Introduction
Vision is the dominant sense in humans and most artificial intelligence systems. Accurate sensing of environmental illumination and efficient signal processing are fundamental for understanding visual information. To adapt and survive in changing natural environments, humans have developed an efficient vision system, equipped with diverse retinal neurons that extract different types of visual information. For instance, the strategy of temporal integration reduces random noise and highlights static objects, while the sensing of dynamically changing light information emphasizes moving objects. With diverse signal processing occurring in different types of neurons, the human eye boasts exceptional capabilities. Furthermore, the vertical alignment of the densely packed neuron array ensures our high-resolution vision.
Conventional CMOS image sensors are well developed and frame-based image sensors. The devices can achieve high fidelity reconstruction of visual information, giving rise to the wide application in various applications. However, the separation design of sensing, memory and processing units, and the redundant signal communication significantly reduce the efficiency in features such as speed, space and energy consumption. Neuromorphic hardware with in-device integration of sensing, memory and processing functions can dramatically increase the efficiency in specialized applications[1−10], however, a specialized processing can hardly fit in diversified applications. Therefore, combining the high compatibility of a frame-based image sensor and the high efficiency of a neuromorphic image sensor, a device can have strong capabilities and find use in various applications including machine vision and robotics.
In this study, we developed a nanowire array based dual-mode image sensor, which can be switched between frame-based standard imaging mode and neuromorphic imaging mode. The device is structured on a Pb-CsPbI3-SnO2-ITO foundation. Under a small external bias of 0.01 V, photo-generated electrons and holes can be efficiently separated and transferred to the external circuit, resulting in a standard photo response for frame-based imaging. In this mode, the device directly reconstructs visual information that is compatible with most artificial vision applications. Under a higher bias of 0.5 V, the holes can be blocked and accumulate in CsPbI3, leading to a synaptic photo response. In this neuromorphic imaging mode, the device integrates sensing, memory, and processing functions, exhibiting high efficiency. For instance, the device's photocurrent demonstrates the temporal integration of the visual signal, filtering random noise to enhance contrast. We demonstrated image reconstruction in both modes with a 10 × 10 crossbar array. Moreover, the biomimetic dense (>107 mm-2) vertical alignment of the nanowire array ensures high-resolution potential. Given the pitch between nanowires is ~200 nm, the device has potential for diffraction limit imaging. Furthermore, as the all-inorganic perovskite nanowire array is protected by the porous aluminum membrane (PAM), the device stability can exceed one year.
In summary, we developed a dual-mode image sensor with electrically switchable standard and neuromorphic imaging functions, making it compatible with various applications. The detailed design and functions are discussed in the following parts.
The biomimetic nanowire array
The biomimetic design of the nanowire array is depicted in Fig. 1. Fig. 1(a) presents schematics of a human eyeball and the retinal neuron array. There are primarily three layers of neurons in the human eye. The photoreceptor cells, which include rod cells and cone cells, are responsible for detecting illumination. Optical stimuli are detected by these photoreceptor cells and transformed into neuronal impulses. Specifically, rod cells, which are sensitive to dim light, play a key role in detecting shape-related information. On the other hand, cone cells are responsible for color perception, as they contain three types of pigments sensitive to red, green, and blue light. The interplay of these color-sensitive cone cells facilitates our ability to perceive the vibrant world around us. These signals are then processed and transferred to bipolar cells through synapses. Bipolar cells accentuate the ascending and descending phases of illumination, thereby enabling the extraction of information related to edges and contrasts. Temporal integration in some bipolar cells can effectively reduce noise and smooth out fluctuations. Subsequently, the signal is relayed to ganglion cells for further processing and encoding into retinal spikes, which are sent to the brain. Different ganglion cell types each process distinct visual features. For instance, certain ganglion cells respond to brightness fluctuations, others discern color variations, while some are tuned to detect motion. There are different types of bipolar and ganglion cells responsible for diversified processing, leading to our efficient visual recognition that supports our survival in changing natural environments.
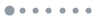
Figure 1.(Color online) (a) Schematic of a human eyeball and the retinal neuron array. (b) Schematic of an electric eye and the biomimetic nanowire array. (c) SEM image of the nanowire array, the scare bar refers to 1 μm. (d) The XRD pattern of CsPbI3 nanowires with Pb nano clusters. (e) The XRD pattern of ITO electrodes. (f) The XRD pattern of SnO2 nanotubes.
