transform (DCT), discrete wavelet transform (DWT), and differential pulse code modulation (DPCM), exploit redundant information within the image to achieve compression. While these methods perform well in many cases, their generalization across different scenes and image types is often limited. In contrast, DL methods, known for their strong generalization and processing efficiency, are increasingly being applied to various image compression scenarios. Image compression is typically divided into two categories: lossy compression, where some information is discarded to reduce file size, and lossless compression.
Lossless compression ensures that no information is lost during compression, allowing the original data to be fully recovered without any distortion. In 2016, Shen et al.[580] proposed an image coding scheme based on stacked autoencoders, using two independent stacked autoencoders to automatically learn discriminative features from input images through entropy encoding, achieving lossless image compression. In 2019, Mentzer et al.[581] introduced L3C, a lossless image compression method that jointly models the image distribution and learned auxiliary representations, optimizing the network in an end-to-end manner for compression tasks. However, some methods fail to account for both high- and low-frequency components of images. To address this issue, Rhee et al.[582] proposed LC-FDNet in 2022, a lossless compression network utilizing frequency decomposition, which processes low- and high-frequency information separately through a coarse-to-fine encoding and decomposition process. This method adapts better to the color channels, spatial positions, and characteristics of images. For medical image compression, Min et al.[583] suggested a divide-and-conquer approach, dividing images based on anatomical features and generating the best predictor for each region. Adaptive switching is then performed based on the compressed region’s features.
Compared to lossless compression, lossy compression reduces the image size by permanently removing some information, particularly redundant data, which leads to a loss of information during compression. This method is easier to implement. In 2020, Li et al.[584] introduced three levels of residual learning to enhance image compression quality. By combining a ResNet structure with deep channel residual learning, full-resolution global residual learning, and an attention mechanism, they improved the compression results. The network framework and the compression outcomes of different regions of the self-adapting compressed image are shown in Fig. 115. In the same year, Cheng et al.[585] proposed a discretized Gaussian mixed likelihood method to parameterize the potential image distribution, creating a more accurate and flexible entropy model. They also used an attention module for parameter integration, further improving compression performance. In 2021, Chen et al.[586] proposed an end-to-end image compression method, NLAIC, which leverages global and local correlations to derive potential features and their super-priorities. They used an attention mechanism to generate implicit masks that adaptively weigh features, achieving high compression efficiency. In 2022, Tawfik et al.[587] introduced a lossy compression architecture based on a convolutional autoencoder (CAE), which preprocesses the image before compressing it through a code-decode structure, offering high image compression efficiency.
![Image compression network[584]. (a) Network framework. (b) Original image 1. (c) Compressed image 1. (d) Original image 2. (e) Compressed image 2.](/Images/icon/loading.gif)
Figure 115.Image compression network[584]. (a) Network framework. (b) Original image 1. (c) Compressed image 1. (d) Original image 2. (e) Compressed image 2.
In summary, DL methods are highly effective in identifying, extracting, and representing the essential feature information within images. They can also perform HR reconstruction of image data, effectively eliminating redundant information and enhancing both image resolution and visual quality. In the future, leveraging the strengths of DL to significantly improve image compression efficiency and further advance HR reconstruction will remain a key focus of research.
6 Conclusion
Driven by the demand for enhanced information transmission, computational imaging technology integrates a comprehensive design framework that encompasses “computational light source,” “computational medium,” “computational optical system,” “computational detector,” and “computational processing.” This holistic approach breaks away from the traditional optical imaging paradigm of “what you see is what you get,” overcoming limitations related to information dimensions, scale, resolution, field of view, and application scenarios. By achieving disruptive imaging capabilities characterized as “higher, farther, wider, smaller, and stronger,” computational imaging has significantly expanded the scope of optical imaging applications. However, traditional computational imaging methods are heavily reliant on manually designed priors, which introduce challenges such as complex algorithm design, high computational demands, low efficiency, and limited generalizability.
Deep learning methods enable the extraction of complex, abstract characteristics and patterns of light-field information through a data-driven approach, eliminating the need for explicit physical models or prior knowledge. By leveraging their powerful nonlinear fitting capabilities, these methods construct network models to reconstruct light-field information. Compared to traditional approaches, deep learning demonstrates superior robustness, reconstruction quality, and computational efficiency in addressing various nonlinear computational imaging problems, such as imaging through scattering media, compressed sensing imaging, minimalist optical system design, and super-resolution imaging. However, data-driven computational imaging methods also face practical challenges, including limited availability of datasets, poor model generalization, high requirements of computing resources, and deficiencies in model interpretability and controllability.
The 2024 Nobel Prize in physics was awarded to American scientist John J. Hupfield and British–Canadian scientist Geoffrey E. Hutton for their groundbreaking “fundamental discoveries and inventions in machine learning through artificial neural networks.” This achievement underscores the critical role of interdisciplinary research in driving scientific innovation and highlights the transformative impact of deep learning across diverse fields. The deep integration of deep learning and computational imaging is evolving from “algorithm-assisted imaging” to “redefining imaging systems.” Looking ahead, the deeper integration of computational imaging with deep learning promises to address existing problems in practical applications, Achieving efficient collaborative optimization between physical models and neural networks. This synergy is expected to enable superior imaging capabilities, improve adaptability across various scenarios, and support a wide range of applications, including space remote sensing, biomedical imaging, underwater imaging, and military imaging systems.