The rapid development of artificial general intelligence (AGI) introduces significant performance challenges for next-generation computing. Electronic devices such as graphics processing units (GPUs) are constrained by computational and energy efficiency limitations, hindering the advancement of modern AGI models.1 In contrast, photonic computing offers unprecedented low-power computing at the speed of light, promising superior performance for intelligent tasks.2,3 Spatial photonic computing, exemplified by diffractive deep neural networks (),4,5 achieves large-capacity computing but faces scalability issues due to the use of passive photonic devices. Meanwhile, integrated photonic computing, leveraging highly scalable Mach-Zehnder interferometers (MZIs),6 typically involves hundreds to thousands of parameters, posing challenges for large-capacity computing. Additionally, inherent analog noise and time-varying errors in these systems limit them to simple tasks and shallow models, which are inadequate for real-world AGI applications.
In their recently published paper, Xu et al. introduce Taichi, as illustrated in Fig. 1(a), a large-scale, highly scalable distributed photonic computing architecture designed for real-world AGI tasks, leveraging the advantages of optical diffraction and interference.7 The double diffractive units for large-scale input and output passively perceive high-dimensional data and compactly represent them through universal diffraction, as illustrated in Fig. 1(b). Task-specific feature embeddings are efficiently achieved via tunable matrix multiplication with fully reconfigurable MZI arrays. These components together form the scalable “DE-IE-DD” framework of Taichi, which can significantly reduce the required scale of the reconfigurable MZI array and support diverse and complex tasks with 3.8% reconfigurable part of 4256 total neurons. And the distributed architecture divides large tasks into several subtasks, which are parallel processed using Taichi chiplets, as depicted in Fig. 1(c). Computing resources are allocated to multiple independent clusters, each organized separately for subtasks and ultimately synthesized to handle complex advanced tasks. The authors report an experimental 1000-category classification of 91.89% on the 1623-category Omniglot dataset, marking the first attempt at achieving on-chip capability with 13.96 million neurons and an energy efficiency of 160 TOPS/W. This represents a highly promising approach in the field.
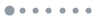
Figure 1.The large-scale distributed photonic computing with Taichi. (a) The schematics for Taichi. (b) The execution units of Taichi. (c) Large tasks are divided into several subtasks and are parallel handled using a series of the execution units of Taichi.
The authors further showcase on-chip photonic computing for content generation. By treating each note generation as a classification problem and employing the Bach-index as an evaluation metric, Taichi successfully implements a music generation network. Random noise served as the initial input with a Bach-index of 6.61%. With each iteration, the Bach-index increased, reaching 95.17% after 500 iterations, indicating a strong Bach style in the generated music. Additionally, the Taichi chip supports two-dimensional input and output signals. Its capabilities are further verified by functions such as building an image generation network to produce stylized images that imitate an artist’s style, converting font styles, and extracting advanced semantic information from two-dimensional images, showcasing the chip’s advanced content generation potential.
Sign up for Advanced Photonics TOC Get the latest issue of Advanced Photonics delivered right to you!Sign up now
Simply scaling up existing photonic computing architectures for large-scale, high-energy efficiency computing has proven impractical due to the exponential increase in analog noise that adversely affects performance. Early research proposed an all-analog chip that combined electronic and photonic computing, utilizing joint optimizations based on adaptive training to achieve superior system robustness.8 In contrast, Taichi’s distributed architecture leverages its shallow depth and broad width to achieve accuracy on the CIFAR-10 dataset comparable to a 16-layer VGG-16 network with only four distributed layers. The next logical step is wafer-level integration for real-life applications. Utilizing CMOS-compatible silicon photonic process platforms, photo-electric co-design, and advanced 3D packaging solutions could enable the design of a commercial photonic arithmetic computing engine (PACE).9 This development marks an exciting prospect for photonic computing and positions the rapidly advancing field as one to watch closely.
Hang Chen is a postdoctoral researcher and research associate at Tsinghua University. He received his BS degree, MS degree, and PhD degree in the School of Instrumentation Science and Engineering from Harbin Institute of Technology in 2015, 2017, and 2022, respectively. His current research interests include photonic neural networks and integrated photonic chips. He has published more than 10 peer-reviewed journal papers, including first authored papers on Advanced Photonics, Light: Science & Applications, and Engineering. He is a young editorial board member of Acta Optica Sinica and Chinese Laser Press.
Yichen Shen is the founder and CEO of Lightelligence, a company using integrated photonics technology to accelerate machine learning computation. Yichen received his PhD degree in physics from MIT in 2016, while his research is mainly focused on nanophotonics and artificial intelligence. During his PhD, Yichen has filed 8 US patents and published more than 25 peer-reviewed journal papers, including first authored papers in Science, Nature Photonics, and ICML. In 2017, Yichen Shen was recognized in Forbes 30 Under 30 (global) and Technology Review 35 Innovators Under 35 (TR35) China.