Inspired by the structure of the retinal neuron array, we developed a perovskite nanowire array with dense vertical alignment, as shown in Fig. 1(b). Biomimetic eye devices that mimic biological eyes both structurally and functionally have shown great potential in artificial vision applications[11−13]. The key component of a biomimetic eye is the artificial retina, which is inspired by the effective structure and powerful functions of the biological retina. In this work, the artificial retina is based on a PAM-assisted perovskite nanowire array. Fig. 1(c) presents the scanning electron microscope (SEM) image of this nanowire array, while Fig. 1(d) displays the X-ray Diffraction (XRD) pattern of the CsPbI3 nanowires. As the Pb clusters are not fully consumed in the CVD process, Pb peaks are evident in the XRD curve. XRD curves of ITO and SnO2 are shown in Figs. 1(e) and 1(f), respectively. The pitch between the optoelectronic nanowires is approximately 200 nm, resulting in an ultra-high density of over 107 mm-2. This high density affords the potential for diffraction-limited resolution imaging. Furthermore, the nanowire array and the reading circuit are vertically aligned. Compared to traditional horizontal alignment, our system can achieve higher integration density and a relatively larger effective area.
All inorganic perovskite CsPbI3 has garnered extensive research interest due to its exceptional optoelectronic properties, appropriate energy band gap, and superior chemical stability. Its promising capabilities in light absorption, photodetection, and power conversion highlight its immense potential across a broad range of applications[14−17]. With an energy band of approximately 1.8 eV, it can respond to most visible light. Furthermore, its robust temperature and chemical stability prevent decomposition during subsequent ALD processes. The nanowire array is synthesized using a nano template-assisted growth method, with the fabrication process illustrated in Fig. 2. Detailed methods are shown in supplementary information. The advent of novel materials and fabrication techniques has paved the way for the creation of a myriad of nanostructures[18−22]. Among these, the PAM exhibits significant potential in the field of optoelectronics. The PAM, with well-aligned nano channels, is prepared via an anodization process[23, 24]. These channels are filled with Pb nano clusters through an electrochemical deposition process. The all-inorganic perovskite nanowire array is then grown in the freestanding PAM using a chemical vapor deposition (CVD) method. Since the nanowire array is synthesized by a vapor growth method, it exhibits good uniformity. It's worth noting that the PAM effectively shields the nanowire array from air and humidity, thereby ensuring its good stability[25]. On the perovskite nanowire array, we deposit SnO2 nanotubes on the shell of the PAM channels using the atomic layer deposition (ALD) method. Finally, crossbar indium tin oxide (ITO) electrodes are sputtered onto both sides of the artificial retina. This well-designed structure supports the unique bias-dependent photo response, which will be discussed in the following section.
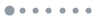
Figure 2.(Color online) The fabrication process of the nanowire array.
Bias dependent photo response
The bias-dependent photo response is depicted in Fig. 3. Under an extremely low bias of 0.01 V, the device exhibits standard photo response behavior, as shown in Fig. 3(a). When the device is exposed to illumination, it rapidly generates a positive photocurrent. Conversely, when the illumination is switched off, the photocurrent abruptly decreases. In the standard photo response mode, the device directly converts optical signals to electrical signals without additional processing. The electrical signal can then be sent to various processors to extract information, including edge detection, motion tracking, and pattern recognition. Working in tandem with well-developed post-processing software, the frame-based image sensor is versatile and compatible with a range of applications.
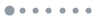
Figure 3.(a) Photo current at 0.01 V under 872 μW/cm2 illumination. (b) Photo current at 0.5 V under 143, 325, 573, 742, 872 μW/cm2 illuminations, respectively. (c) Photo current at 0.5 V under 872 μW/cm2 illuminations with different pulse widths. (d) Photo current at 0.5 V under 10 pulses of 872 μW/cm2 illumination. (e) Photo current at 0.5 V under 100 pulses of 872 μW/cm2 illumination. (f) Normalized Photo current at 0.5 V under 872 μW/cm2 illumination with 1 s pulse width after certain months. The device was kept in indoor environment with a temperature range of 5−35 °C and a humidity range of 30%−80%.
Under a higher bias of 0.5 V, the device operates in a neuromorphic mode. The device exhibits a synaptic photo response that integrates sensing, memory, and preprocessing functions. The optical stimulus-dependent plasticity is shown in Fig. 3(b). Five optical stimuli with the same pulse width but different light intensities are directed at the device. During each stimulus, the photocurrent steadily increases and gradually decreases after the stimulus. A stronger optical stimulus can generate a higher photocurrent after a certain time, with the signal being retained for a while.
The synaptic duration-dependent plasticity is shown in Fig. 3(c). The device detects five optical stimuli with the same light intensity but different pulse widths. The photocurrent generated under a longer pulse width stimulus is higher, and the signal is retained for a longer time. Both optical stimulus-dependent plasticity and synaptic duration-dependent plasticity mimic the formation and deformation processes of biological synapses, where a stronger or longer stimulus can lead to a stronger and longer-lasting synaptic connection. Additionally, synaptic number-dependent plasticity is demonstrated in Figs. 3(d) and 3(e), where 10 and 100 optical stimuli are respectively directed at the device. Under more cycles of optical stimuli, the photocurrent reaches a higher level and is retained for a longer time. This is similar to biological synapses, where long-term plasticity can be achieved through multiple optical stimuli. The optical synaptic behavior under 0.5 V enables the device to learn and preprocess the input signal, optimizing the efficiency of subsequent pattern recognition and other applications. Besides, the device exhibits good stability. Fig. 3(f) shows the normalized photocurrent under the same optical stimulus at specific months after the device's fabrication. The device retains more than 90% of its performance after 14 months, making it reliable for commercial applications.
To further illustrate the synaptic photo response in neuromorphic mode, we examined spike frequency-dependent plasticity, paired-pulse facilitation (PPF), and color-dependent PPF. The results are presented in Figs. 4−6. Fig. 4 illustrates spike frequency-dependent plasticity. When subjected to optical stimuli of the same pulse width, the device produces a higher photocurrent under illumination of higher frequency. Fig. 5 displays PPF under optical stimuli of varying frequencies, with the photocurrent from the second stimulus significantly amplified. Fig. 6 depicts PPF under different color illuminations of the same light intensity. The photocurrent is higher under shorter wavelengths due to the higher photon energy, which reduces recombination and bolsters carrier separation. In sum, the device reliably exhibits synaptic photo responses in neuromorphic mode, making it a strong contender for various applications.
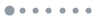
Figure 4.(a)−(c) Spike frequency-dependent plasticity of the device under 872 μW/cm2 optical pulses with the same pulse widths and different pulse intervals at 0.5 V.
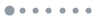
Figure 5.Paired pulse facilitation of the device under 872 μW/cm2 optical pulses with (a) shorter and (b) longer pulse widths and pulse intervals at 0.5 V.
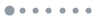
Figure 6.(Color online) Paired pulse facilitation of the device at 0.5 V under 712 μW/cm2 optical pulses with wavelengths of (a) 650 nm, (b) 520 nm and (c) 405 nm, respectively.
The unique bias-dependent photo response originates from the energy band diagram. In Fig. 7 we discussed the working mechanism in the standard mode and the neuromorphic mode. Figs. 7(a)−7(c) illustrate the working mechanism of the standard imaging mode under a 0.01 V bias. The Fermi level of CsPbI3 is slightly lower than the work function of the Pb bottom electrode, forming a Schottky junction at the interface (Fig. 7(a)). Under illumination, photo-generated electrons are transported to SnO2, while holes are blocked by the Schottky junction between Pb and CsPbI3 (Fig. 7(b)). As a result, the CsPbI3 becomes positively charged, suppressing electron transport. Due to more efficient carrier transport, the photocurrent initially reaches its peak, then decreases to a steady state as the device achieves a new equilibrium. This results in a photocurrent spike, as seen in Fig. 7(a). Because a small bias can hardly block holes or cause hole accumulation in the perovskite nanowire, the device exhibits a steady photo response. Once the illumination is turned off, the device ceases to generate photocurrent.
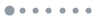
Figure 7.(Color online) (a)−(c) Working mechanism of standard mode. (a) Energy band diagram at 0.01 V bias. (b) Energy band diagram at 0.01 V bias under short time illumination. (c) Energy band diagram at 0.01 V bias under long time illumination. (d)−(f) Working mechanism of standard mode. (d) Energy band diagram at 0.5 V bias. (e) Energy band diagram at 0.5 V bias under short time illumination. (f) Energy band diagram at 0.5 V bias under long time illumination.
The mechanism of the neuromorphic imaging mode is shown in Figs. 7(d)−7(f). When a higher bias of 0.5 V is applied, the hole-blocking effect of the Schottky junction is enhanced (Fig. 7(d)). Under illumination, holes accumulate in the CsPbI3 (Fig. 7(e)). As the hole-blocking effect is enhanced by the external bias, the holes continue to accumulate in the nanowire, gradually increasing its conductivity (Fig. 7(f)). Thus, the photocurrent continuously increases under illumination, leading to synaptic plasticity. The bias-dependent photo response behavior supports the dual-mode imaging function, which will be further discussed and demonstrated in the next section.
Dual-mode imaging for various artificial vision applications
The typical process flows of visual recognition based on the two modes are depicted in Fig. 8. Similar to a conventional image sensor, the device can reconstruct optical stimuli into frames in the standard imaging mode. The device captures images from the environment, converts them into frame-based electrical signals, and stores them in a memory unit. The signals are then extracted and preprocessed by a preprocessor using strategies such as temporal integration and frame differencing. These preprocessed signals are stored in memory for extraction by the processor for further processing, such as pattern recognition or motion detection. Because frame-based signals can be sent to different types of preprocessors and processors, the standard imaging mode is compatible with most artificial vision applications. For example, dynamic optical information sensing plays a crucial role in motion detection, where moving objects are identified from a scene using established algorithms like frame differencing or background subtraction. However, these methods are incompatible with images preprocessed by the device in neuromorphic mode, as the signals from the static background are significantly enhanced in this mode.
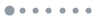
Figure 8.(Color online) Imaging process flows of standard and neuromorphic modes.
Under the neuromorphic imaging mode, the signal is preprocessed within the device using synaptic plasticity, allowing it to be directly sent to the processor. In this mode, the device achieves high efficiency by integrating the functions of sensing, memory, and preprocessing. Furthermore, the simplified structure, devoid of redundant units and signal communication, can significantly reduce both space occupation and energy consumption. While the preprocessed signal might not be suitable for all applications, it can dramatically increase the efficiency of certain application. Specifically, in-device signal integration can significantly reduce noise and enhance contrast, thereby greatly improving the efficiency of static pattern recognition.
To showcase the powerful capabilities of our device, we fabricate a 10 × 10 crossbar array to demonstrate basic image reconstruction under both modes. The schematic device structure is shown in Fig. 9(a). Given the well-aligned high-density nanowire array and the PAM's effectiveness in blocking crosstalk between nanowires, we only need to pattern the ITO electrode to create a pixel array. Fig. 9(b) schematically shows the electrical connection of the device. Each layer of the crossbar electrode is connected to a multiplexer, and the signals are read out by a source meter through pixel-by-pixel scanning. The crossbar ITO electrodes are fabricated via sputtering method with shadow masks. The width and thickness of the ITO electrodes are 0.5 mm and 150 nm, respectively. The pitch between the electrodes is 1 mm.
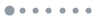
Figure 9.(Color online) (a) Schematic of the crossbar image sensor. (b) Schematic of the crossbar image sensor and its read-out circuit. (c) Input optical pattern for the imaging demonstrations. (d) Image acquired by the device under standard mode. (e) Images acquired by the device under neuromorphic mode after 1, 2, and 3 s learning process under exposure, and 1, 2, and 3 s forgetting process in dark environment.
Fig. 9(c) presents the input optical pattern, which includes two rectangles with gradient brightness. The pattern reconstruction under the standard mode is depicted in Fig. 9(d), where the device accurately reconstructs the input pattern. Fig. 9(e) illustrates imaging under the neuromorphic mode. During the learning process, when the device is exposed to the optical pattern, holes accumulate in the nanowire, gradually increasing its conductivity, and thereby developing a learning function for the input pattern. In this scenario, the photocurrent represents the temporal integration of optical stimuli. During the forgetting process, which begins after the light is turned off, the photocurrent gradually decreases, embodying a forgetting function of the previously learned pattern. Similar to a biological retina, the device can be trained by the optical pattern. In an imaging application, noise randomly occurs while the input pattern is static. Therefore, the learning and forgetting function can help to enhance the contrast and filter the noise. After this in-device preprocessing process, the accuracy of subsequent pattern recognition can be significantly improved[13].
High resolution imaging is important for imaging devices[26]. In our system, the PAM can isolate perovskite nanowires to avoid crosstalk. Thus, electrode patterning methods can be used to achieve high resolution. Potentially, there are two high-resolution structures: crossbar electrodes, and the combination of a global electrode with an active matrix. For crossbar structures, high-resolution electrodes can be fabricated via high-resolution inject printing[27], laser patterning[28], lithography[29], etc. For the integration of active matrices, the device has the potential to be integrated with a high resolution thin film transistor (TFT)[30] or a CMOS array using proper bonding methods.
By combining the standard imaging mode, which offers excellent compatibility, with the neuromorphic imaging mode, which provides high efficiency, the dual-mode image sensor has great potential in building powerful artificial vision systems. These systems can support the accelerating development of artificial intelligence applications, including computer vision, autonomous driving, and robotics.
Summary
Inspired by the structure and function of the human retina, we fabricated a dual-mode image sensor that can be electrically switched between standard and neuromorphic imaging modes. The biomimetic device is based on a vertically aligned, dense nanowire array that mimics the dense array of retinal neurons. The high nanowire density of >107 mm-2 suggests its potential in diffraction-limited resolution imaging. The device integrates the excellent compatibility of the standard imaging mode with the high efficiency of the neuromorphic imaging mode. This work presents a novel biomimetic device with powerful capabilities that could find use in various artificial vision applications.